Dual quaternion based dynamic movement primitives to learn industrial tasks using teleoperation
2023 IEEE INTERNATIONAL CONFERENCE ON ROBOTICS AND AUTOMATION, ICRA(2023)
摘要
Dynamic movement primitives (DMPs) provide an effective method of learning manipulation skills from human demonstration. DMPs can be especially useful for imitating industrial manipulation tasks which are performed by humans and are difficult to model, for instance, deformable object manipulation. In this work the effectiveness of a conventional Cartesian space DMP is enhanced using a compact and efficient representation of dual quaternions (DQ). We demonstrate that our DQ based DMP learning approach that utilizes the geometrical meaning of screw-based kinematics, outperforms traditional decoupled task-space DMPs in terms of accuracy during learning in certain situations. Our DMP formulation affords two additional applications: (1) Filter the noisy and irregular sensing of human demonstration; (2) Limit the robotic manipulator's task-space velocity during teleoperation, thus improving the safety of the robot and the environment. The learning and filtering strategies are validated on a bimanual robotic system and a motion capture system. We demonstrate the effectiveness of DMP based manipulation of deformable object by learning a bimanual deformation trajectory and then using it to perform the same task in new scenarios.
更多查看译文
AI 理解论文
溯源树
样例
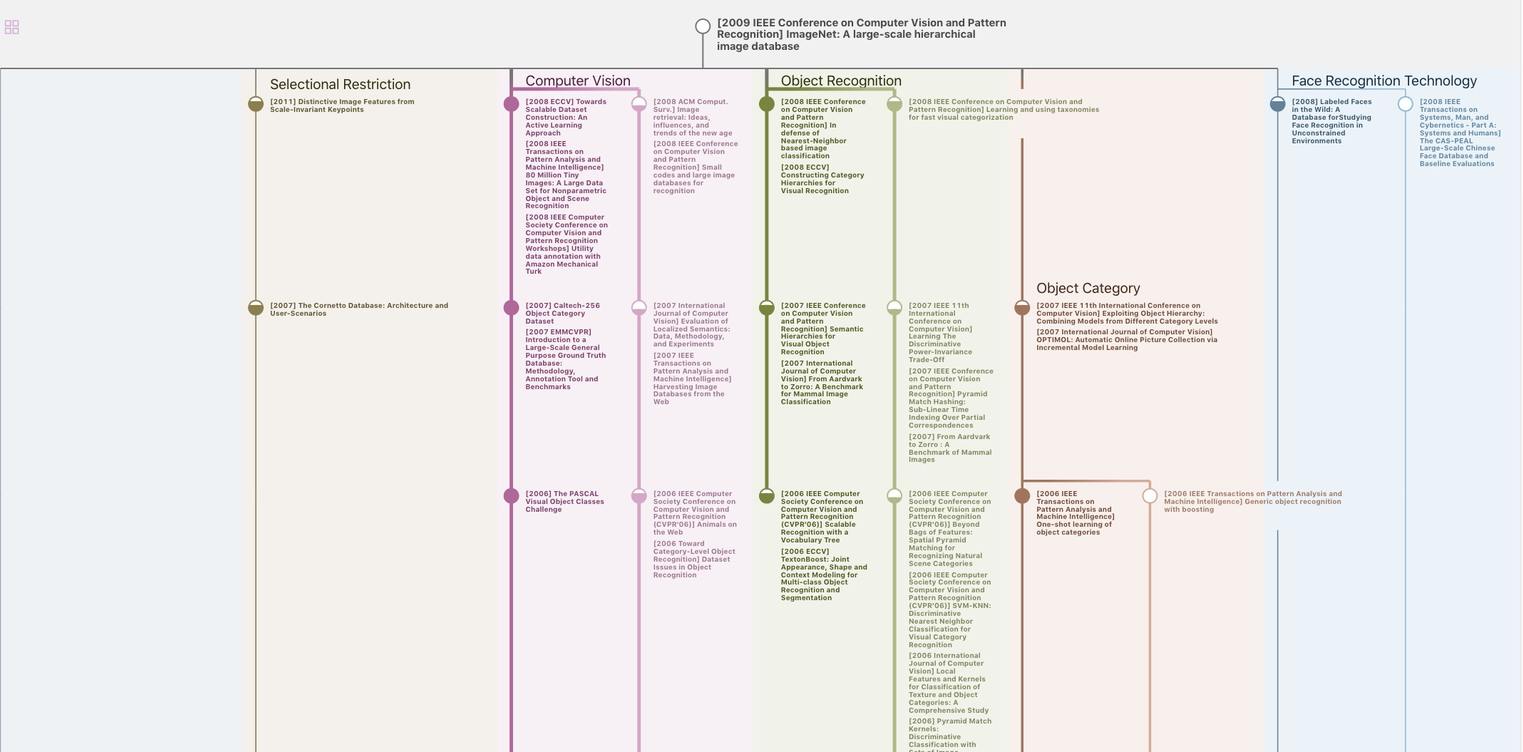
生成溯源树,研究论文发展脉络
Chat Paper
正在生成论文摘要