Exploring Federated Learning for Speech-based Parkinson's Disease Detection
18TH INTERNATIONAL CONFERENCE ON AVAILABILITY, RELIABILITY & SECURITY, ARES 2023(2023)
摘要
Parkinson's Disease is the second most prevalent neurodegenera-tive disorder, currently affecting as high as 3% of the global popula-tion. Research suggests that up to 80% of patients manifest phona-tory symptoms as early signs of the disease. In this respect, various systems have been developed that identify high risk patients by analyzing their speech using recordings obtained from natural dia-logues and reading tasks conducted in clinical settings. However, most of them are centralized models, where training and inference take place on a single machine, raising concerns about data privacy and scalability. To address these issues, the current study migrates an existing, state- of-the-art centralized approach to the concept of federated learning, where the model is trained in multiple inde-pendent sessions on different machines, each with its own dataset. Therefore, the main objective is to establish a proof of concept for federated learning in this domain, demonstrating its effectiveness and viability. Moreover, the study aims to overcome challenges asso-ciated with centralized machine learning models while promoting collaborative and privacy-preserving model training.
更多查看译文
关键词
Federating Learning,Parkinson's Disease,Speech Articulation
AI 理解论文
溯源树
样例
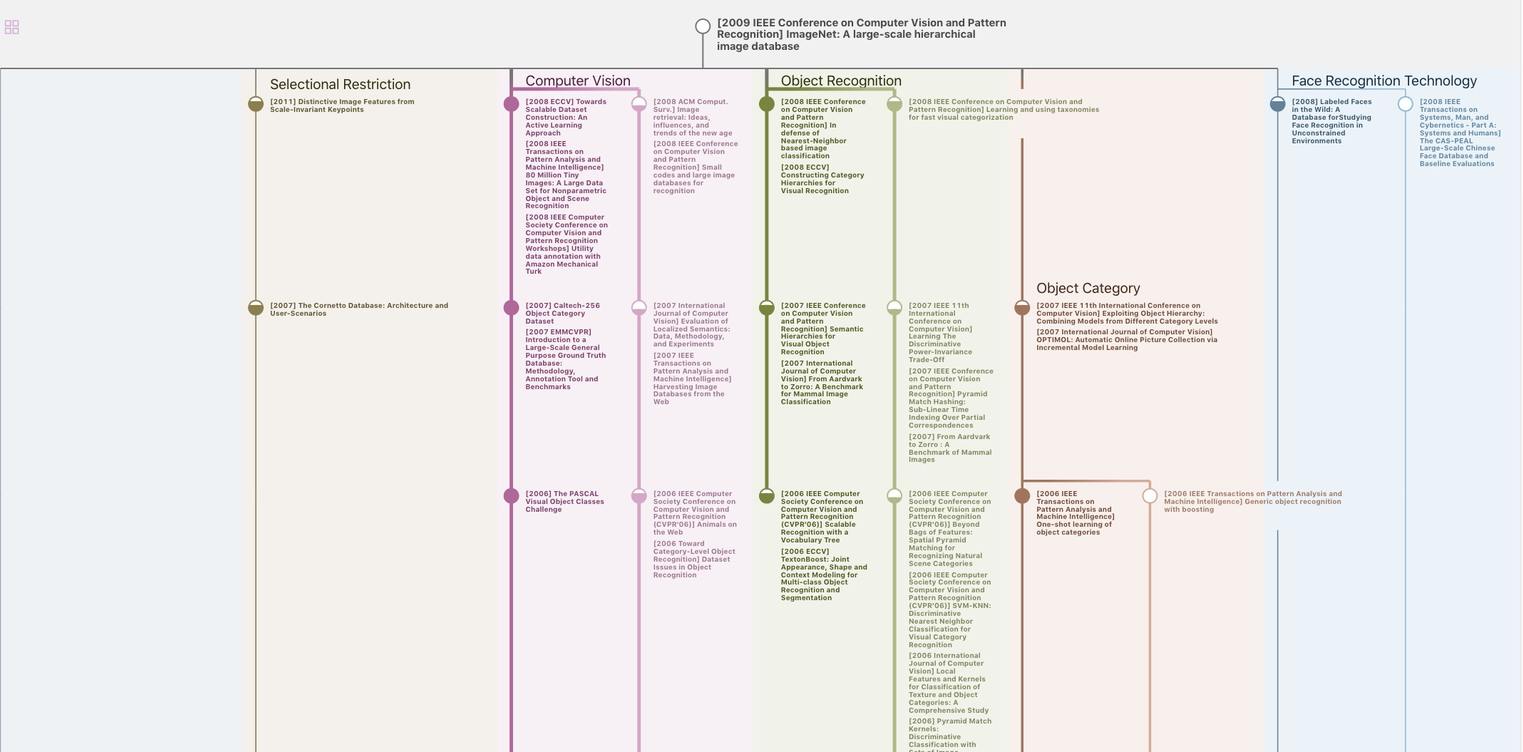
生成溯源树,研究论文发展脉络
Chat Paper
正在生成论文摘要