Enhanced Named Entity Recognition through Joint Dependency Parsing.
IJCNN(2023)
摘要
Named entity recognition (NER) is the task of identifying and classifying named entities from texts. NER can benefit from linguistic dependency information, yet existing NER models can only utilize such information on datasets where dependency annotations are readily available. Dependency parsing (DP) models can be used to generate annotations, which are trained independent of the NER task and can cause error propagation to NER. In this paper, we propose a joint NER and DP model through multi-task learning, which allows the NER and DP modules to benefit from the joint training and provides an end-to-end solution to dependency-guided NER. Our model JOINDER uses a shared contextualized embedder, a word encoder, a biaffine dependency classifier, and a multi-hop dependency-guided NER. Experiments on several standard datasets in four languages show the effectiveness of joint learning and the outstanding performance of JOINDER compared to existing models. Moreover, our model can transfer dependency knowledge to other datasets with no dependency annotat
更多查看译文
关键词
biaffine dependency classifier,dependency annotations,dependency knowledge,dependency parsing models,DP model,enhanced named entity recognition,error propagation,joint dependency parsing,joint learning,joint NER,joint training,linguistic dependency information,model JOINDER,multihop dependency guided NER,multitask learning,NER models,NER task,shared contextualized embedder,word encoder
AI 理解论文
溯源树
样例
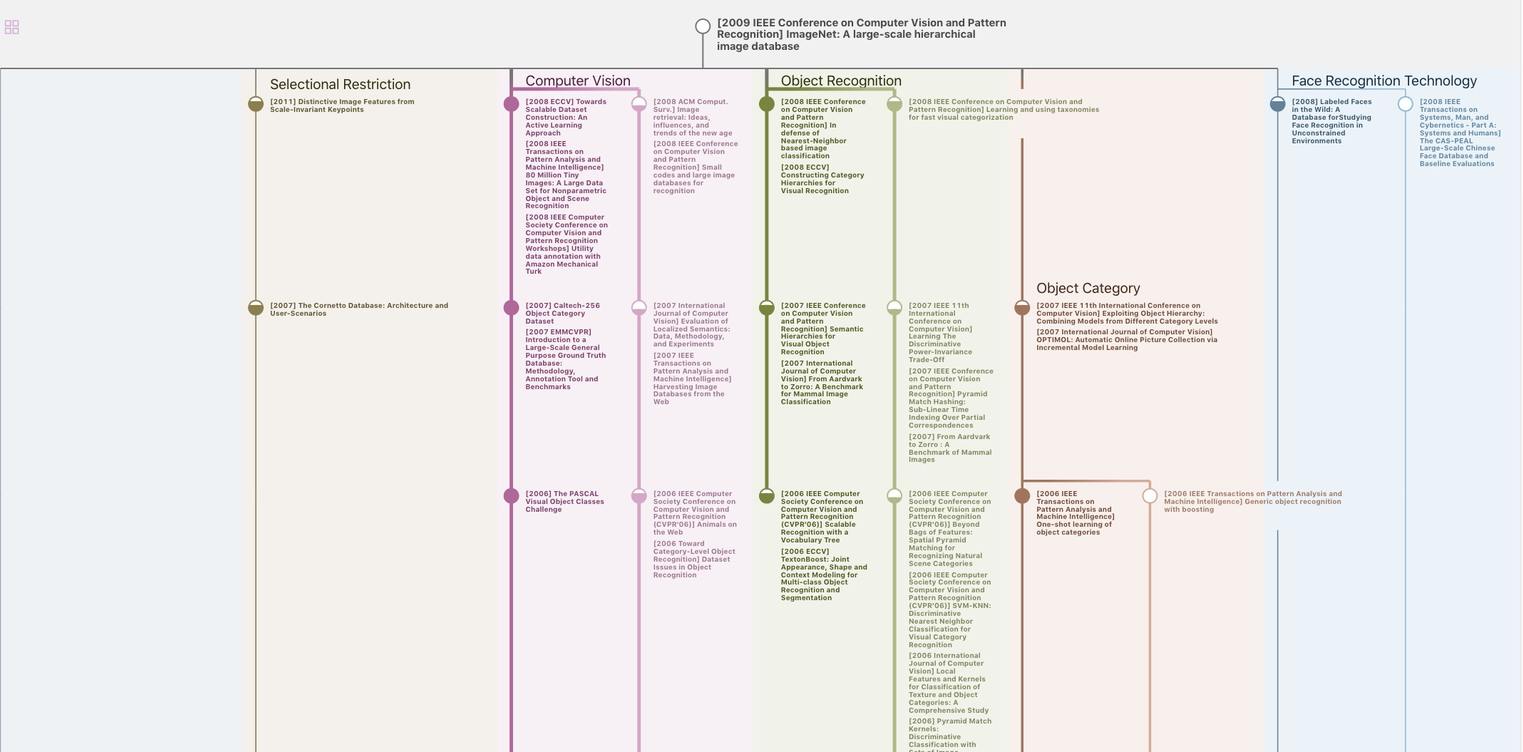
生成溯源树,研究论文发展脉络
Chat Paper
正在生成论文摘要