Assessing Forgetfulness in Data Stream Learning - The Case of Hoeffding AnyTime Tree Algorithm.
EGOV(2023)
摘要
Many efforts around the world have emerged on regulations concerning personal management data guarantee, being one of them related to the ‘Right to Be Forgotten’. There are many divergences on what type of data must be considered in this matter. If some governmental policy interprets that some data collected in a given domain is property of an individual, and this individual has the right to request forgetfulness of this data portion, this data must be erased from third-party tools and services, including e-government services. One important challenge in this scenario is when these data portions have been used for constructing machine learning-based models, as the knowledge composing these models were partially obtained by the data to be forgotten. Moreover, there can be of special interest when it is demanded to a company to forget huge parts of their source data, which can lead to lower quality estimators. So, it is fundamental to present machine learning tools to support these types of policies as well as investigating the impact of data forgetting to machine learning-based estimators. In this paper, we investigate the impact of these learning and forgetting policies in Data Stream Learning (DSL) using an algorithm called Hoeffding AnyTime Tree (HATT). This is an interesting algorithm as it incorporates the ability to negatively weighting instances, which can be seen as a property of data forgetting. We subject the HATT algorithm to 4 levels of forgetting and investigate the impact of data forgetting in the obtained predictive performance. They are compared against control instances (upper and lower bound) of the HATT algorithm using four non-stationary stream datasets. Our results showed that as the forgetting rate increases, the model approaches the lower bound behavior in terms of accuracy for 2 out of 4 datasets, indicating that this is a promising approach.
更多查看译文
关键词
data stream learning,hoeffding anytime tree algorithm,forgetfulness
AI 理解论文
溯源树
样例
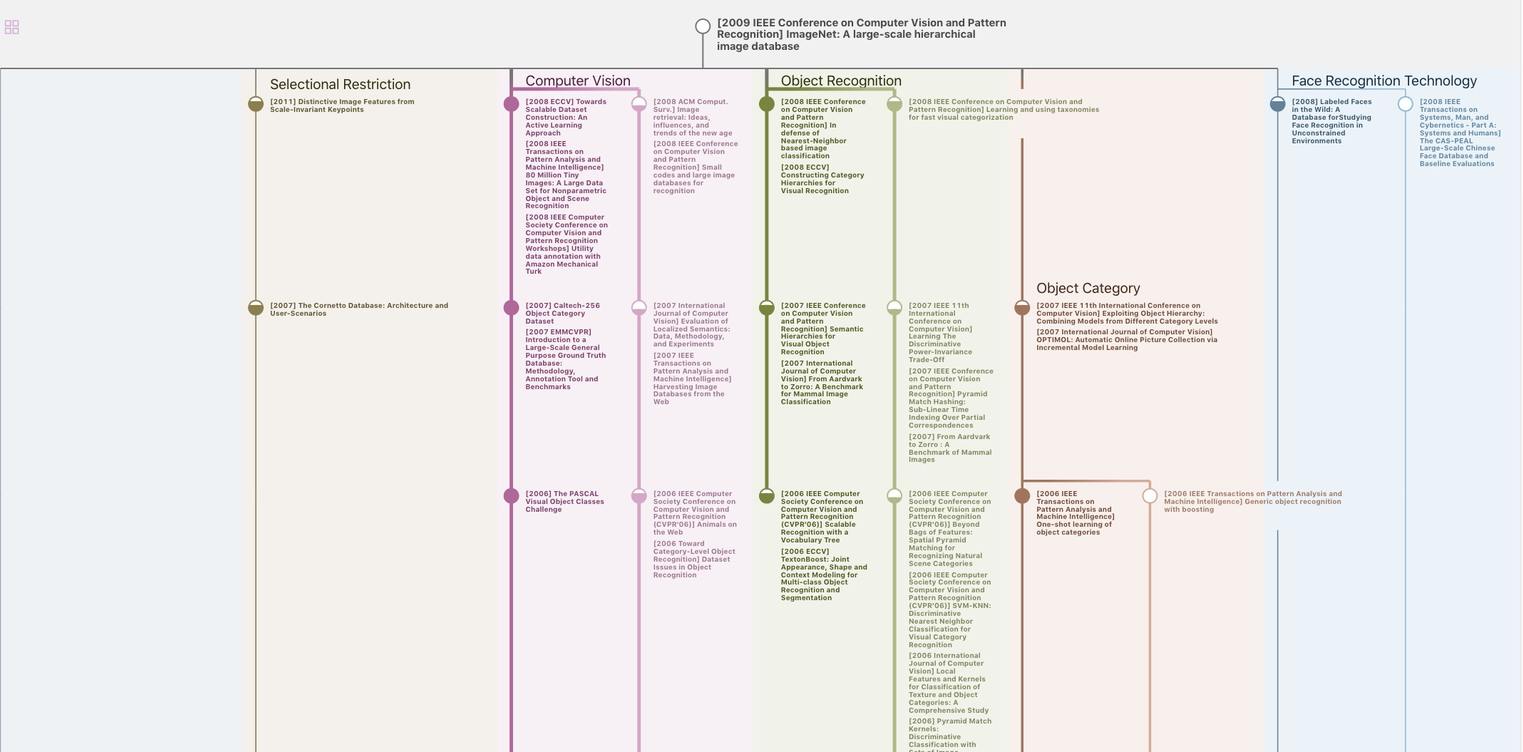
生成溯源树,研究论文发展脉络
Chat Paper
正在生成论文摘要