Event-Guided Attention Network for Low Light Image Enhancement.
IJCNN(2023)
摘要
In the low-light conditions, images are corrupted by low contrast and severe noise, but event cameras can capture event streams with clear edge structures. Therefore, we propose an Event-Guided Attention Network for Low-light Image Enhancement (EGAN) using a dual branch Network and recover clear structure with the guide of events. To overcome the lack of paired training datasets, we first synthesize the dataset containing low-light event streams, low-light images, and the ground truth (GT) normal-light images. Then, we develop an end-to-end dual branch network consisting of a Image Enhancement Branch (IEB) and a Gradient Reconstruction Branch (GRB). The GRB branch reconstructs image gradients using events, and the IEB enhances low-light images using reconstructed gradients. Moreover, we develops the Attention based Event-Image Feature Fusion Module (AEIFFM) which selectively fuses the event and low-light image features using the spatial and channel attention mechanism, and the fused features are concatenated into the IEB and GRB, which respectively generate the enhanced images with clear structure and more accurate gradient images. Extensive experiments on synthetic and real datasets demonstrate that the proposed EGAN produces visually more appealing enhancement images, and achieves a good performance in structure preservation and denoising over state-of-the-arts.
更多查看译文
关键词
accurate gradient images,clear edge structures,clear structure,end-to-end dual branch network,enhancement images,event cameras,Event-guided attention network,Event-Guided Attention Network,Event-Image Feature Fusion Module,Gradient Reconstruction Branch,GRB branch reconstructs image gradients,ground truth normal-light images,Image Enhancement Branch,low light image enhancement,low-light conditions,low-light event streams,Low-light Image Enhancement,low-light image features,low-light images
AI 理解论文
溯源树
样例
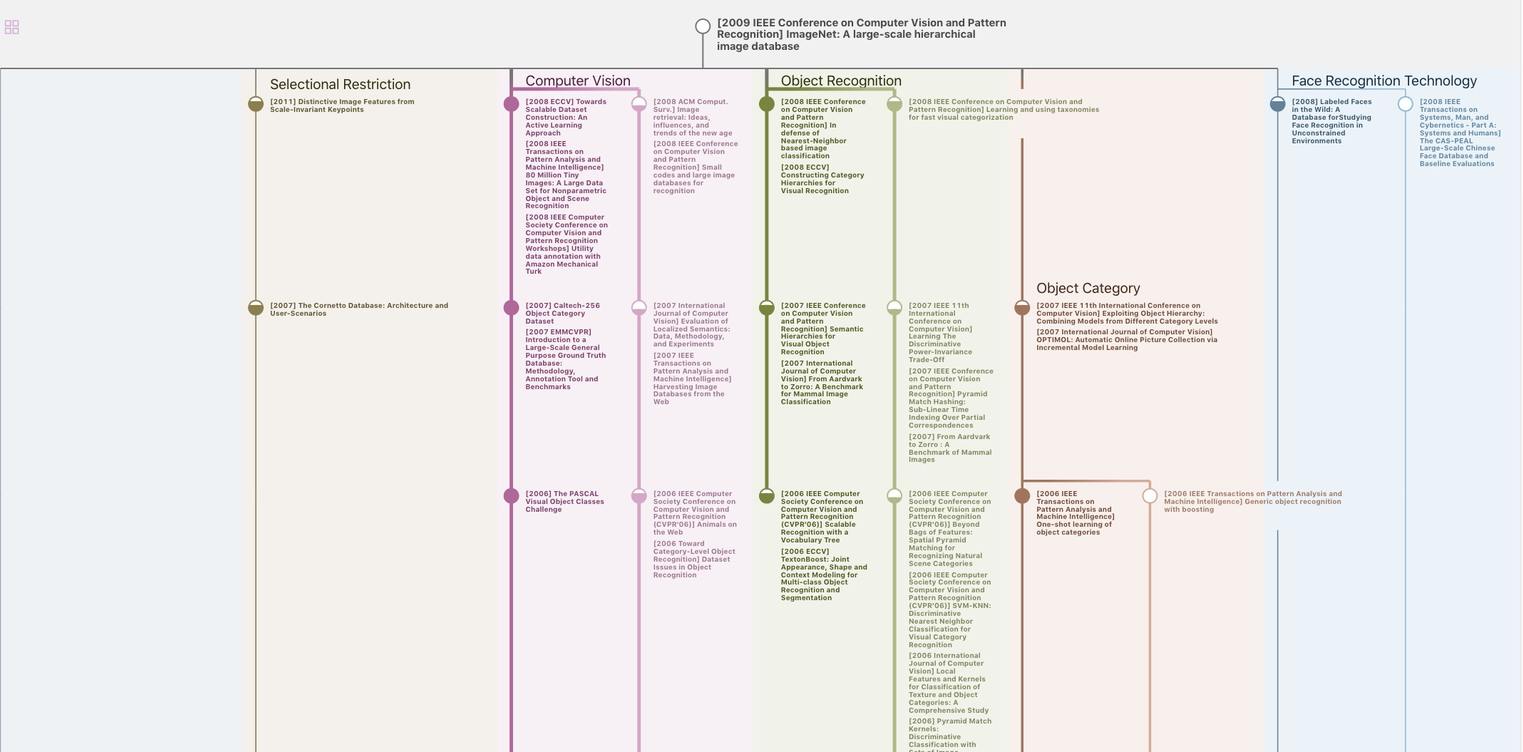
生成溯源树,研究论文发展脉络
Chat Paper
正在生成论文摘要