Learnable Query Guided Representation Learning for Treatment Effect Estimation.
IJCNN(2023)
摘要
The estimation of Individual Treatment Effect (ITE) is a challenging problem in causal inference, due to the missing counterfactual data and the selection bias. In this paper, we propose a novel representation learning framework via the Learnable Query based transformer for Treatment Effect Estimation (LQTEE). A certain number of queries are learned in a sample-agnostic way and extract global critical features from covariate and treatment data separately. We also propose the hierarchical propensity score regularized adversarial loss to obtain balanced covariate representations, and the mutual orthogonal constraint to force queries to focus on diverse parts of covariates, thus the impact of instrumental variables can be adaptively reduced. Treatment representation learning enables our estimator to support general-purpose treatments, and more importantly, it can reveal the underlying patterns of data-generation process efficiently. Extensive experiments show that our ITE estimator significantly outperforms the state-of-the-art methods.
更多查看译文
关键词
treatment effect,representation learning,covariate and treatment,learnable query based transformer
AI 理解论文
溯源树
样例
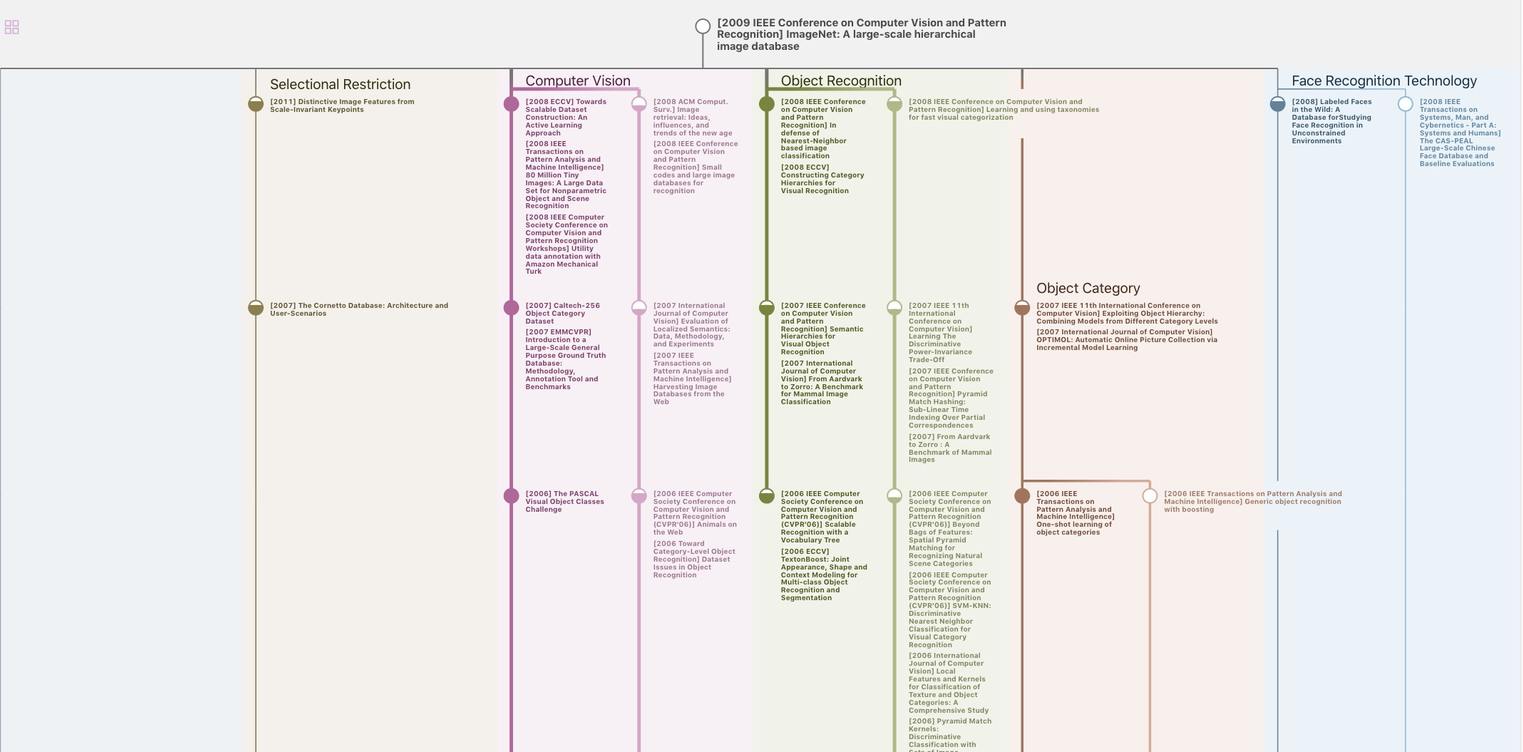
生成溯源树,研究论文发展脉络
Chat Paper
正在生成论文摘要