An Unseen Features Enhanced Text Classification Approach.
IJCNN(2023)
摘要
In this paper, we discuss the issue of features that emerge during the prediction phase of a machine learning model, termed as unseen features. Because unseen features are absent from the vocabulary of the trained model, they are often rejected during the preprocessing stage of the learning model in standard machine learning approaches. We introduce the idea of unseen features and a method for identifying and using them for classification tasks. Because the dimension of feature vector required for trained machine learning model is going to differ upon incorporating unseen features of the testing data sample, it is not practical to directly incorporate unseen features since they only exist during the prediction phase of a machine learning model. As a result, the feature space for the training set is transformed to the embedding space which facilitates the use of unseen features. The proposed approach is empirically evaluated using standard metrics over three benchmark datasets in diverse circumstances (natural and balanced datasets) and on various text types - long-texts (aka structured texts) and short-texts (aka unstructured texts) considering five distinct classification algorithms. The experimental findings confirm the effectiveness of using unseen features during a machine learning model's deployment phase. The proposed unseen features enhanced technique outperforms the conventional approaches in both balanced class distribution and natural class distribution scenarios by a significant margin of at least 10%.
更多查看译文
关键词
Machine learning,Unseen features,Out-of-distribution,Text classification
AI 理解论文
溯源树
样例
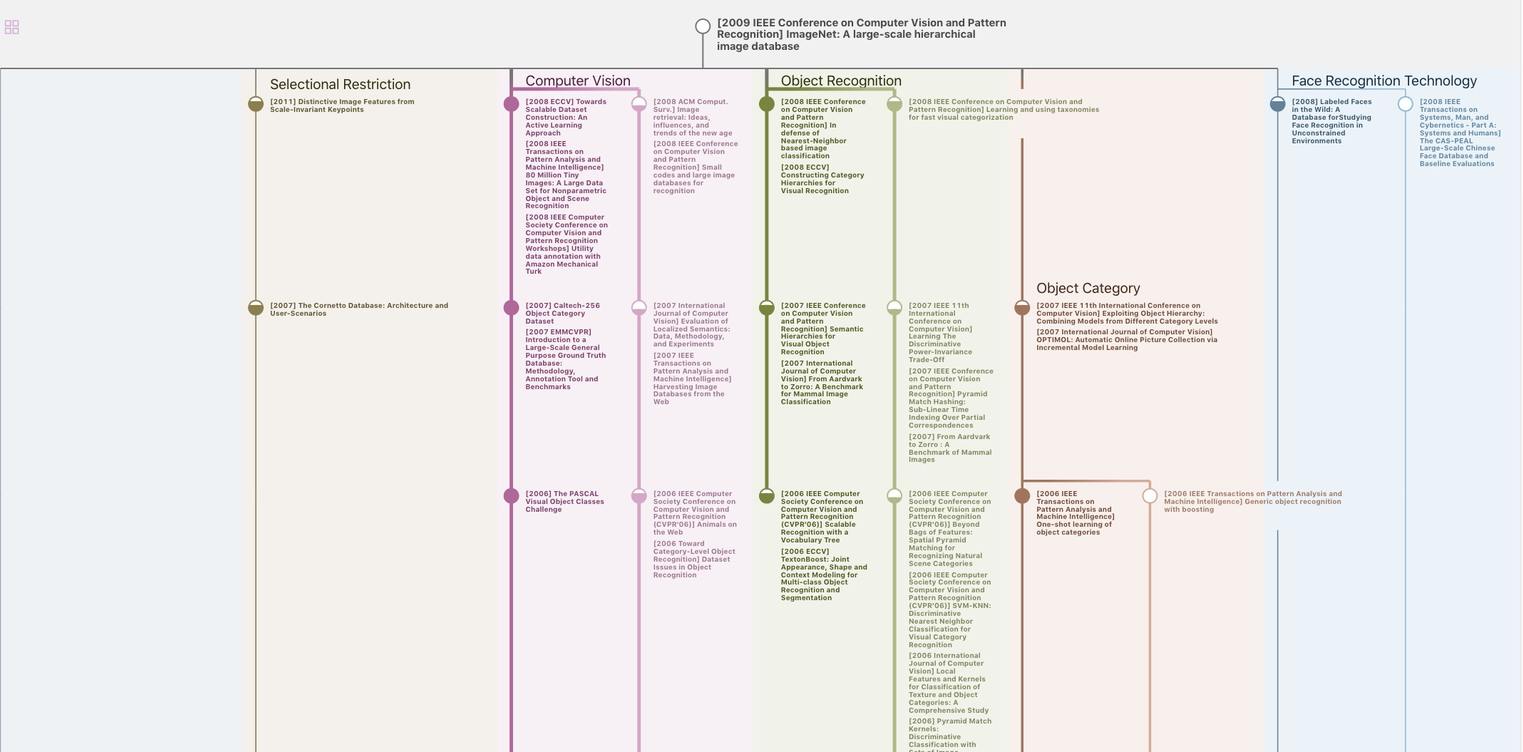
生成溯源树,研究论文发展脉络
Chat Paper
正在生成论文摘要