Deep Feature Extraction for Data Assimilation with Ensemble Smoother
IJCNN(2023)
摘要
History matching is applied to update reservoir parameters, such as the porosity and permeability of the subsurface rocks, according to new indirect observations. Local fluid production and pressure measurements in the drilled wells are the commonly dynamic observations used in the process. Another dynamic reservoir observation is the time-lapse seismic data, consisting of several elastic parameter cubes at different production time steps. This data provide indirect spatial information about the changes in fluid saturation and pressure caused by the fluid flow of the production. Ensemble Smoother Multi-Data Assimilation is presented in the literature as a sound and stable algorithm for solving History Matching problems. The application of this algorithm to seismic data is still a significant challenge due to the dimensionality issues related to the high number of data points of the seismic. Deep learning methods have been successfully applied to learn feature representations for high-dimensional data and represent the original data into latent information with lower dimensions. In this paper, the deep learning method is exploited for feature extraction of time-lapse seismic data and integrated with ES-MDA to update the reservoir parameters. Instead of training a deep model from scratch, we propose using deep models with fully convolutional auto-encoder structures trained with natural images dataset. Different from other proposals, this work can be adapted to any reservoir case through a transfer learning step. The result shows a considered improvement in the ES-MDA update process, reducing the processing time and increasing the ensemble variability between model realizations.
更多查看译文
关键词
Deep Learning,Feature Extraction,Ensemble Smoother,Multiple Data Assimilation
AI 理解论文
溯源树
样例
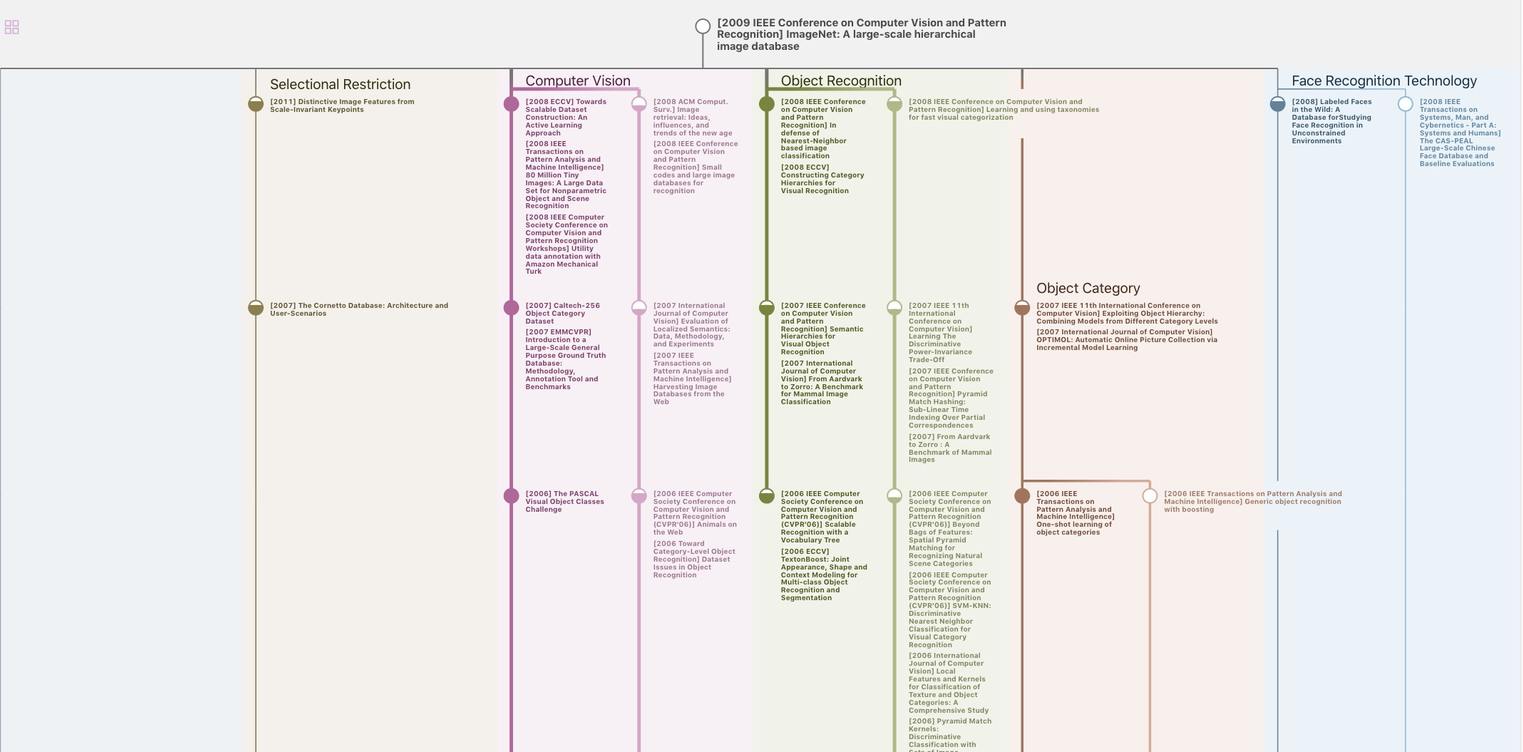
生成溯源树,研究论文发展脉络
Chat Paper
正在生成论文摘要