A biologically-inspired locally-connected spiking network for efficient and robust ground reaction force estimation in a legged robot.
IJCNN(2023)
摘要
The paper introduces a new structure of Liquid State Machine (LSM) characterised by local connectivity within the excitatory neurons of the reservoir layer. The architecture learning and testing capabilities are compared with the classical LSM network on an important robotic task of estimating exteroceptive information from proprioceptive signals coming from a simulated quadruped robot. The main advantages of the proposed architecture are discussed. The actual arrangement of the network resembles specific learning structures within the insect brain, endowed with interesting reaction-diffusion dynamics and classification capabilities. Simulation results are reported and compared with those ones obtained using a classic LSM. The proposed architecture, resembling typical biological solutions present in simple brains, through reaction-diffusion local mechanisms, significantly reduces the computational requirements needed by standard massively-connected neural network solutions. Moreover, the robustness of the introduced network, against faults in the sensory system, is demonstrated. In these conditions, the network is able to partially reconstruct the lacking sensory input signal from the learned relation with the other sensory input streams.
更多查看译文
关键词
architecture learning,biologically-inspired locally-connected spiking network,classical LSM network,classification capabilities,efficient ground reaction force estimation,excitatory neurons,exteroceptive information,insect brain,legged robot,liquid state machine,massively-connected neural network solutions,proprioceptive signals,reaction-diffusion local mechanisms,reservoir layer,robust ground reaction force estimation,sensory input signal,simulated quadruped robot,specific learning structures
AI 理解论文
溯源树
样例
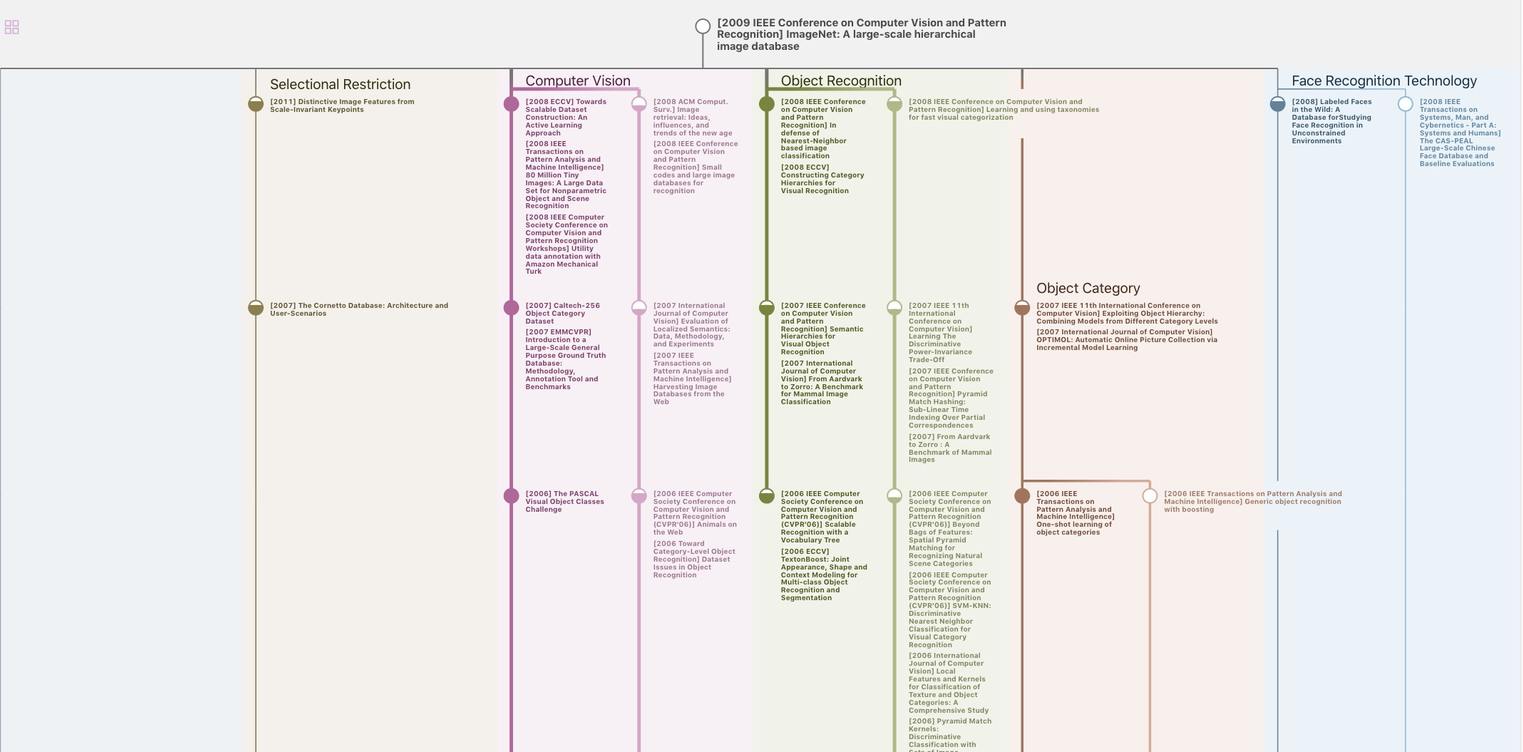
生成溯源树,研究论文发展脉络
Chat Paper
正在生成论文摘要