PointCMT: An MLP-Transformer Network for Contrastive Learning of Point Representation.
IJCNN(2023)
摘要
The irregular and unordered structure makes deep learning based point cloud analysis become a challenging task. Although the recent success of Transformer frameworks in Natural Language Processing (NLP) leads to a series of key advances in dealing with point cloud, most approaches in this category mainly focus on global information representation. In this paper, we propose a Point based MLP-Transformer Contrastive Learning architecture, termed as PointCMT. Specifically, by the introduction of the key ideas and advantages behind the MLP and Transformer, a locally Multi-scale Contrastive Feature Representation Learning module is developed as the core component of our network, which involves a local neighborhood embedding layer, and a contrastive learning model with our well-designed MLP-Transformer feature extractor (MFormer) as fundamental blocks. Experimental evidences on public benchmark datasets indicate that, compared with most state-of-the-arts, our proposed framework can obtain competitive or even better performance in terms of 3D point cloud analysis tasks. The code and trained models will be available at Github.
更多查看译文
关键词
Point Cloud, MLP, Transformer, Contrastive Learning, Classification, Segmentation
AI 理解论文
溯源树
样例
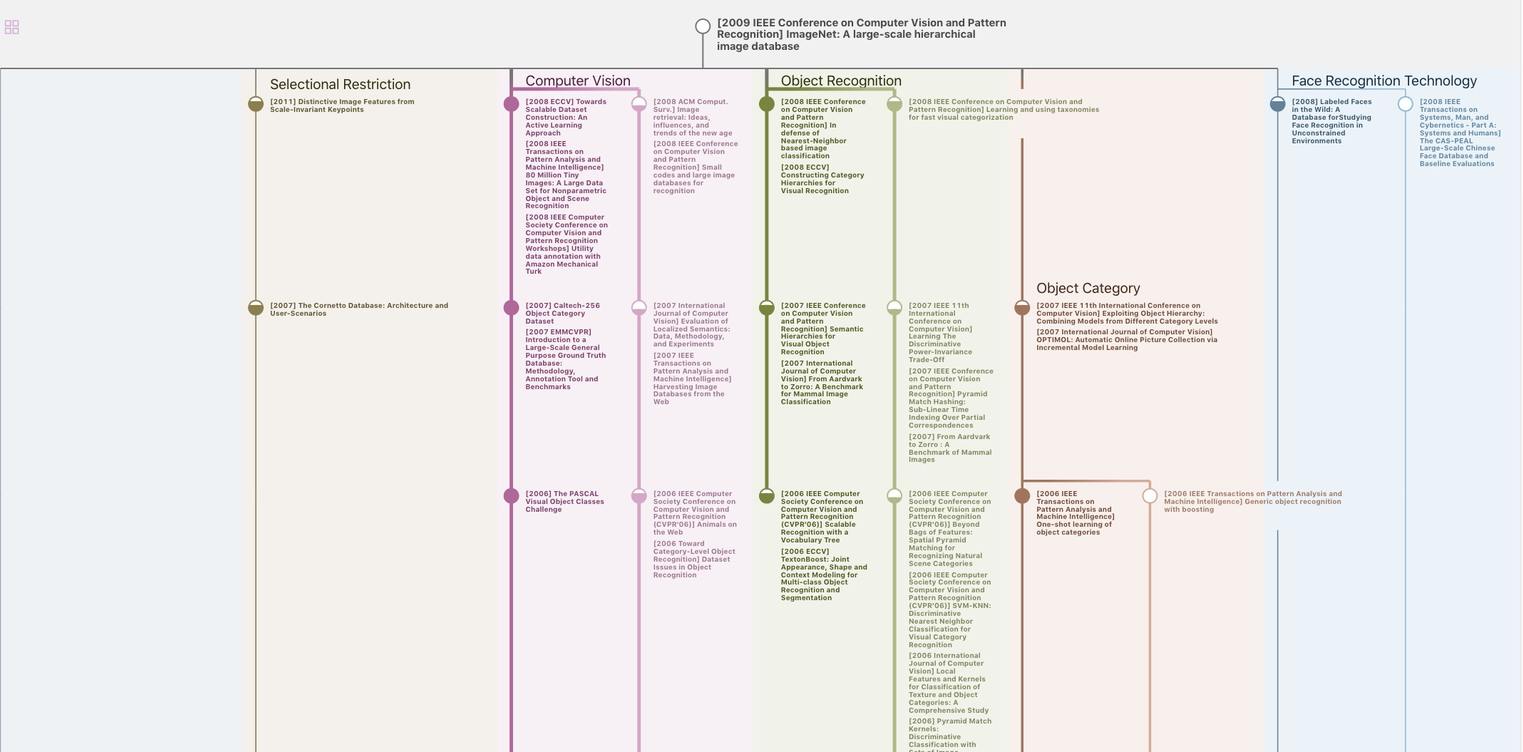
生成溯源树,研究论文发展脉络
Chat Paper
正在生成论文摘要