Principled OOD Detection via Multiple Testing.
ISIT(2023)
摘要
We study the problem of Out-of-Distribution (OOD) detection, that is, detecting whether a Machine Learning (ML) model's output can be trusted at inference time. While a number of tests for OOD detection have been proposed in prior work, a formal framework for studying this problem is lacking. We propose a definition for the notion of OOD that includes both the input distribution and the ML model, which provides insights for the construction of powerful tests for OOD detection. We also propose a multiple hypothesis testing inspired procedure to systematically combine any number of different statistics from the ML model using conformal p-values. We further provide strong guarantees on the probability of incorrectly classifying an in-distribution sample as OOD. In our experiments, we find that threshold-based tests proposed in prior work perform well in specific settings, but not uniformly well across different OOD instances. In contrast, our proposed method that combines multiple statistics performs uniformly well across different datasets and neural networks.
更多查看译文
关键词
conformal p-values,datasets,in-distribution sample,input distribution,machine learning,ML model,multiple hypothesis testing,multiple statistics performs,neural networks,OOD instances,out-of-distribution detection,principled OOD detection,threshold-based tests
AI 理解论文
溯源树
样例
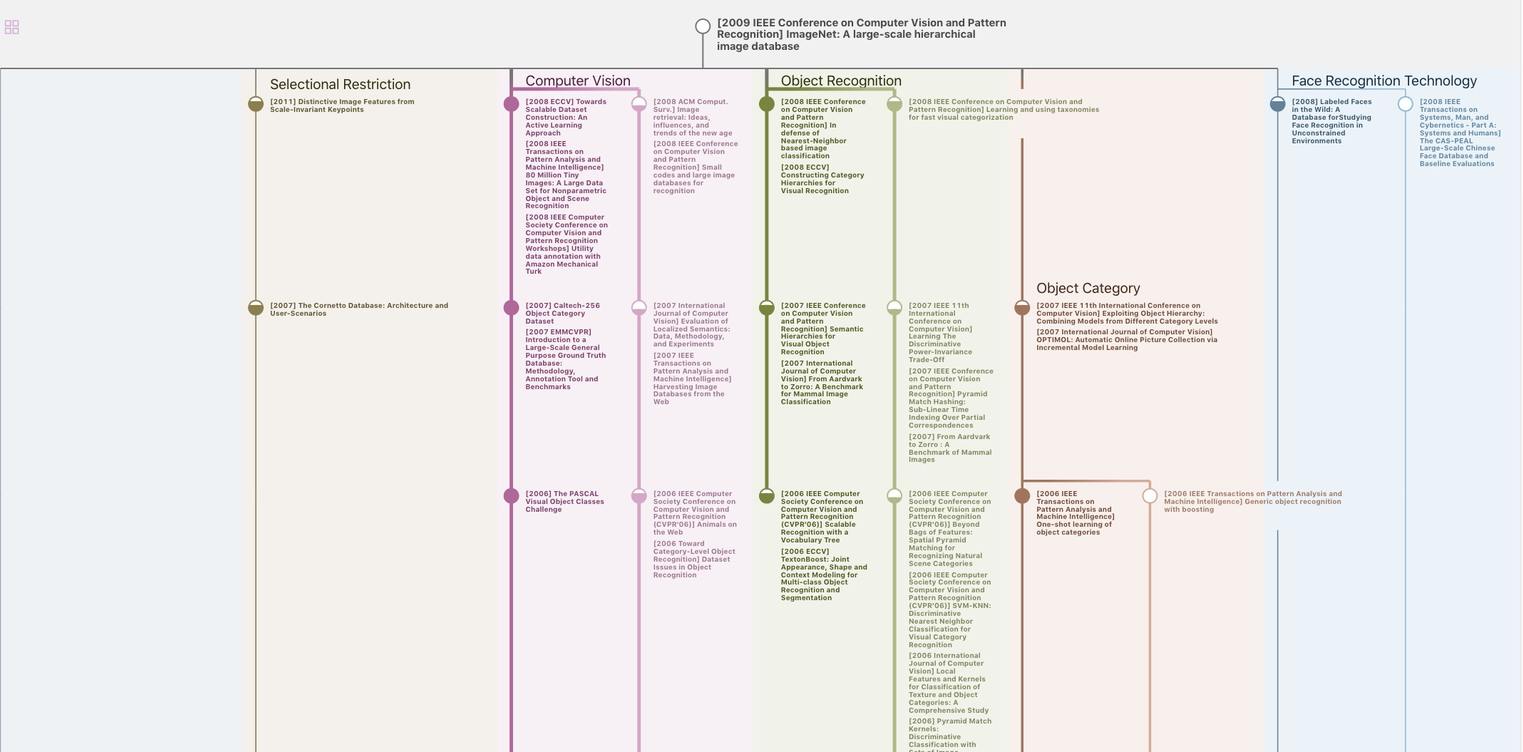
生成溯源树,研究论文发展脉络
Chat Paper
正在生成论文摘要