Boosting Aerial Object Detection Performance via Virtual Reality Data and Multi-Object Training.
IJCNN(2023)
摘要
Deep neural network (DNN) architectures, such as R-CNN and YOLO, have demonstrated impressive performance in object detection tasks with respect to both time and accuracy. However, detecting small aerial objects remains challenging from both a data and algorithmic perspective. Collecting and annotating videos to detect small aerial objects is a time-consuming task and can quickly become a burden when new classes of objects are added to a database. In addition, the current objective functions for DNNs are not specifically designed for smaller objects. To address these challenges, we propose a virtual reality (VR) dataset for aerial object detection, which can generate large volumes of small-object aerial data. By combining VR data with real-world data, we are able to improve the performance of aerial object detection. We also introduce a cost function derived from the normalized Wasserstein distance to replace the Intersection-over-Union loss for YOLO. Experimental results demonstrate that the VR dataset and normalized Wasserstein distance improve the performance of state-of-the-art object detection methods in detecting small aerial objects. Our source code is publicly available at https://github.com/naddeok96/yolov7 mavrc
更多查看译文
关键词
Object Detection,Virtual Reality,YOLO,Normalized Wasserstein distance
AI 理解论文
溯源树
样例
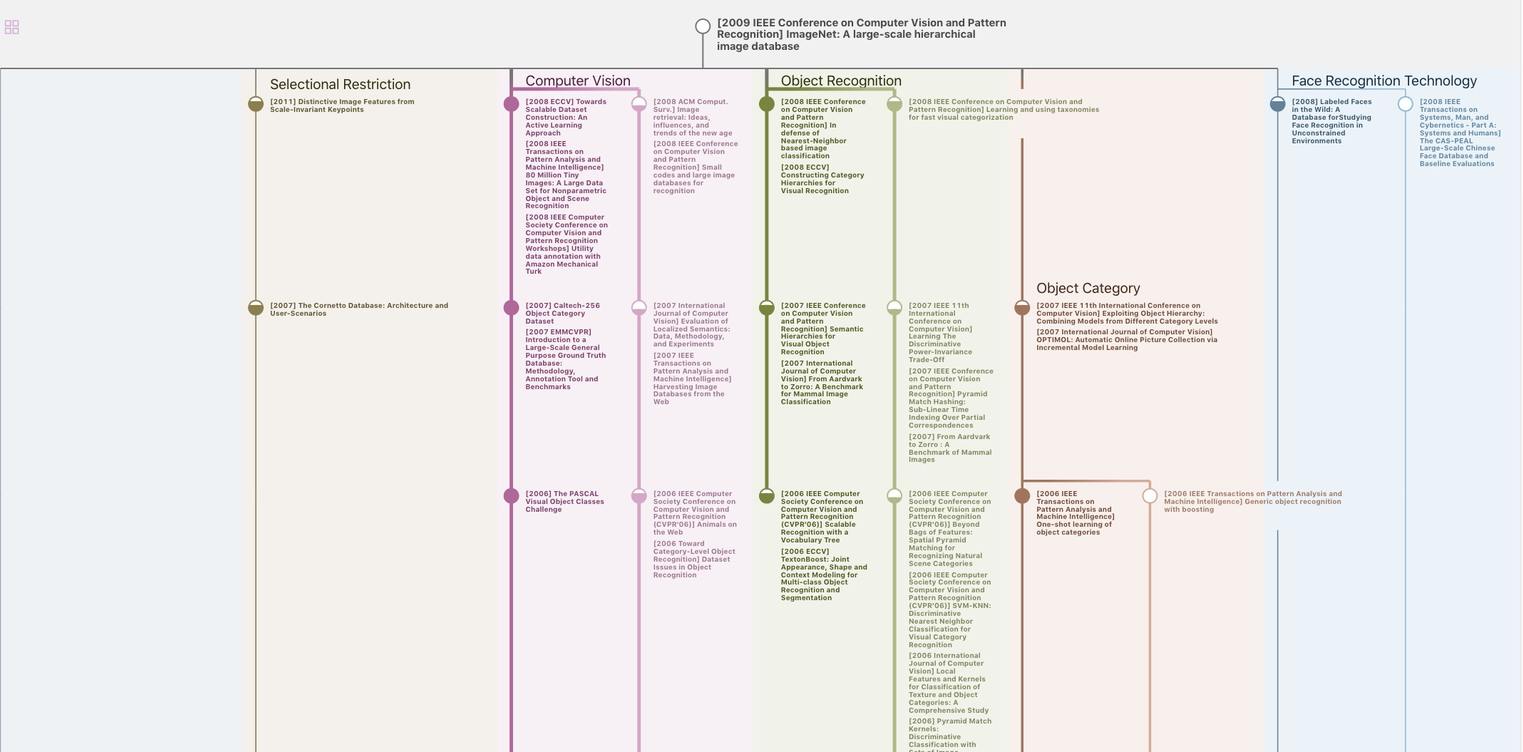
生成溯源树,研究论文发展脉络
Chat Paper
正在生成论文摘要