Bi-ClueMVSNet: Learning Bidirectional Occlusion Clues for Multi-View Stereo.
IJCNN(2023)
摘要
Deep learning-based multi-view stereo (MVS) methods have achieved promising results in recent years. However, very few existing works take the occlusion issues into consideration, leading to poor reconstruction results on the boundaries and occluded areas. In this paper, the Bidirectional Occlusion Cluesbased Multi-View Stereo Network (Bi-ClueMVSNet) is proposed as an end-to-end MVS framework that explicitly models the occlusion obstacle for depth map inference and 3D modeling. To this end, we use bidirectional projection for the first time to reduce the propagation and accumulation of incorrect matches and build the occlusion-enhanced network to further advance the representational ability from 2D visibility maps to 3D occlusion clues. As for depth map estimation, we combine the characteristics of both regression and classification approaches to propose the adaptive depth map inference strategy. Besides, the robustness of the training process is further guaranteed and elevated by the occlusion clues-based loss function. The proposed method significantly improves the accuracy of depth map inference in boundaries and heavily occluded areas and brings the overall quality of the reconstructed point cloud to a new altitude. Extensive experiments are performed on DTU, Tanks and Temples, and BlendedMVS datasets to demonstrate the persuasiveness of the proposed framework.
更多查看译文
关键词
2D visibility maps,3D occlusion clues,adaptive depth map inference strategy,Bi-ClueMVSNet,bidirectional occlusion clues-based multiview stereo network,deep learning-based multiview stereo methods,depth map estimation,DTU,occlusion clues-based loss function,occlusion obstacle,occlusion-enhanced network
AI 理解论文
溯源树
样例
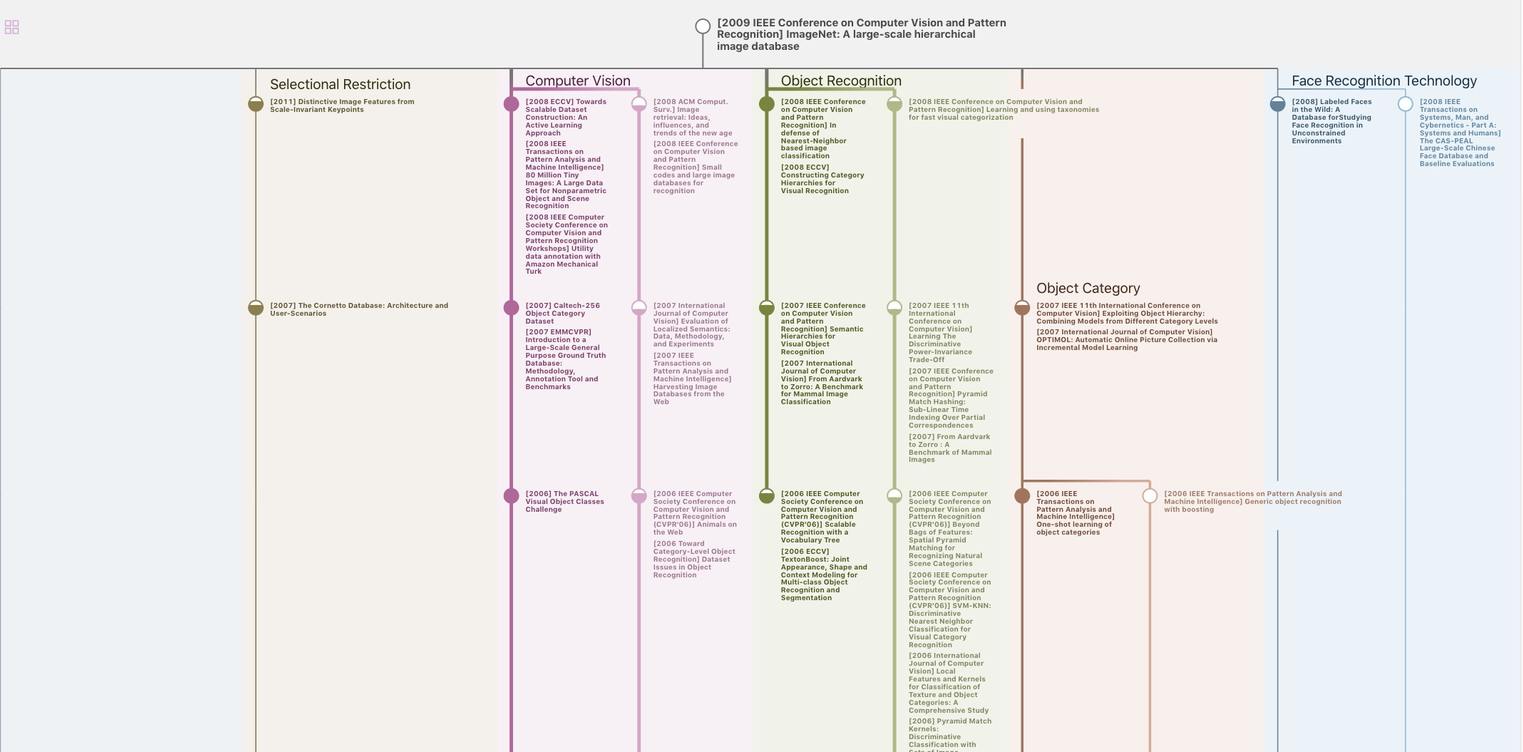
生成溯源树,研究论文发展脉络
Chat Paper
正在生成论文摘要