ML-based Individual Contribution Assessment of Basketball Players from Their Trajectories.
MDM(2023)
摘要
The increasing use of trajectory data and machine learning has advanced our understanding of human behavior. Specifically, in situations such as team sports, in which multiple players form a group and interact with each other, predicting performance using their trajectory data as input has been attracting attention. However, understanding the role and contributions of individuals in the group by a deep understanding of the whole trajectory has not yet been well-investigated. In this study, we propose a method to quantitatively evaluate the single player’s contribution based on a deep learning model that predicts shooting success from the trajectory data of all players and a ball, leveraging official data of a professional basketball league. We use the difference of two output values by the prediction model when the trajectory data of the target player is given or not given as the model inputs. Our evaluation using the professional basketball dataset for one season confirmed that the predictive model had an accuracy of AUC=0.92. We also confirmed that the scoring contribution of each player calculated from this predictive model was significantly correlated with an existing player’s overall performance metric (R = 0.37, p < 0.001). The results suggest that our proposed method could be a new method to quantify a player's contribution to the team performance from trajectory data only instead of conventional experience-based player performance metrics.
更多查看译文
关键词
trajectory,deep learning,CNN,sports,basketball
AI 理解论文
溯源树
样例
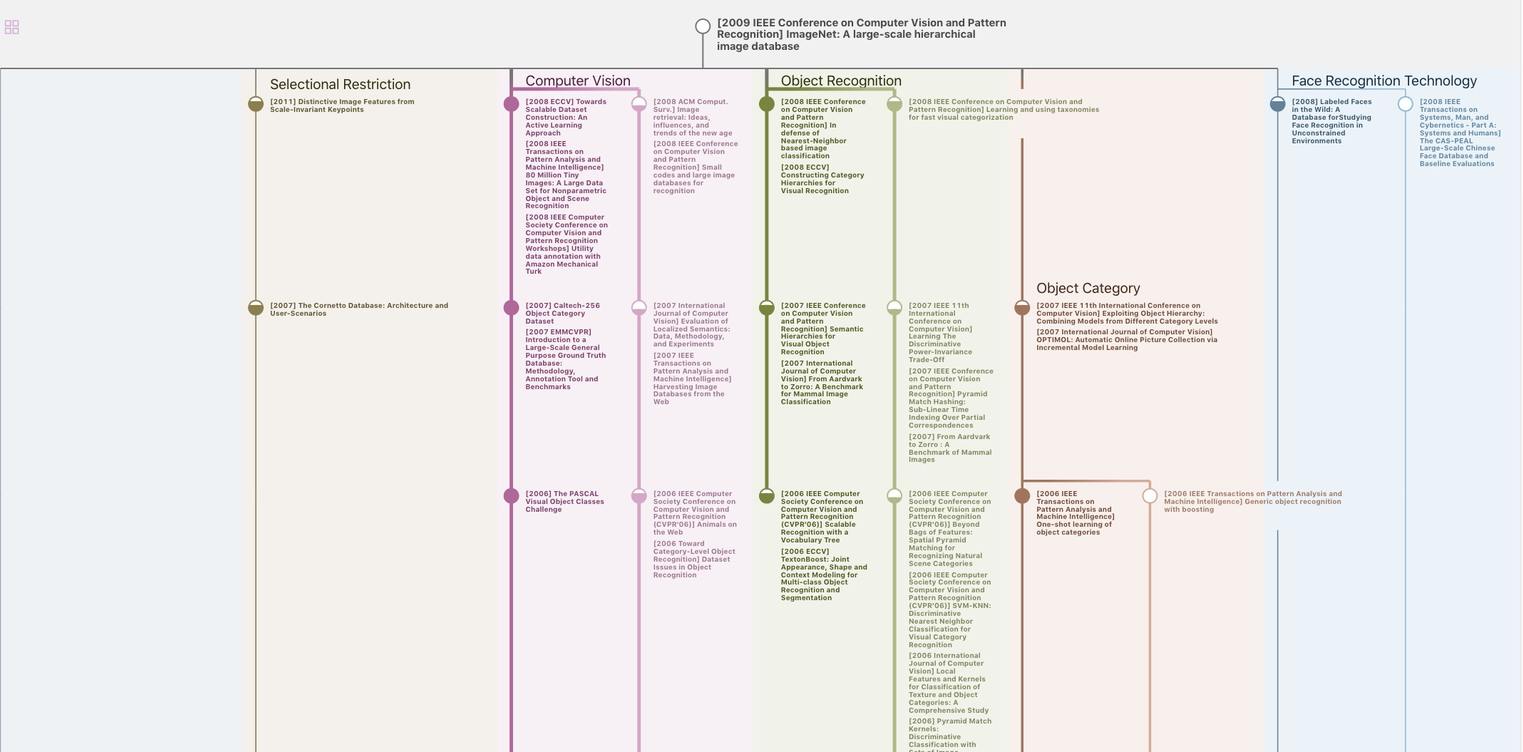
生成溯源树,研究论文发展脉络
Chat Paper
正在生成论文摘要