Privacy-Preserving Federated Learning with Hierarchical Clustering to Improve Training on Non-IID Data.
NSS(2023)
摘要
Federated learning (FL), as a privacy-enhanced distributed machine learning paradigm, has achieved tremendous success in solving the data silo problem. However, data heterogeneity (Non-IID data) between parties (data owners) poses challenges for the vanilla federated learning aggregation approach (FedAvg), including more interaction rounds and lower accuracy of the global model. To address this challenge, some works make improvements based on FedAvg. However, most of these works do not consider the privacy protection of gradients, which would leak private data information about the parties involved in the training. To protect parties’ privacy and enhance the FL training on Non-IID data at the same time, in this paper, we present PPFL+HC, an efficient, private FL framework. Our PPFL+HC follows the state-of-the-art Non-IID FL method (FL+HC IJCNN’20), which presents a modification to FL by introducing a hierarchical clustering step to separate clusters of parties by the similarity of their local gradients, adapting it to the privacy-preserving context. We design a series of secure cryptographic protocols to ensure the privacy of parties. Specifically, first, we use additive secret sharing to protect local gradients and global gradients privacy, while using pseudorandom generation techniques to reduce half the communication overhead. Second, we design a secure and efficient Euclidean distance computation and Manhattan distance computation protocol to accelerate the secure hierarchical clustering process. Finally, to improve the computational efficiency of the clustering process, we perform randomized gradient cropping to reduce the computational overhead while ensuring the accuracy of clustering. Moreover, experiments conducted on two real-world datasets demonstrate that our PPFL+HC achieves secure and efficient FL training for Non-IID data.
更多查看译文
关键词
hierarchical clustering,privacy-preserving,non-iid
AI 理解论文
溯源树
样例
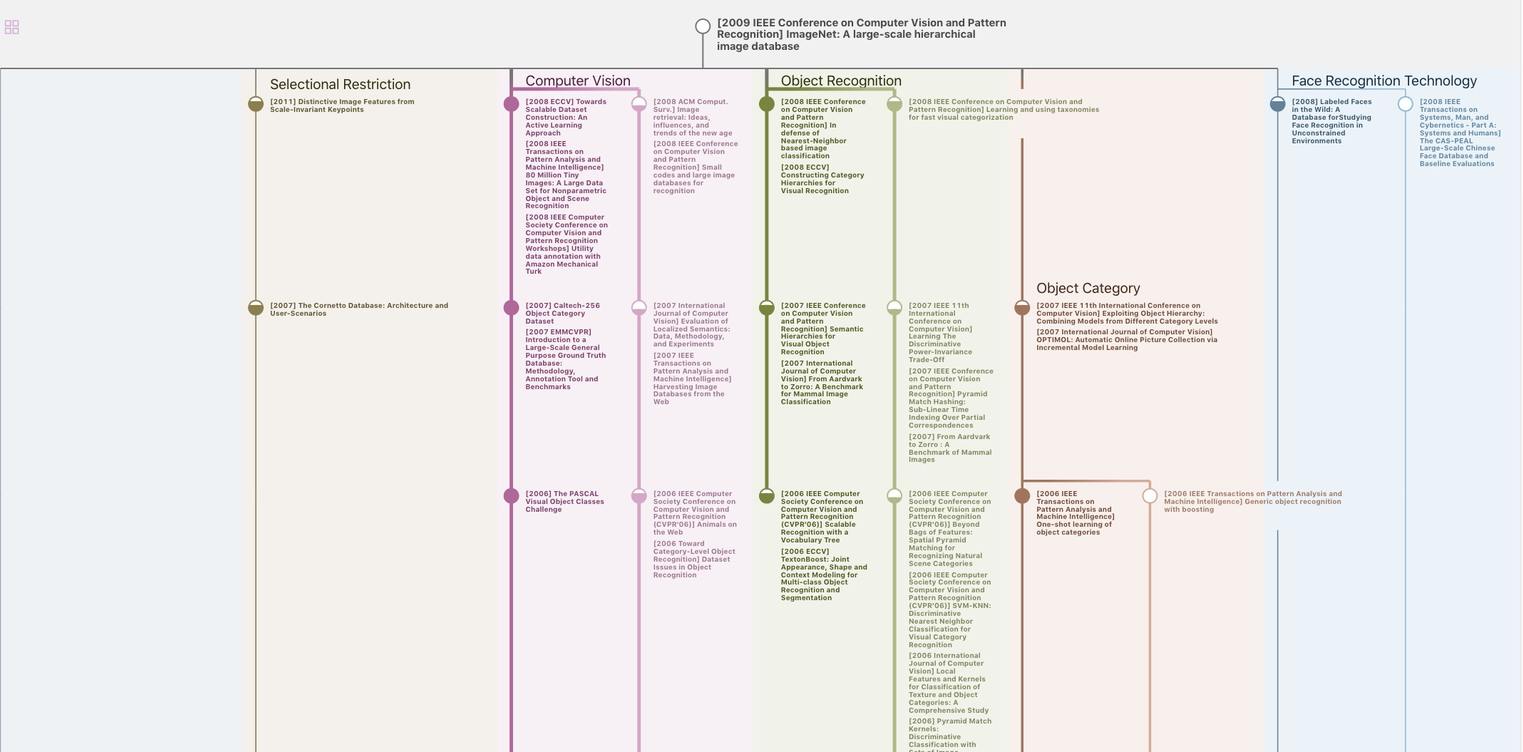
生成溯源树,研究论文发展脉络
Chat Paper
正在生成论文摘要