Deep Unsupervised Visual Odometry Via Bundle Adjusted Pose Graph Optimization
2023 IEEE INTERNATIONAL CONFERENCE ON ROBOTICS AND AUTOMATION, ICRA(2023)
摘要
Unsupervised visual odometry as an active topic has attracted extensive attention, benefiting from its label-free practical value and robustness in real-world scenarios. However, the performance of camera pose estimation and tracking through deep neural network is still not as ideal as most other tasks, such as detection, segmentation and depth estimation, due to the lack of drift correction in the estimated trajectory and map optimization in the recovered 3D scenes. In this work, we introduce pose graph and bundle adjustment optimization to our network training process, which iteratively updates both the motion and depth estimations from the deep learning network, and enforces the refined outputs to further meet the unsupervised photometric and geometric constraints. The integration of pose graph and bundle adjustment is easy to implement and significantly enhances the training effectiveness. Experiments on KITTI dataset demonstrate that the introduced method achieves a significant improvement in motion estimation compared with other recent unsupervised monocular visual odometry algorithms.
更多查看译文
AI 理解论文
溯源树
样例
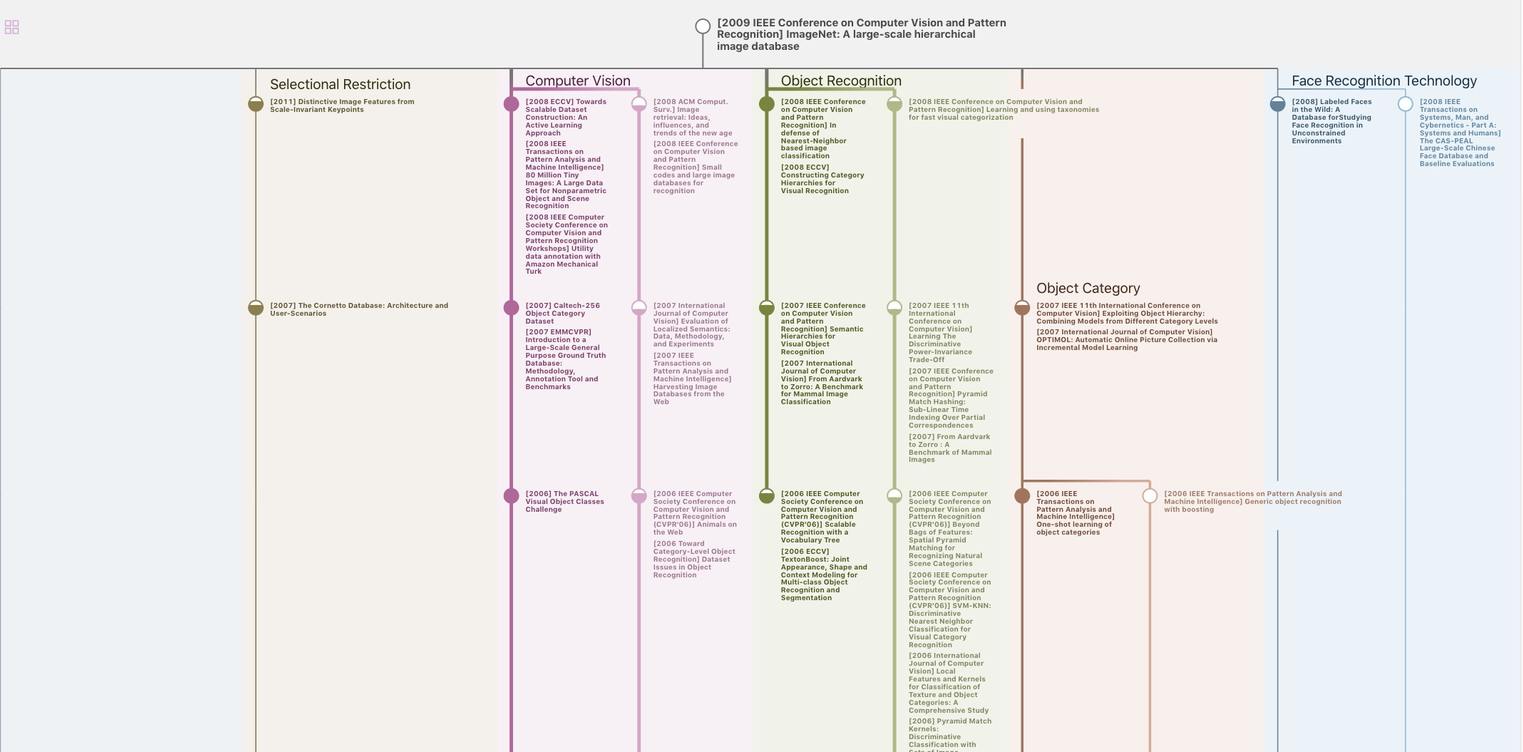
生成溯源树,研究论文发展脉络
Chat Paper
正在生成论文摘要