Improving Stock Trend Prediction with Multi-granularity Denoising Contrastive Learning.
IJCNN(2023)
摘要
Stock trend prediction (STP) aims to predict the price fluctuation, which is critical in financial trading. The existing STP approaches only use market data with the same granularity (such as daily market data). However, in the actual financial investment, there are a large number of more detailed investment signals contained in finer-grained data (e.g. high-frequency data). This motivates us to research how to leverage multi-granularity market data to capture more useful information and improve the accuracy in the task of STP. However, the effective utilization of multi-granularity data presents a major challenge. Firstly, the iteration of multi-granularity data with time will lead to more complex noise, which makes it difficult to identify and extract it. Secondly, the difference in granularity may lead to opposite target trends in the same time interval. Thirdly, the target trends of stocks with similar features can be quite different, and different sizes of granularity will aggravate this gap. In order to address the above three challenges, in this paper, we present a self-supervised framework of multi-granularity denoising contrast learning (MDC). Specifically, we construct a dynamic dictionary of memory, which can obtain clear and unified representations by filtering noise and aligning multi-granularity data. Moreover, we design two contrast learning objectives to solve the differences in trends by constructing additional self-supervised signals. Extensive experiments on the CSI 300 datasets show that our framework stands out from the existing top-level systems and has excellent profitability in real investing scenarios.
更多查看译文
关键词
Contrastive Learning, Multi-granularity Data, Memory, Denoising, Stock Trend Prediction
AI 理解论文
溯源树
样例
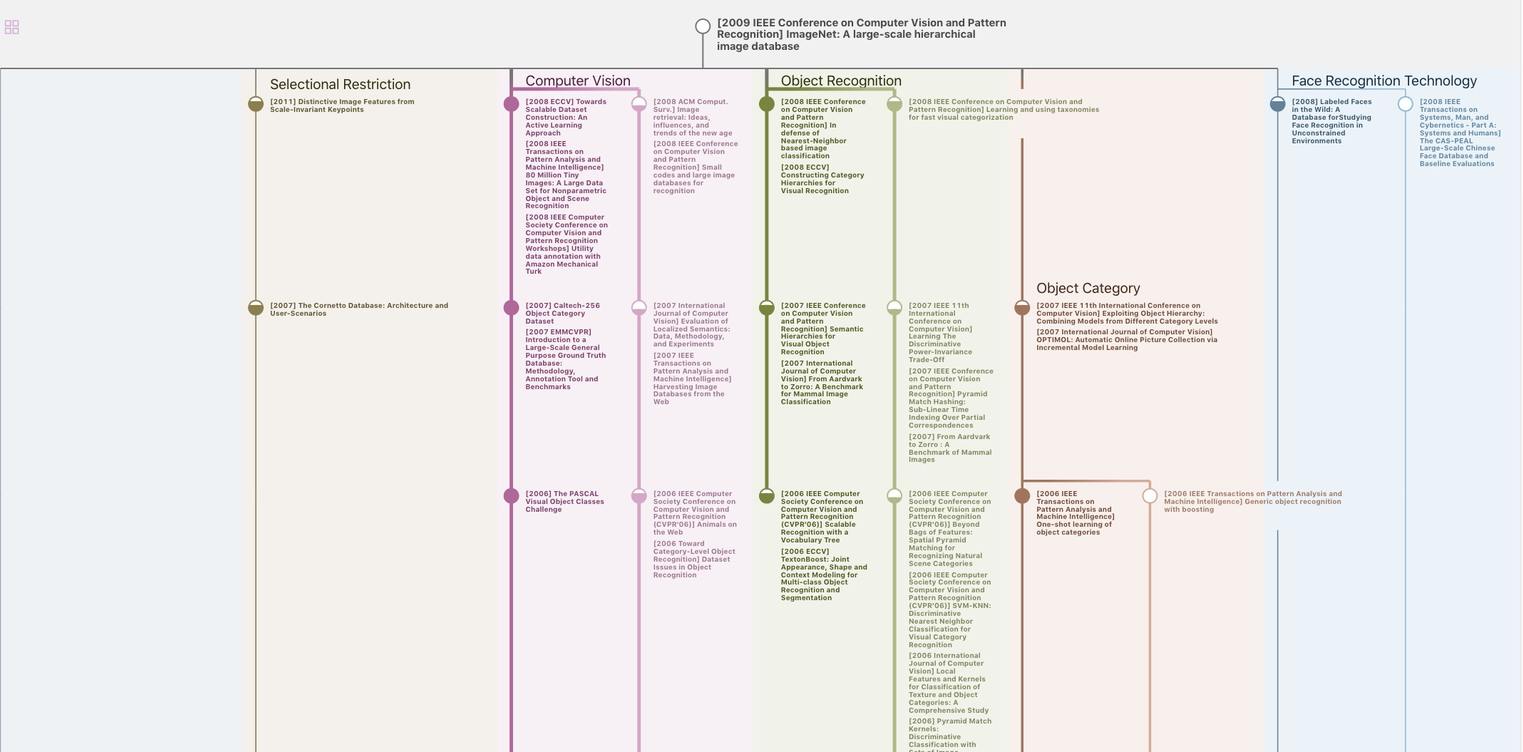
生成溯源树,研究论文发展脉络
Chat Paper
正在生成论文摘要