FOGL: Federated Object Grasping Learning
2023 IEEE INTERNATIONAL CONFERENCE ON ROBOTICS AND AUTOMATION, ICRA(2023)
摘要
Federated learning is a promising technique for training global models in a data-decentralized environment. In this paper, we propose a federated learning approach for robotic object grasping. The main challenge is that the data collected by multiple robots deployed in different environments tends to form heterogeneous data distributions (i.e., non-IID) and that the existing federated learning methods on such data distributions show serious performance degradation. To tackle this problem, we propose federated object grasping learning (FOGL) that uses cross-evaluation in a general federated learning process to assess the training performance of robots. We cluster robots with similar training patterns and perform independent federated learning on each cluster. Finally, we integrate the global models for each cluster through an ensemble inference. We apply FOGL to various federated learning scenarios in robotic object grasping and show state-of-the-art performance on the Cornell grasping dataset.
更多查看译文
AI 理解论文
溯源树
样例
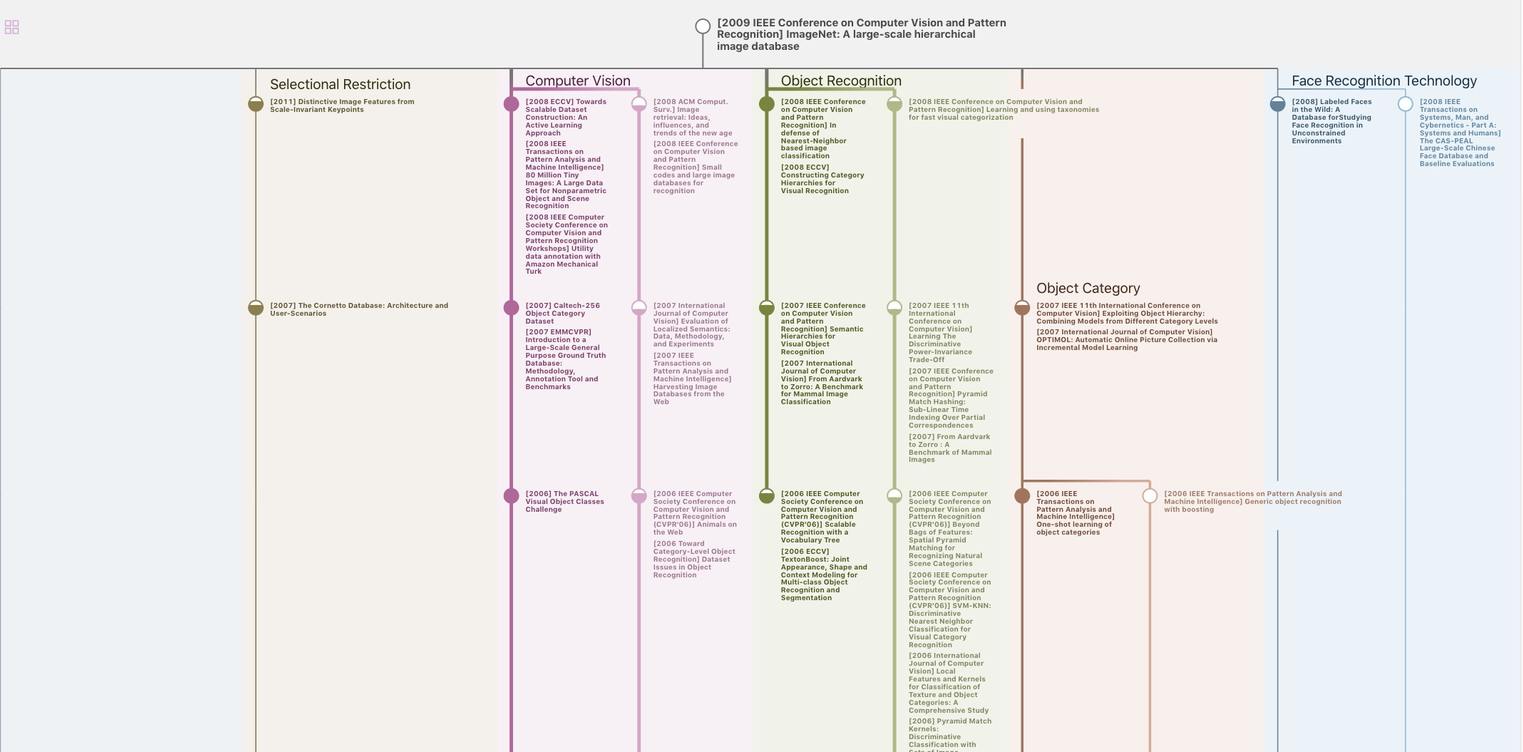
生成溯源树,研究论文发展脉络
Chat Paper
正在生成论文摘要