Multi-channel Attentive Weighting of Visual Frames for Multimodal Video Classification.
IJCNN(2023)
摘要
Multimodal video classification aims to incorporate semantic information to regularize the visual representation learning of videos. Conventional methods typically focus on analyzing all information extracted from different modals rather than key information. However, they usually face the problem of handling the redundant video frames of little categorical information. To address this problem, this paper proposes a novel approach that employs multi-channel weighting of visual frames to mitigate the interference of redundant information. Specifically, the proposed algorithm, termed MCA-WF, includes two main modules, where the multi-channel attentive weighting of video frames (McAW) module performs the multi-granularity and multi-channel frame weighting mechanism based on visual self-attention, contrastive attention and cross-modal attention constraints to filter visual noise and redundant information. The visual frame selection (VFS) module explores the combination of multi-channel attention mechanisms to select the key visual information in the video. Experiments were conducted on MSR-VTT and ActivityNet Captions datasets in terms of performance comparison, ablation study, in-depth analysis, and case studies. The results verified that MCA-WF can notice the key information in the classification and effectively improve the ability of information complementation and integration between modals, which leads to better performance than the state-of-the-art methods.
更多查看译文
关键词
Video classification,Multimodal information,Multi-channel,Key-frame selection,Attention
AI 理解论文
溯源树
样例
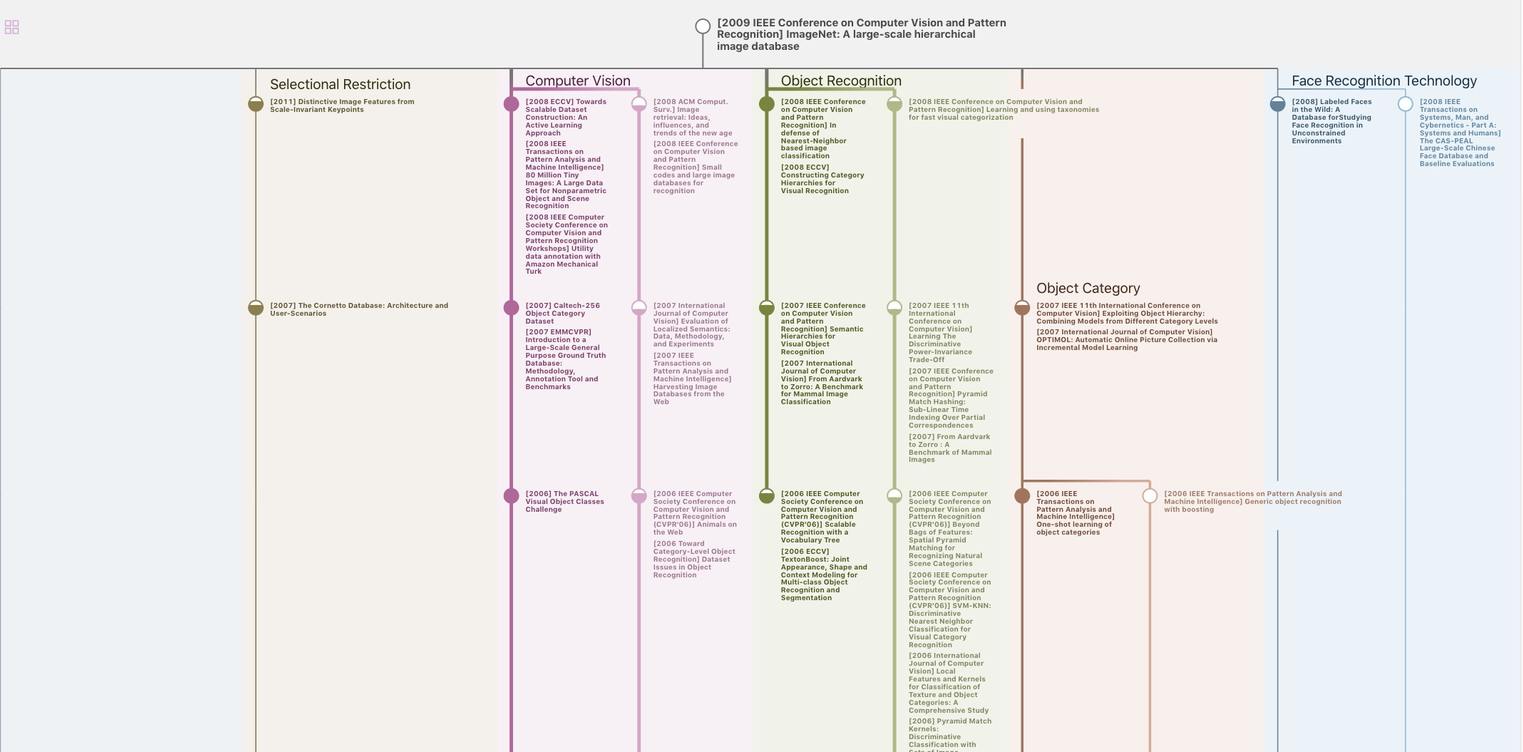
生成溯源树,研究论文发展脉络
Chat Paper
正在生成论文摘要