Design Optimization and Data-driven Shallow Learning for Dynamic Modeling of a Smart Segmented Electroadhesive Clutch.
ICRA(2023)
摘要
Electroadhesive clutches have attracted a great deal of interest in the last decade as semi-active actuators for human-robot interaction due to their lightweight, low power consumption, and tunable high-torque output capability. However, because of the complexity of their dynamics, in most cases, they are utilized in an ON/OFF-control strategy. In this regard, the non-autonomous (time-dependent) degradation of electroadhesive behavior is an inherent challenge that injects unpredictability and uncertainty into the behavior of this family of semi-active clutches. We propose a novel approach to preventing degradation of electroadhesion using a segmented electrode design that modulates the electrical field on the dielectric surface while using a direct current signal and securing low power consumption. This paper, for the first time, presents an optimization process based on a novel analytic model of the proposed actuator. It also develops a data-driven model augmentation using a hybrid shallow learning approach composed of a long short-term memory (LSTM) architecture which is combined with the analytical model. The performance of the proposed semi-active clutch and the data-driven hybrid model is experimentally validated in this paper.
更多查看译文
关键词
analytical model,data-driven hybrid model,data-driven model augmentation,data-driven shallow learning,design optimization,dielectric surface,direct current signal,dynamic modeling,human-robot interaction,hybrid shallow learning approach,long short-term memory architecture,LSTM,nonautonomous degradation,ON-OFF -control strategy,power consumption,segmented electrode design,semiactive actuators,semiactive clutch,smart segmented electroadhesive clutch,tunable high-torque output capability
AI 理解论文
溯源树
样例
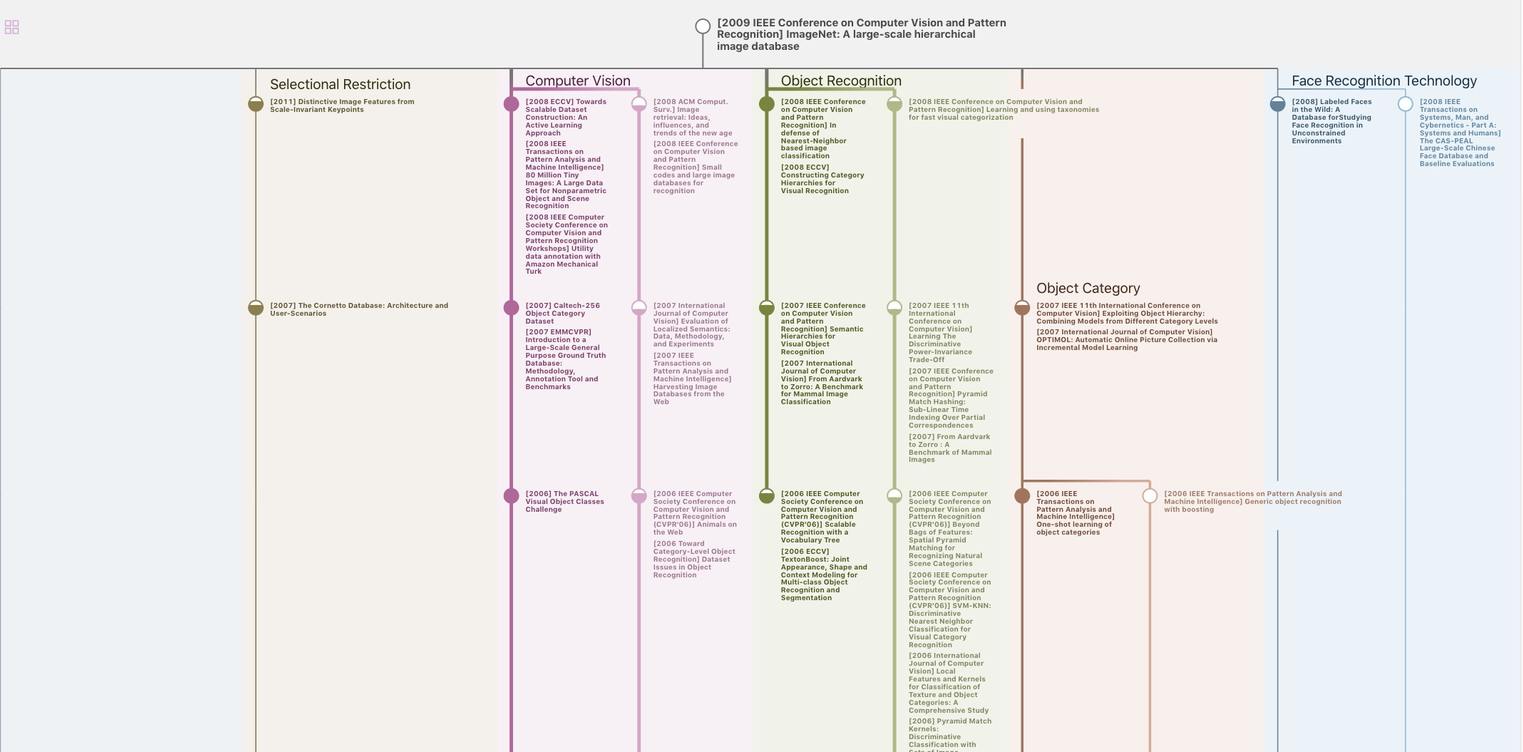
生成溯源树,研究论文发展脉络
Chat Paper
正在生成论文摘要