Loosely-coupled localization fusion system based on track-to-track fusion with bias alignment
2023 IEEE INTERNATIONAL CONFERENCE ON ROBOTICS AND AUTOMATION, ICRA(2023)
摘要
The localization system is an essential element in robotics, which can provide accurate position information. Multiple localization systems can be integrated for reliable localization operations because there are various methods for measuring the position or processing algorithms. Significantly, the track-to-track (T2T) fusion method can fuse multiple localization systems using each system's estimate without accessing the sensor's low data. However, most T2T fusion-based localization systems ignore slowly varying biases, such as drift errors, odometry errors, and offsets among multiple maps. This can degrade the localization performance because a slowly varying bias is directly reflected in the localization estimate. Therefore, a slowly varying bias must be considered in the fusion process to derive reliable estimates. This study proposes a T2T fusion-based localization system that considers a slowly varying bias. First, the slow-varying bias difference between the systems was estimated. Because each localization system can have a different bias, the estimated bias difference was used to align it with the reference system. Second, a fused estimate can be obtained by T2T fusion using bias-aligned estimates. The proposed fusion system can also be used without limiting the number of inputs to the localization system. The proposed system was compared with various T2T-based localization fusion algorithms for verification in a simulation environment, and it exhibited the best performance in RMSE error comparison.
更多查看译文
关键词
bias alignment,different bias,estimated bias difference,fusion algorithms,fusion process,localization estimate,localization fusion system,localization performance,multiple localization systems,reference system,reliable localization operations,slowly varying bias,T2T fusion-based localization system,track-to-track fusion method
AI 理解论文
溯源树
样例
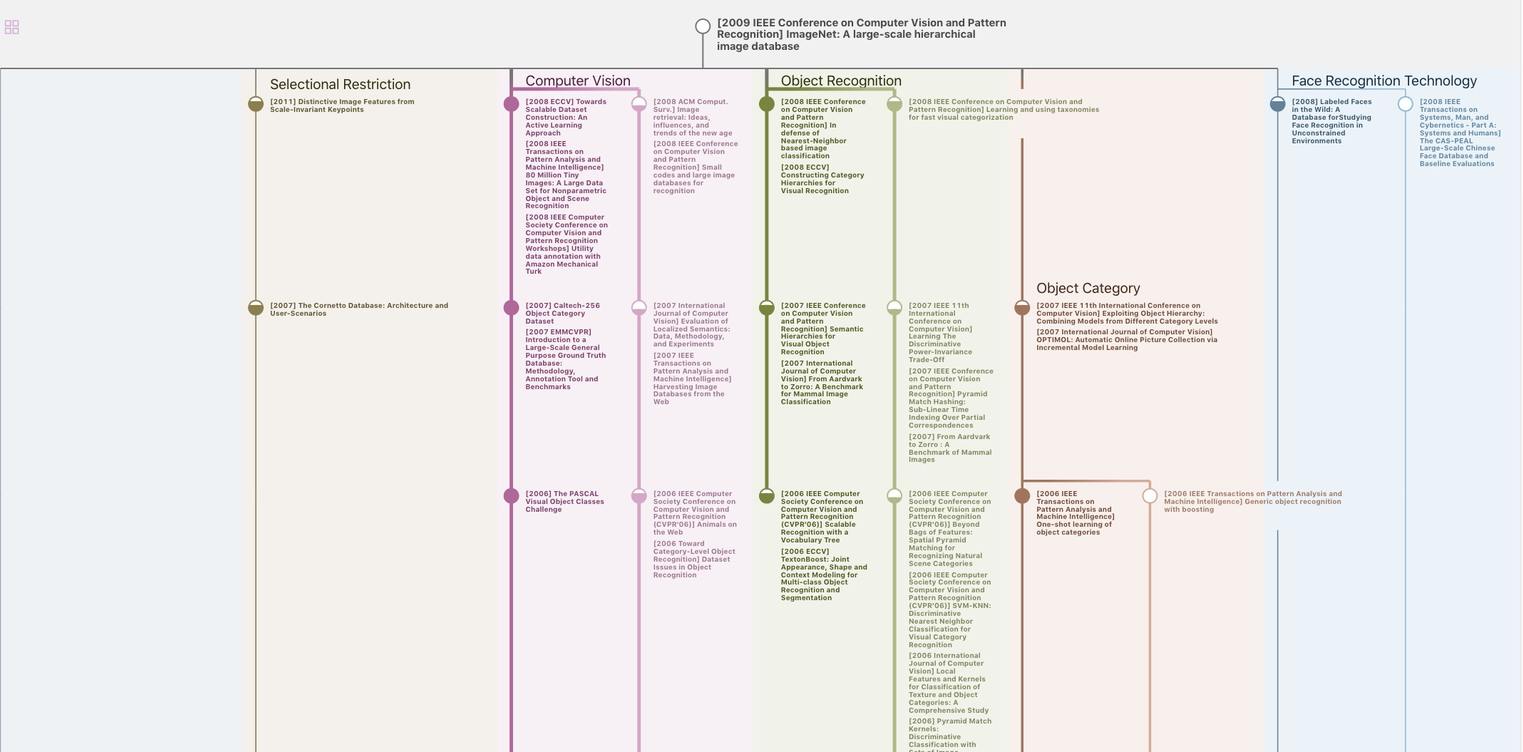
生成溯源树,研究论文发展脉络
Chat Paper
正在生成论文摘要