Detection of retinal microlesions through YOLOR-CSP architecture and image slicing with the SAHI algorithm.
IJCNN(2023)
摘要
Diabetic retinopathy affects millions of working-age people worldwide. Of the countries in Latin America, Brazil has the highest incidence of cases. Diabetic retinopathy is detected through images of the fundus that contain lesions such as hard exudates, soft exudates, microaneurysms, and hemorrhages. Early identification of these lesions prevents the progression of the disease, which leads to a decrease in visual capacity. In addition, the early identification of these lesions allows the screening of patients who need priority care. The detection of these lesions occurs through the processing and analysis of fundus images using deep learning models. In this work, we present a new method that uses the You Only Learn One Representation with Cross Stage Partial Network (YOLOR-CSP) architecture combined with the Slicing Aided Hyper Inference (SAHI) framework to detect lesions. The proposed method was trained, adjusted, and evaluated using the Dataset for Diabetic Retinopathy (DDR) and the Indian Diabetic Retinopathy Image Dataset (IDRiD). The proposed method obtained in the data set DDR mAP equal to 38.08%, in the validation set, and 22.25% in the test set with SGD optimizer. The presented results were superior in the detection of eye fundus lesions in comparison with similar works found in the state-of-the-art literature.
更多查看译文
关键词
diabetic retinopathy, retinal microlesions detection, fundus image, SAHI, YOLOR
AI 理解论文
溯源树
样例
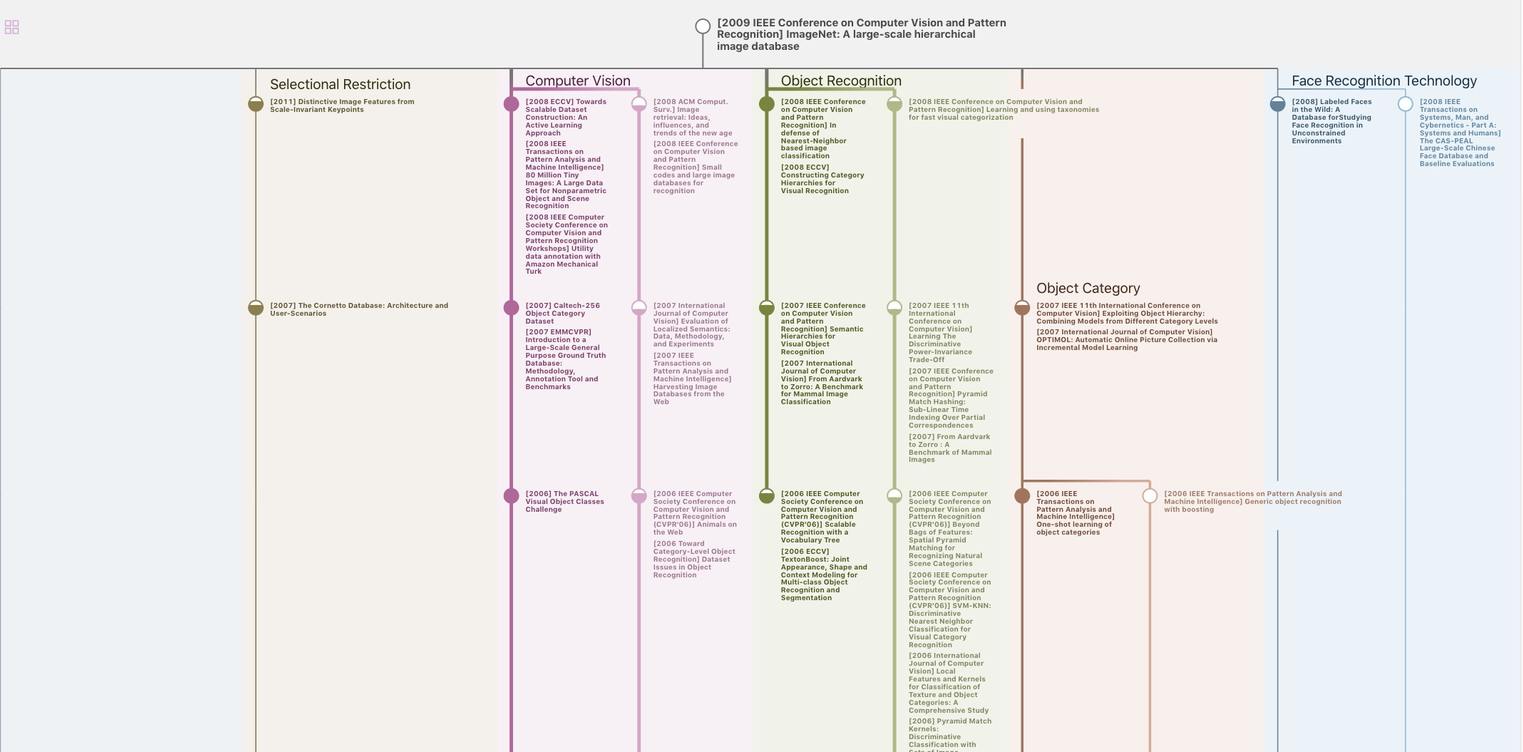
生成溯源树,研究论文发展脉络
Chat Paper
正在生成论文摘要