Flexible Contribution Estimation Methods for Horizontal Federated Learning.
IEEE International Joint Conference on Neural Network(2023)
摘要
Federated Learning (FL), as an emerging distributed learning paradigm to protect data privacy, facilitates model training collaborations among entities. However, performance of FL model in such a promising paradigm would drop dramatically without adequate participating entities' data. To maintain sustainability and efficiency of FL system, it is inevitable to design a reasonable and fair contribution estimation method. Despite of success, existing studies of contribution estimation do not adequately balance efficiency and fairness. To overcome such a limitation, we propose two plug-and-play methods, named as vector projection method (VPM) and income exclusion method (IEM), to estimate the contribution of each entity in FL. As standalone contribution measurement modules, they can be deployed to almost any FL server flexibly. We conduct extensive experiments on widely-used Fashion-MNIST and CIFAR-10 datasets. The experimental results demonstrate the promising effectiveness of contribution estimation, and show the convergence acceleration under contribution-weighted aggregation mechanism.
更多查看译文
关键词
federated learning,contribution estimation
AI 理解论文
溯源树
样例
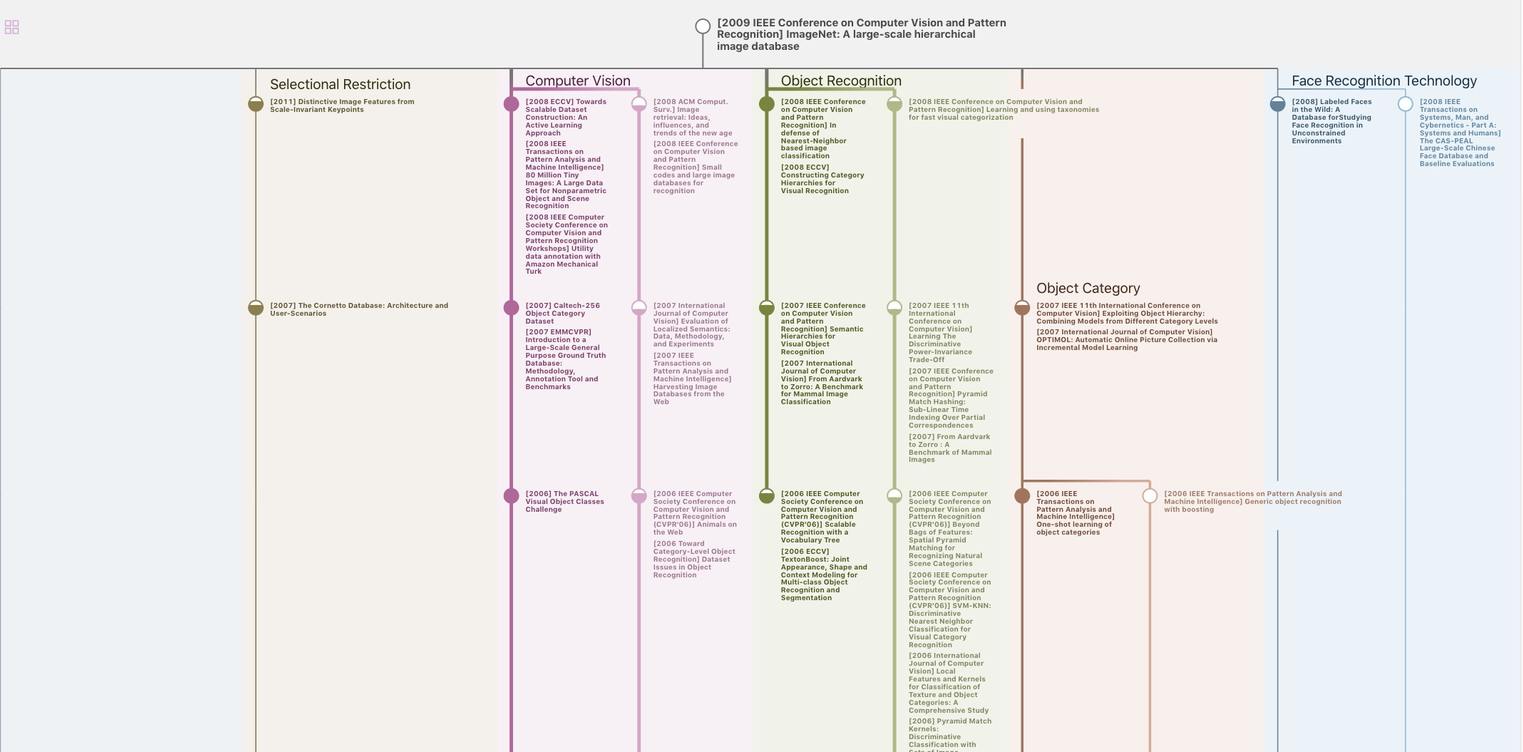
生成溯源树,研究论文发展脉络
Chat Paper
正在生成论文摘要