Guiding Reinforcement Learning with Shared Control Templates.
ICRA(2023)
摘要
Purposeful interaction with objects usually requires certain constraints to be respected, e.g. keeping a bottle upright to avoid spilling. In reinforcement learning, such constraints are typically encoded in the reward function. As a consequence, constraints can only be learned by violating them. This often precludes learning on the physical robot, as it may take many trials to learn the constraints, and the necessity to violate them during the trial-and-error learning may be unsafe. We have serendipitously discovered that constraint representations for shared control - in particular Shared Control Templates (SCTs) - are ideally suited for safely guiding RL. Representing constraints explicitly, rather than implicitly in the reward function, also simplifies the design of the reward function. The main advantage of the approach is safer, faster learning without constraint violations (even with sparse reward functions). We demonstrate this in a pouring task in simulation and on a real robot, where learning the task requires only 65 episodes in 16 minutes.
更多查看译文
关键词
constraint representations,constraint violations,physical robot,reinforcement learning,RL,SCT,shared control templates,sparse reward functions,trial-and-error learning
AI 理解论文
溯源树
样例
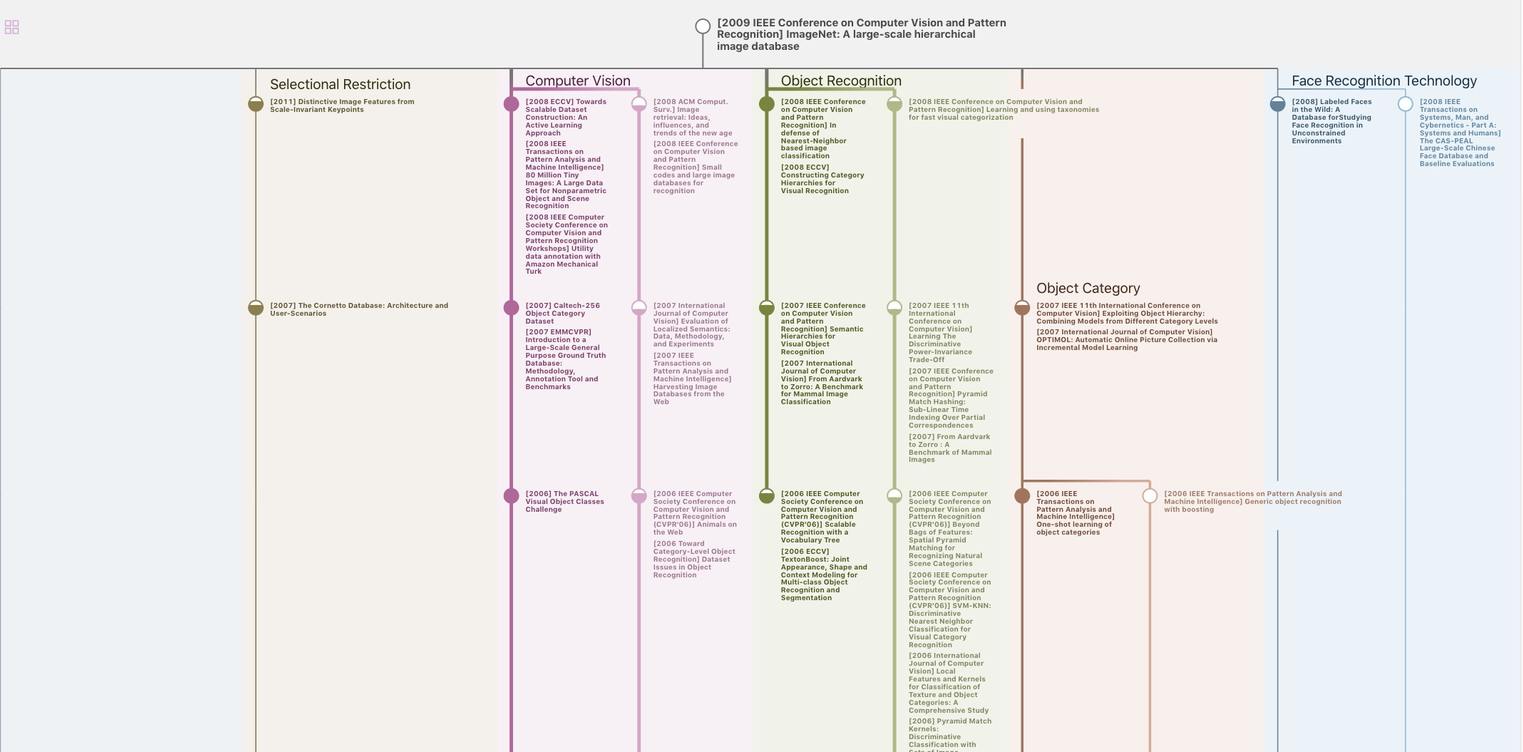
生成溯源树,研究论文发展脉络
Chat Paper
正在生成论文摘要