Online Trajectory Anomaly Detection Based on Intention Orientation.
IJCNN(2023)
摘要
Trajectory anomaly detection has become increasingly important in many fields. However, most existing methods do not support online detection, and have limited performance in multi-source/multi-destination environments. To address these challenges, we propose an unsupervised method named intention orientation based trajectory anomaly detection (IO-TAD) which detects anomalies by inferring their underlying intentions in an online manner. IO-TAD is also robust in multi-source/multi-destination environments. To achieve this, we leverage Inverse Reinforcement Learning and recover the reward functions as a representation of the agents' intentions. Then we use the inferred value functions to quantify the agents' intention orientation pattern. The intuition behind our method is that different normal agents take different routes, or have different intentions, but the ways they achieve their intentions are similar. In other words, the values of normal trajectories tend to be monotonically increasing, while anomalous behaviour is non-monotonic. Our experiments show IO-TAD can achieve good online anomaly detection performance with up to 18.1% improvement in F-1 score over the comparable state-of-the-art methods.
更多查看译文
关键词
Anomaly Detection, Inverse Reinforcement Learning, Intention Prediction
AI 理解论文
溯源树
样例
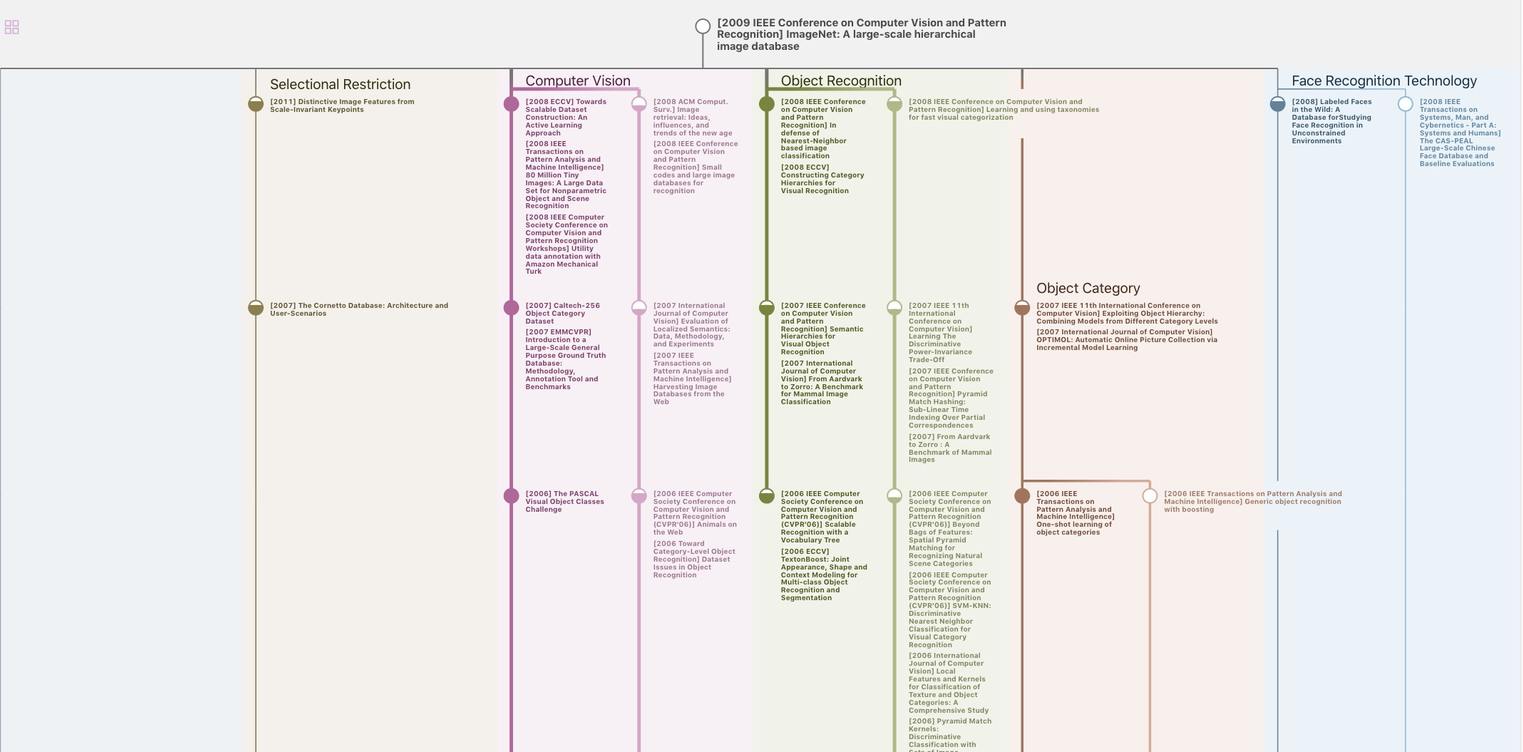
生成溯源树,研究论文发展脉络
Chat Paper
正在生成论文摘要