Universal Compression of High Dimensional Gaussian Vectors with James-Stein shrinkage.
ISIT(2023)
摘要
We study universal compression of n i.i.d. copies of a k−variate Gaussian random vector, when the mean is an unknown vector in an Euclidean ball of ℝ
k
, and the covariance is known. We adopt the high dimensional scaling k = Θ(n) to bring out a compression perspective on the inadmissibility of unbiased estimates of a k−variate Gaussian (when k ≥ 3), in particular focusing on the optimal unbiased Maximum Likelihood estimate. We use arguments based on the redundancy-capacity theorem to show that the redundancy of a universal compressor in this high dimensional setting must be lower bounded as Θ(n). We show that natural compression schemes based on the Maximum Likelihood estimate of the mean have suboptimal Θ(n log n) redundancy, but a scheme based on the James-Stein biased estimate of the mean incurs redundancy that is also Θ(n).
更多查看译文
关键词
Euclidean ball,high dimensional Gaussian vectors,high dimensional scaling k,high dimensional setting,James-Stein biased estimate,James-Stein shrinkage,k-variate Gaussian random vector,natural compression schemes,optimal unbiased maximum likelihood estimate,redundancy-capacity theorem,unbiased estimates,universal compression,universal compressor,unknown vector
AI 理解论文
溯源树
样例
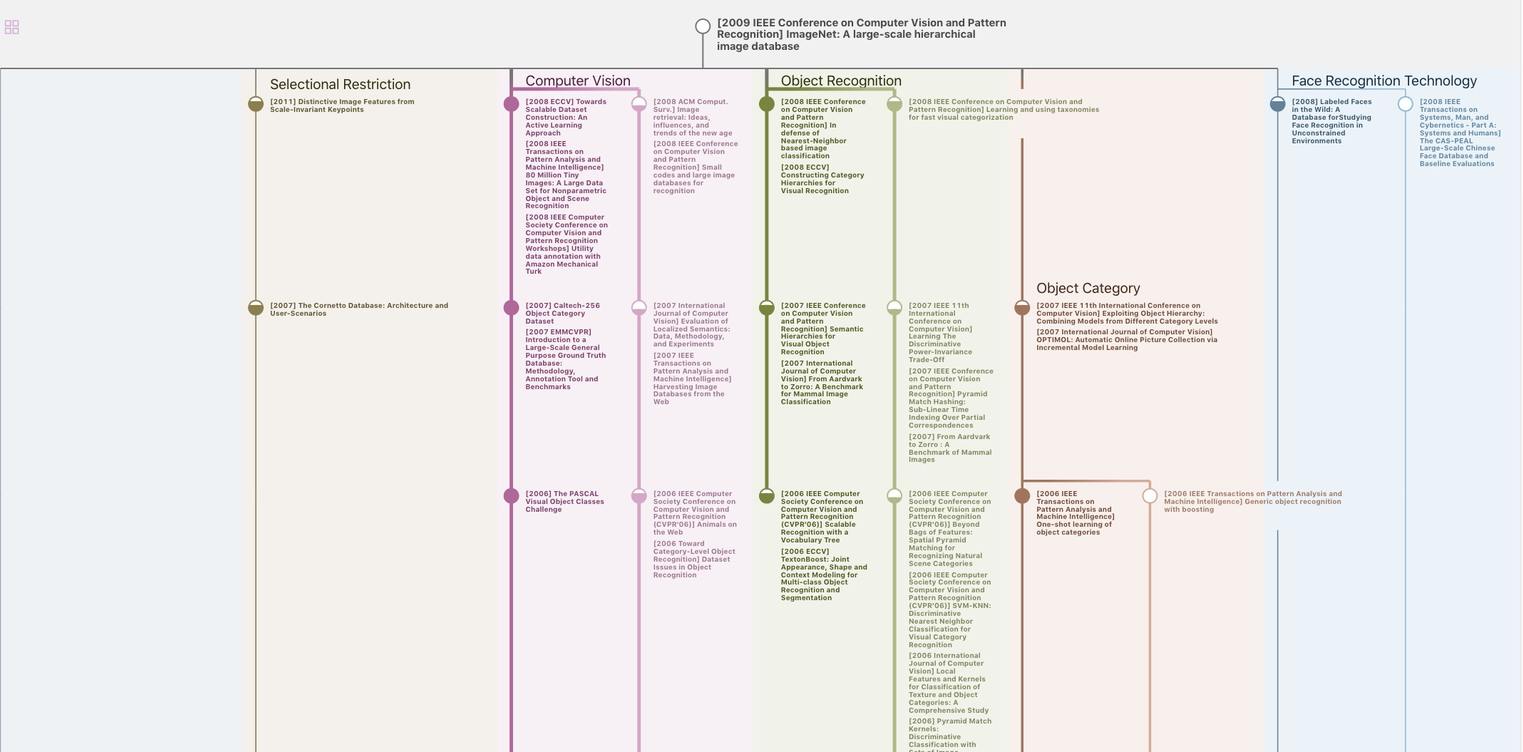
生成溯源树,研究论文发展脉络
Chat Paper
正在生成论文摘要