Enhancing Discriminative Ability among Similar Classes with Guidance of Text-Image Correlation for Unsupervised Domain Adaptation.
IJCNN(2023)
摘要
In deep learning, unsupervised domain adaptation (UDA) is commonly utilized when the availability of abundant labeled data is often limited. Several methods have been proposed for UDA to overcome the difficulty of distinguishing between semantically similar classes, such as person vs. rider and road vs. sidewalk. The confusion of the classes results from the collapse of the distance, caused by the domain shift, between classes in the feature space. In this work, we present a versatile approach based on text-image correlation-guided domain adaptation (TigDA), which maintains a distance to properly adjust the decision boundaries between classes in the feature space. In our approach, the feature information is extracted through text embedding of classes and the aligning capability of the text features with the image features is achieved using the cross-modality. The resultant cross-modal features play an essential role in generating pseudo-labels and calculating an auxiliary pixel-wise cross-entropy loss to assist the image encoder in learning the distribution of cross-modal features. Such a guiding process allows the extension of the distance between similar classes in feature space so that a proper distance for adjusting the decision boundary is maintained. Our TigDA achieved the highest performance among other UDA methods in both single-resolution and multi-resolution cases with the help of GTA5 and SYNTHIA for the source domain and Cityscapes for the target domain. The simplicity and versatility of TigDA will be widely applicable for enhancing the self-training capabilities of most UDA methods.
更多查看译文
关键词
Unsupervised domain adaptation, Text-image correlation, Self-training
AI 理解论文
溯源树
样例
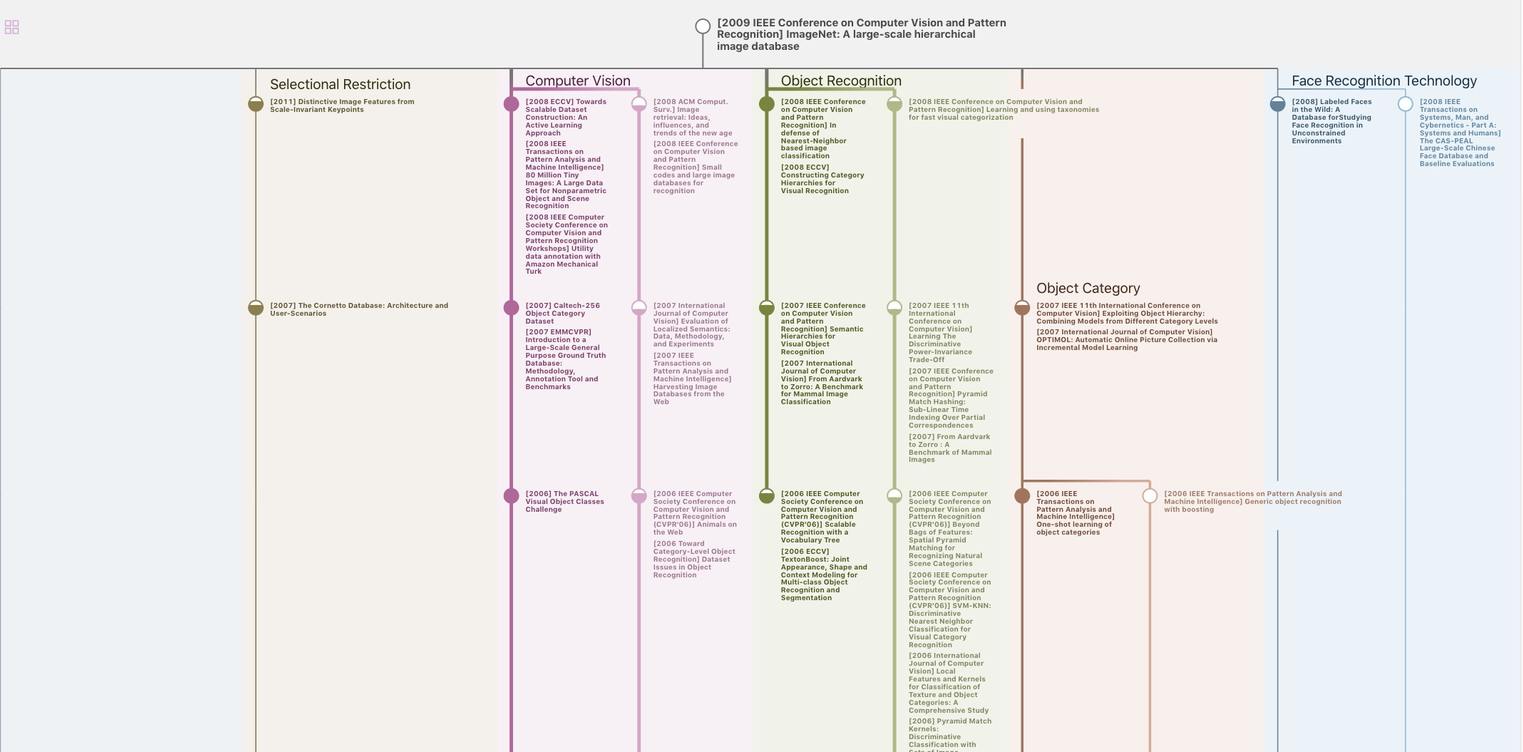
生成溯源树,研究论文发展脉络
Chat Paper
正在生成论文摘要