Tolerating Device-to-Device Variation for Memristive Crossbar-Based Neuromorphic Computing Systems: A New Bayesian Perspective
2023 INTERNATIONAL JOINT CONFERENCE ON NEURAL NETWORKS, IJCNN(2023)
摘要
Memristive crossbar-based architecture provides an energy-efficient platform to accelerate neural networks (NNs) thanks to its Processing-in-Memory (PIM) nature. However, the device-to-device variation (DDV), which is typically modeled as Lognormal distribution, deviates the programmed weights from their target values, resulting in significant performance degradation. This paper proposes a new Bayesian Neural Network (BNN) approach to enhance the robustness of weights against DDV. Instead of using the widely-used Gaussian variational posterior in conventional BNNs, our approach adopts a DDV-specific variational posterior distribution, i.e., Lognormal distribution. Accordingly, in the new BNN approach, the prior distribution is modified to keep consistent with the posterior distribution to avoid expensive Monte Carlo simulations. Furthermore, the mean of the prior distribution is dynamically adjusted in accordance with the mean of the Lognormal variational posterior distribution for better convergence and accuracy. Compared with the state-of-the-art approaches, experimental results show that the proposed new BNN approach can significantly boost the inference accuracy with the consideration of DDV on several well-known datasets and modern NN architectures. For example, the inference accuracy can be improved from 18% to 74% in the scenario of ResNet-18 on CIFAR-10 even under large variations.
更多查看译文
关键词
device-to-device variation,neuromorphic computing,bayesian inference
AI 理解论文
溯源树
样例
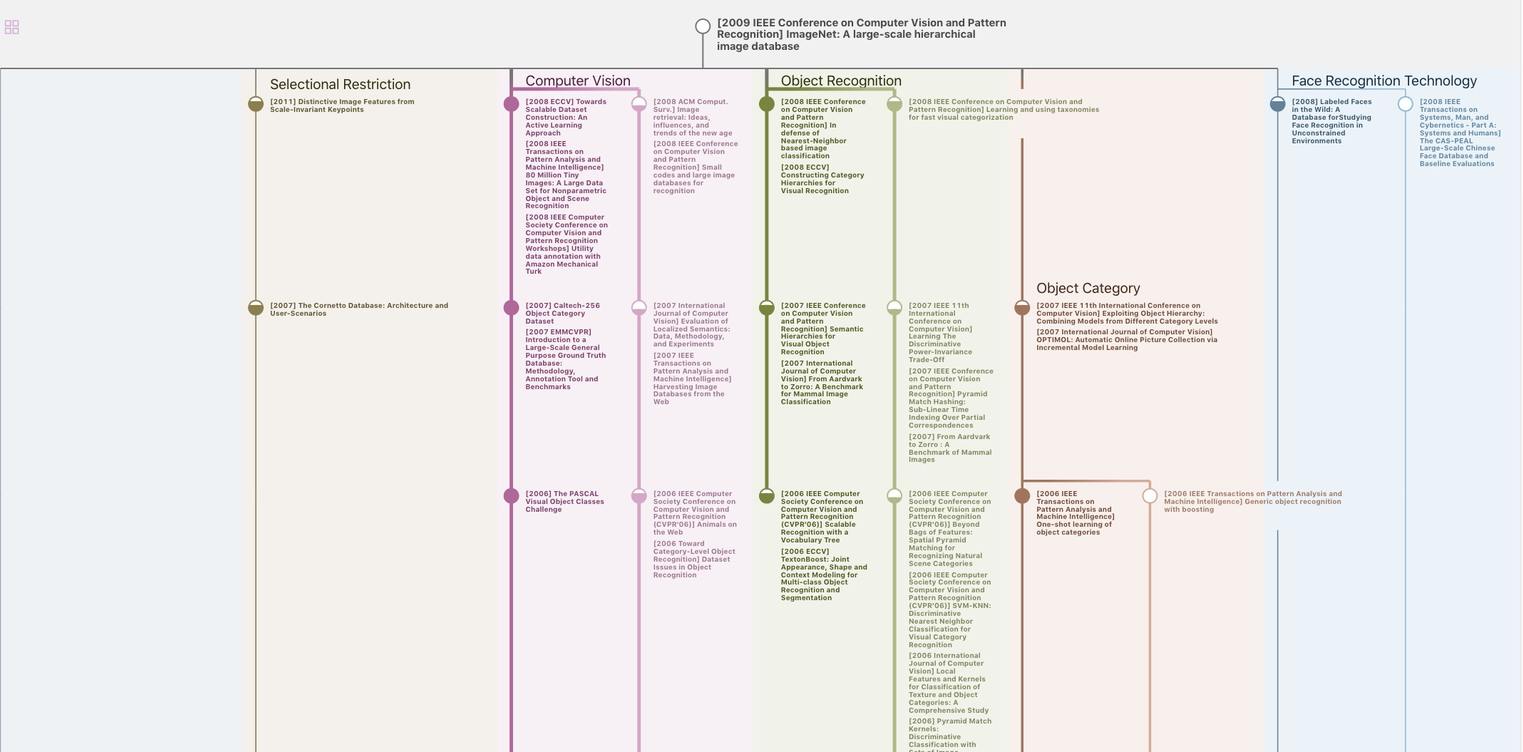
生成溯源树,研究论文发展脉络
Chat Paper
正在生成论文摘要