When Whisper Meets TTS: Domain Adaptation Using only Synthetic Speech Data.
TSD(2023)
摘要
Automatic Speech Recognition is among the most important areas of Artificial Intelligence research today. One of the most notable advances in this area is the development of end-to-end models, which have shown state-of-the-art performance in many benchmark scenarios. In spite of the recent improvements, these architectures still require large amounts of transcribed speech data to be trained, which can be challenging in low resource languages, or in specific domains due to privacy concerns. This study proposes a methodology to fine-tune Whisper-based models using only synthetic speech. The aim is to enable training robust systems for specific domains and low resource languages, where large labeled corpora are difficult to collect. Our approach is based on a language model adaptation by fine-tuning only the decoder of the model, thus the network is able to learn specific vocabulary that is not initially available. The proposed methodology is evaluated with data from different languages and domains. In addition, Parameter Efficient Fine-Tuning strategies were used to efficiently adapt the large pre-trained Whisper models. This is one of the first studies that considers the effect of using only synthetic speech for domain adaption of speech recognition systems in non-English data, providing word error rate reductions in low resource languages between 2 and 30 points, depending on the Whisper version.
更多查看译文
关键词
domain adaptation,tts,whisper,speech
AI 理解论文
溯源树
样例
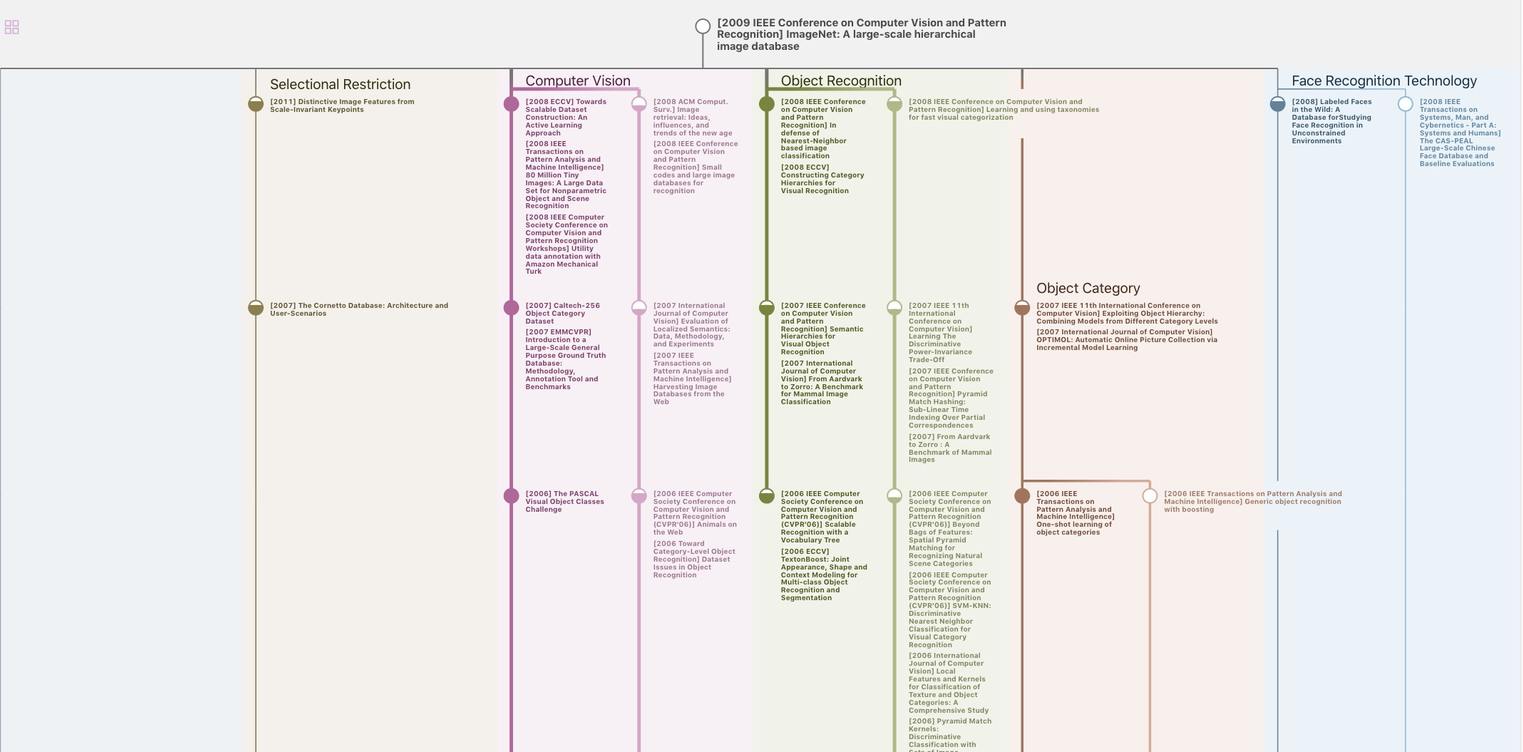
生成溯源树,研究论文发展脉络
Chat Paper
正在生成论文摘要