Multi-output Deep-Supervised Classifier Chains for Plant Pathology.
IJCNN(2023)
摘要
Plant leaf disease classification is an important task in smart agriculture which plays a critical role in sustainable production. Modern machine learning approaches have shown unprecedented potential in this classification task which offers an array of benefits including time saving and cost reduction. However, most recent approaches directly employ convolutional neural networks where the effect of the relationship between plant species and disease types on prediction performance is not properly studied. In this study, we proposed a new model named Multi-output Deep Supervised Classifier Chains (MoDsCC) which weaves the prediction of plant species and disease by chaining the output layers for the two labels. Mo-DsCC consists of three components: A modified VGG-16 network as the backbone, deep supervision training, and a stack of classification chains. To evaluate the advantages of our model, we perform intensive experiments on two benchmark datasets Plant Village and PlantDoc. Comparison to recent approaches, including multi-model, multi-label (Power-set), multi-output and multi-task, demonstrates that Mo-DsCC achieves better accuracy and F1-score. The empirical study in this paper shows that the application of Mo-DsCC could be a useful puzzle for smart agriculture to benefit farms and bring new ideas to industry and academia.
更多查看译文
关键词
Deep Learning,Convolutional Neural Networks,Multi-Prediction,Plant Identification,Leaf Disease Classification,Plant Pathology
AI 理解论文
溯源树
样例
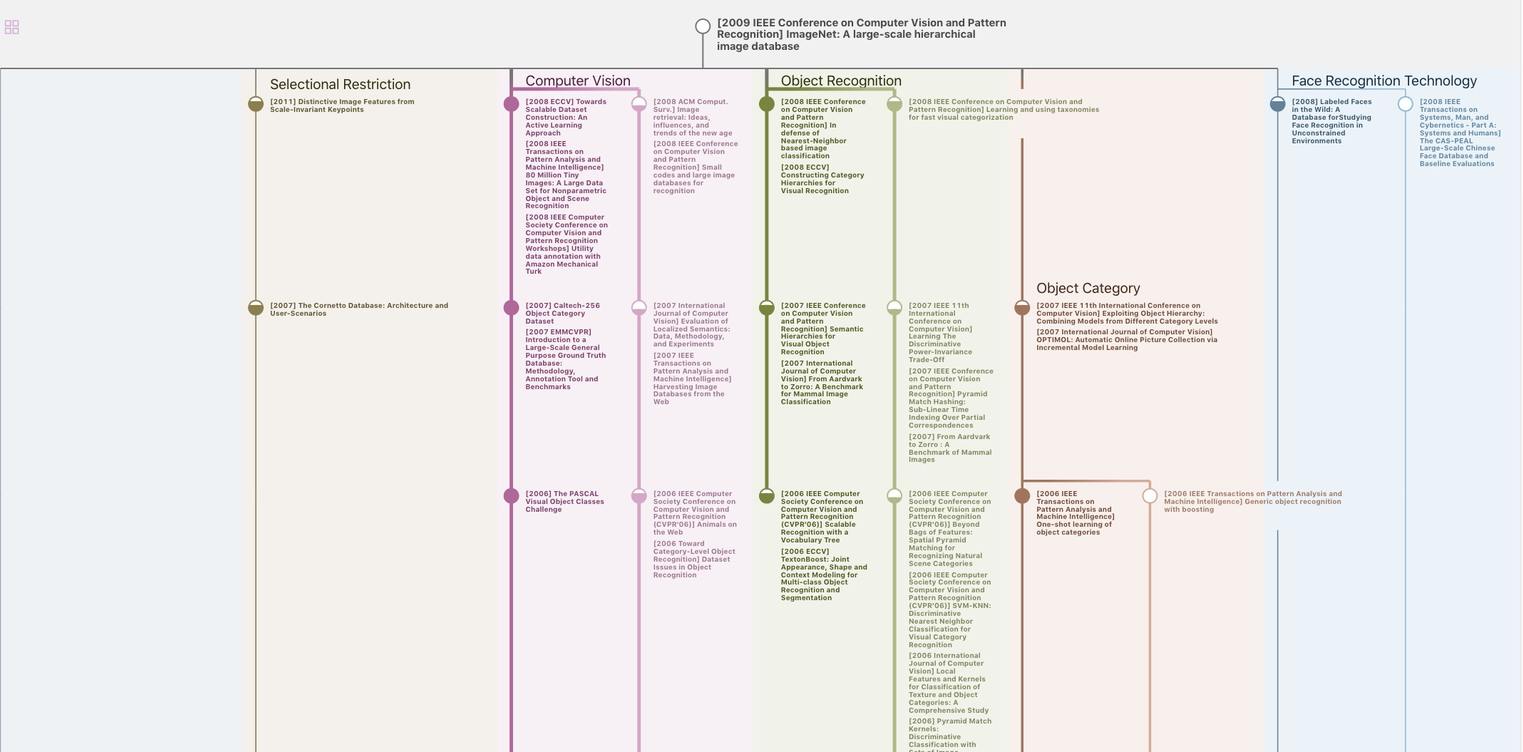
生成溯源树,研究论文发展脉络
Chat Paper
正在生成论文摘要