Rate Distortion via Constrained Estimated Mutual Information Minimization.
ISIT(2023)
摘要
This paper proposes a novel methodology for the estimation of the rate distortion function (RDF) in both continuous and discrete reconstruction spaces. The approach is input-space agnostic and does not require prior knowledge of the source distribution, nor the distortion function, i.e., it treats them as "black box" models. Thus, our method is a general solution to the RDF estimation problem. The approach leverages neural estimation and optimization of information measures to optimize a generative model of the input distribution. In continuous spaces we learn a sample generating model and a PMF model is proposed for discrete spaces. Formal guarantees of the proposed method are explored and implementation details are discussed. We demonstrate the performance on both high dimensional and large alphabet synthetic data. This work has the potential to contribute to the fields of data compression and machine learning through the development of provably consistent and competitive compressors optimized for the fundamental limit of the RDF.
更多查看译文
关键词
alphabet synthetic data,black box models,continuous reconstruction space,data compression,discrete reconstruction space,generative model optimization,information measures,input distribution,machine learning,mutual information minimization,neural estimation,PMF,rate distortion function,RDF estimation,source distribution
AI 理解论文
溯源树
样例
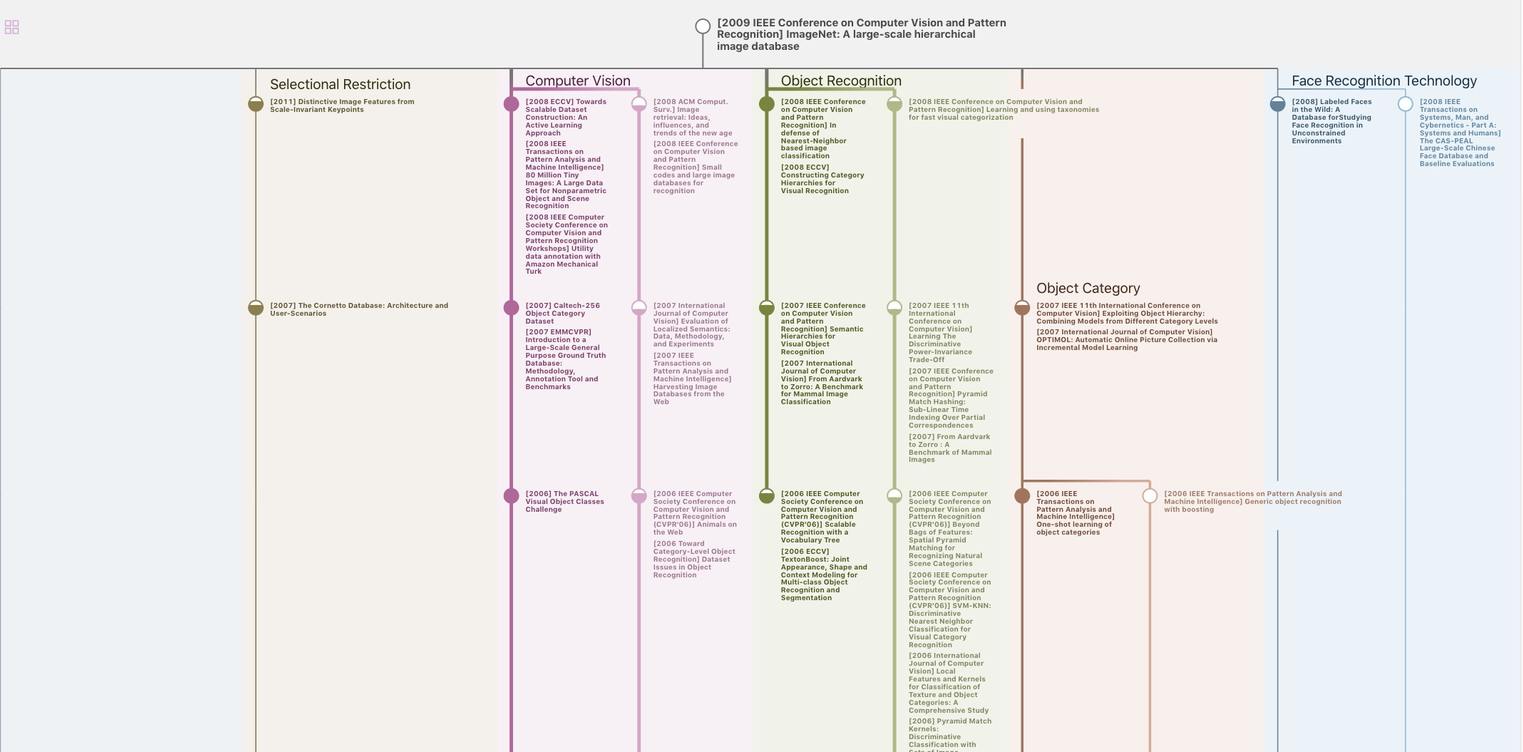
生成溯源树,研究论文发展脉络
Chat Paper
正在生成论文摘要