Disease Diagnosis with Cost-Sensitive Grouped Features Based on Deep Reinforcement Learning.
IJCNN(2023)
摘要
Traditional machine learning algorithms rely on the assumption that all features of a given dataset are available for free. However, in disease diagnosis, acquiring features requires a certain cost because there are many concerns, such as monetary data collection costs, patient discomfort in medical procedures, and privacy impacts of data collection that require careful consideration. Moreover, some medical examination items are grouped in the real world, such as lab tests that return multiple measurements, resulting in naturally grouped features. In this work, to consider features that come in groups, we propose a novel feature selection method for disease diagnosis with cost-sensitive grouped features based on deep reinforcement learning (RL). We optimize the objective which is a tradeoff between the cost of grouped features and the diagnostic accuracy, by training deep RL models. In addition, the approach is flexible, as it can be improved with new deep reinforcement learning algorithms. We evaluate the effectiveness of the proposed method on four publicly available datasets. Compared with the existing competitive feature selection methods, the proposed method can achieve higher learning accuracy with lower feature costs. This paper takes disease diagnosis as an example, and the proposed method is universal for problems with grouped features of different domains. (The source code is available at https://anonymous.4open.science/r/DRL- CSGF-Y821).
更多查看译文
关键词
deep reinforcement learning,cost-sensitive,grouped features,disease diagnosis,trade-off
AI 理解论文
溯源树
样例
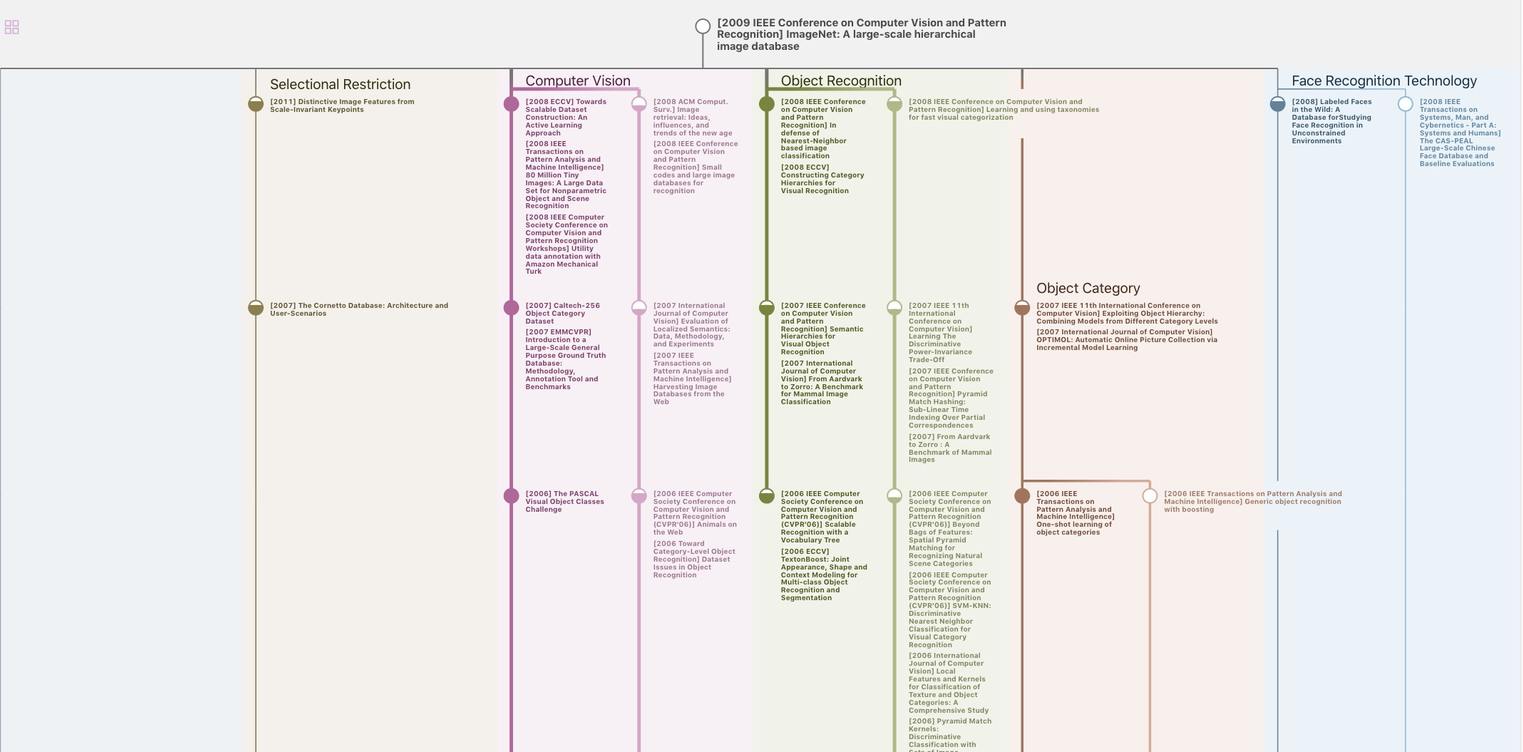
生成溯源树,研究论文发展脉络
Chat Paper
正在生成论文摘要