Automatic Hemiplegia Gait Assessment for Post-Stroke by an Efficient Hybrid Attention-Based GhostNet.
IJCNN(2023)
摘要
Vision-based gait analysis provides the possibility to automatically and unobtrusively detect walking pattern alterations caused by stoke. Therefore, it can be used to determine the severity of stroke during stroke rehabilitation outside the hospital, which greatly releases the economic and labor burden on patients and their families. However, state-of-the-art deep learning algorithms for gait analysis usually suffer from high computational complexity and can even lead to overfitting problems on small-scale pathological gait datasets. To realize an efficient and effective system, we constructed a specially designed dataset and proposed a novel lightweight network to lean discriminative gait representation to map the input into one of the stroke severity levels. More specifically, a simulated hemiplegia gait dataset with multiple severity levels is first constructed, including sufficient 2D image sequences collected from 14 subjects. Different from the existing pathological datasets used for coarse classification, which only distinguish different pathological gait types, our proposed dataset is specifically designed for fine classification to assess the severity of hemiplegia that is defined according to medical prior. Second, considering that pathological datasets are usually small-scale, an attention-based lightweight network is proposed. In detail, a lightweight hybrid attention module (LHAM) based on the 1D adaptive convolution for channel attention interaction was developed to enhance the network's ability to integrate and focus on meaningful spatial and channel features. To further lighten the networks, a proposed efficient ghost module (EGM) is used in the bottleneck structure instead of the normal convolutional layer. Extensive experiments on both self-constructed and publicly available datasets demonstrate that the proposed efficient hybrid attention-based GhostNet realizes an effective and efficient gait analysis for stroke rehabilitation.
更多查看译文
关键词
attention-based lightweight network,automatic hemiplegia gait assessment,channel attention interaction,different pathological gait types,discriminative gait representation,economic labor burden,effective gait analysis,efficient gait analysis,efficient ghost module,efficient hybrid attention-based GhostNet,efficient system,existing pathological datasets,high computational complexity,lightweight hybrid attention module,multiple severity levels,novel lightweight network,pattern alterations,post-stroke,simulated hemiplegia gait dataset,small-scale pathological gait datasets,specially designed dataset,state-of-the-art deep learning algorithms,stroke rehabilitation,stroke severity levels,sufficient 2D image sequences,vision-based gait analysis
AI 理解论文
溯源树
样例
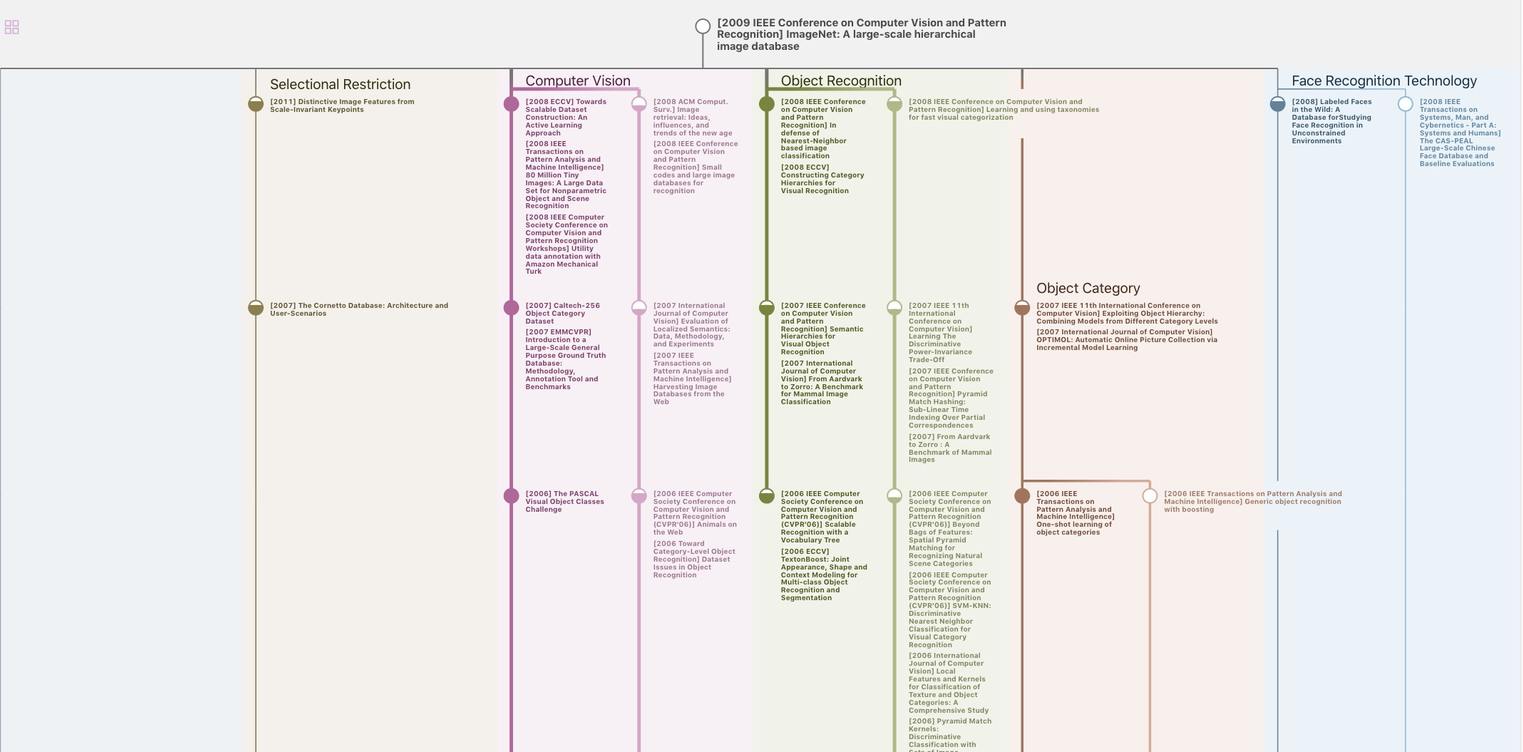
生成溯源树,研究论文发展脉络
Chat Paper
正在生成论文摘要