Start State Selection for Control Policy Learning from Optimal Trajectories
2023 IEEE INTERNATIONAL CONFERENCE ON ROBOTICS AND AUTOMATION, ICRA(2023)
摘要
Combination of optimal control methods and machine learning approaches allows to profit from complementary benefits of each field in control of robotic systems. Data from optimal trajectories provides valuable information that can be used to learn a near-optimal state-dependent feedback control policy. To obtain high-quality learning data, careful selection of optimal trajectories, determined by a set of start states, is essential to achieve a good learning performance. In this paper, we extend previous work with new complementing strategies to generate start points. These methods complement the existing approach, as they introduce new criteria to identify relevant regions in joint state space that need coverage by new trajectories. It is demonstrated that the extensions significantly improve the overall performance of the previous method in simulation on full nonlinear dynamics model of the industrial Manutec r3 robot arm. Further, it is demonstrated that it suffices to learn a policy that reaches the proximity of the goal state, from where a PI controller can be used for stable control reaching the final system state.
更多查看译文
AI 理解论文
溯源树
样例
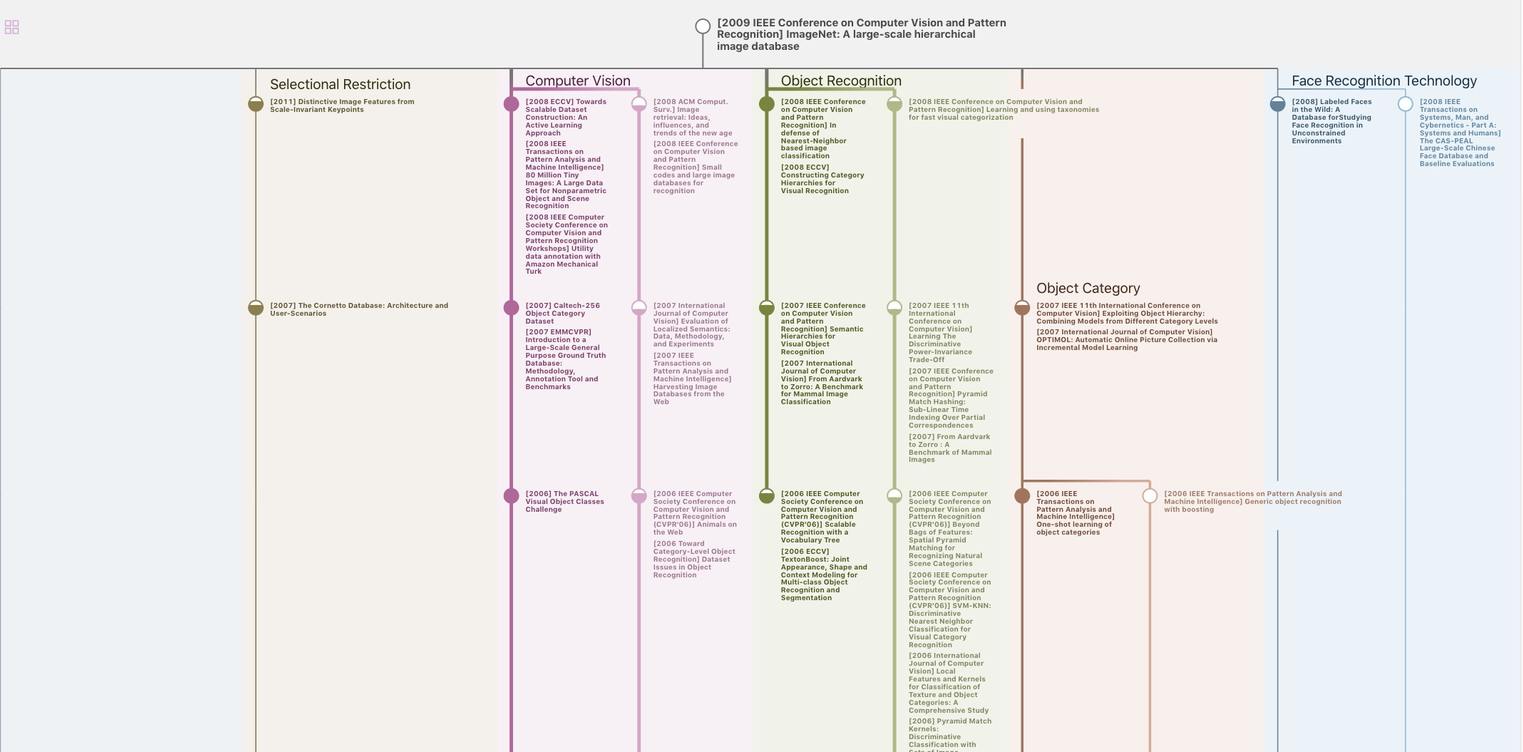
生成溯源树,研究论文发展脉络
Chat Paper
正在生成论文摘要