DDK: A Deep Koopman Approach for Longitudinal and Lateral Control of Autonomous Ground Vehicles
2023 IEEE INTERNATIONAL CONFERENCE ON ROBOTICS AND AUTOMATION, ICRA(2023)
摘要
Autonomous driving has attracted lots of attention in recent years. For some tasks, e.g., trajectory prediction, motion planning, and trajectory tracking, an accurate vehicle model can reduce the difficulty of these tasks and improve task completion performance. Prior works focused on parameter estimation of physical models or modeling nonlinear dynamics using neural networks. Still, these methods rely on internal parameters of vehicles or are not friendly for control due to the strong nonlinearity of models. This paper proposes a data-driven method to approximate vehicle dynamics based on the Koopman operator. The resulting model is an interpretable linear time-invariant model, facilitating controller design and solving related optimization problems. In the proposed approach, the state transition matrix is constructed based on the learned Koopman eigenvalues, while the input matrix is trained as a tensor. Based on the resulting model, a linear model predictive controller is designed to implement coupled longitudinal and lateral trajectory tracking. Simulations and experiments, including vehicle dynamics modeling and coupled longitudinal and lateral trajectory tracking, are performed in a high-fidelity CarSim environment and a real vehicle platform. An oil-driven D-Class SUV is selected in the simulation, while a real electric SUV is utilized in the experiment. Simulation and experiment results illustrate that the model of the nonlinear vehicle dynamics can be identified effectively via the proposed method, and high-quality trajectory tracking performance can be obtained with the resulting model.
更多查看译文
AI 理解论文
溯源树
样例
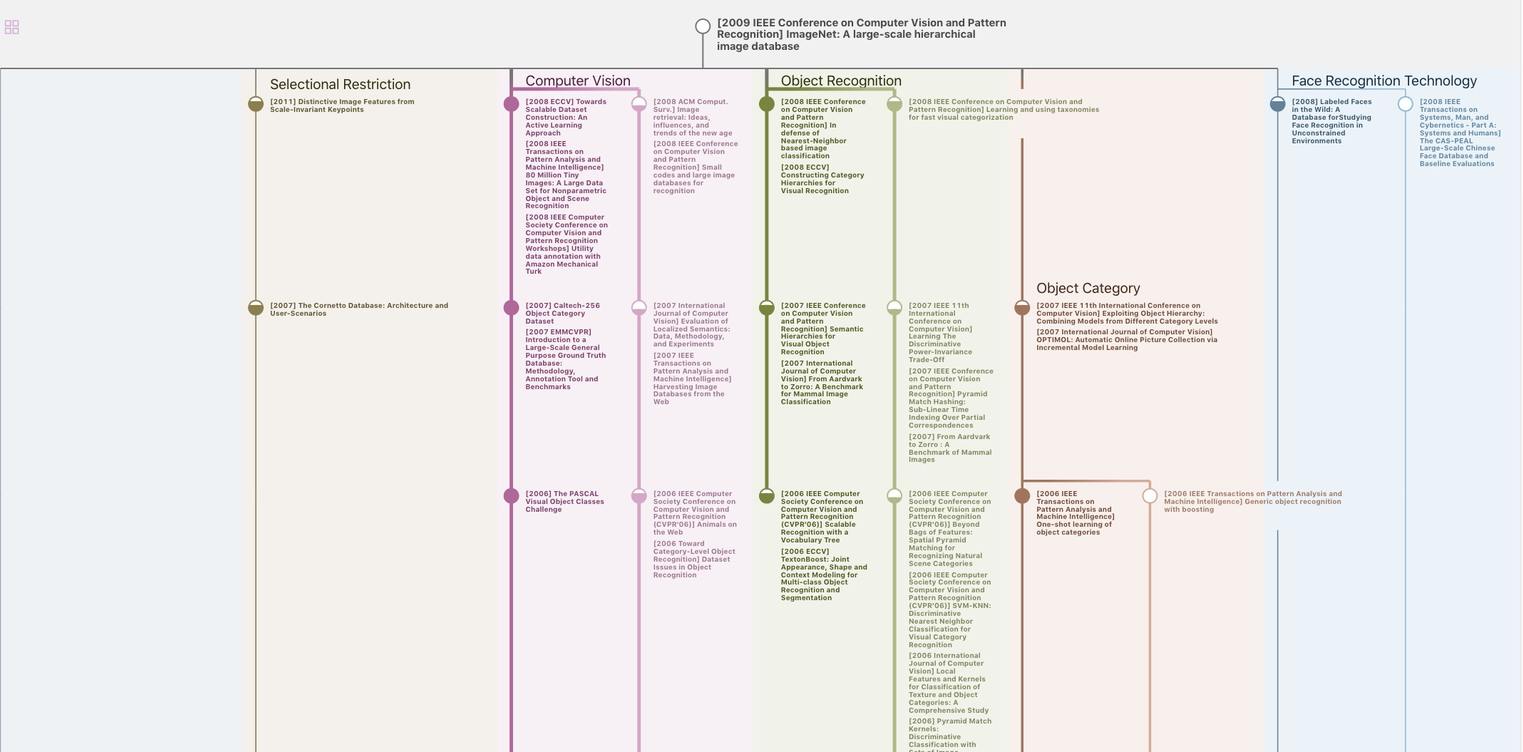
生成溯源树,研究论文发展脉络
Chat Paper
正在生成论文摘要