A multi-view anomalous co-location detection framework considering both intra- and inter-feature couplings.
MDM(2023)
Abstract
Co-location is one of the most fundamental spatial associations in spatial data. Previous anomalous co-location detections only aim to detect abnormal inter-feature co-locations on bivariate datasets, while the abnormal intra-feature co-locations are lost, which may have limits to applications. Thus, we propose a novel multi-view anomalous co-location detection framework (MACDF). Two kinds of relationships are captured by two proposed couplings, that is, intra-feature coupling and inter-feature coupling (intra-coupling and inter-coupling for short). Moreover, the neighboring concept in co-location relationships is redefined with a mixture-considered inter-coupling for point-like instances, inspired by the concept for polygonal instances, and a concreted algorithm, i.e. multi-view low-rank analysis with direction for anomalous co-location detection (MLAD-ACD), is designed to discover the anomalous co-locations, on not only bivariate datasets but also multivariate datasets. Experiments show the instantiated algorithm MLAD-ACD can detect anomalous co-locations effectively and efficiently on spatial datasets. Specifically, MLAD-ACD obtains at least a 71% higher AUC score than the state-of-the-art algorithms in an acceptable time.
MoreTranslated text
Key words
Spatial Data Mining,Anomalous Co-location Detection,Coupling,Anomalous Region
AI Read Science
Must-Reading Tree
Example
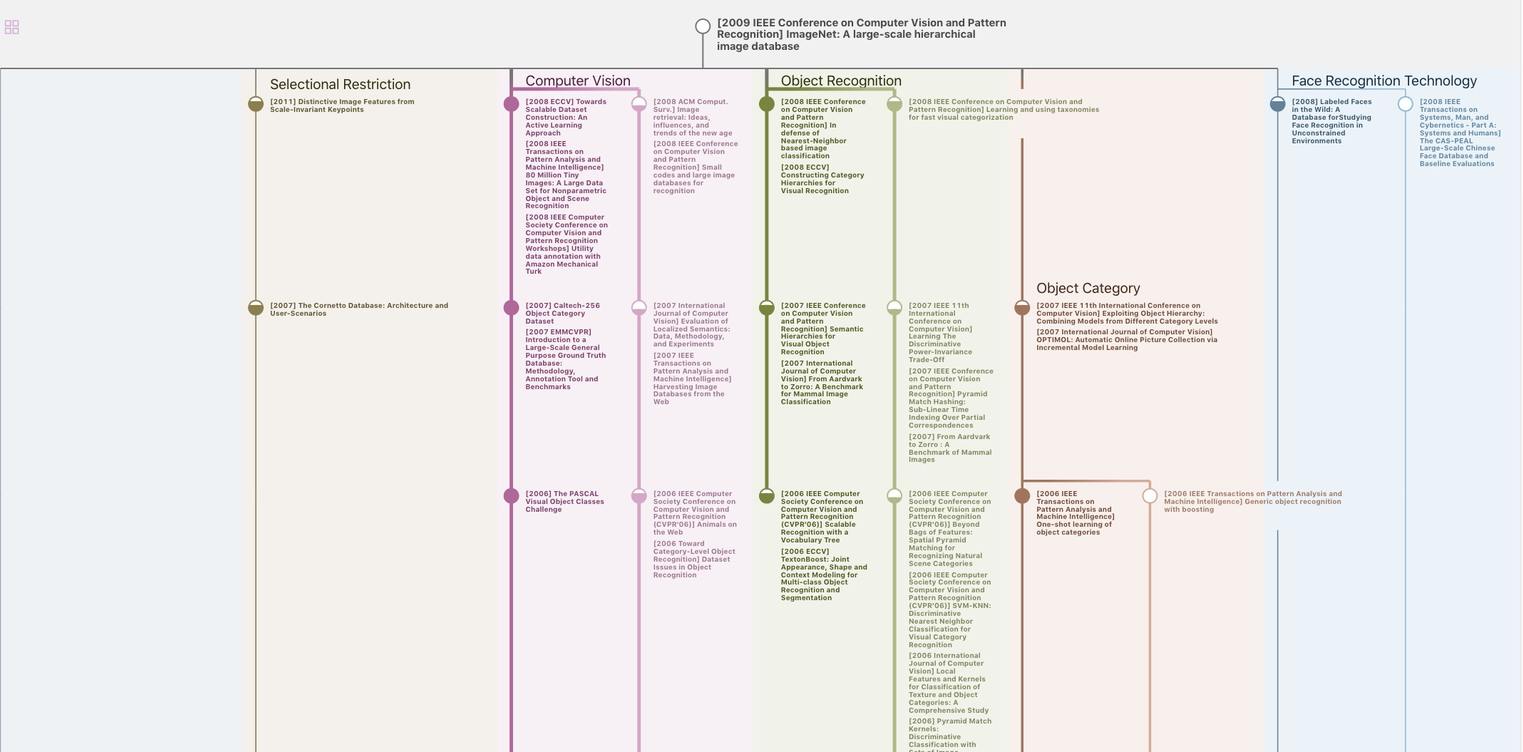
Generate MRT to find the research sequence of this paper
Chat Paper
Summary is being generated by the instructions you defined