Arbitrariness of Outward Closeness in Laplacian Dimensionality Reduction.
EUSFLAT/AGOP(2023)
摘要
In this paper, we recall the dimensionality reduction technique of Laplacian eigenmaps. Its result (lower-dimensional embedding, e.g. a single real vector) depends on the initial setting of weights describing closeness on the set of all data points. Then, a weighted graph naturally emerges. We propose to split these weights in two parts: the outward closeness describes the closeness of any point to all the other points, whereas the inward closeness is given by the evaluation of all self-loops. In this contribution, we are interested in the inverse problem to the dimensionality reduction which consists in finding the weights leading to a given result. We show that under certain conditions, the outward closeness is almost arbitrary, i.e. only the inward closeness must be computed to fit the result of the Laplacian eigenmaps to the given vector.
更多查看译文
关键词
outward closeness
AI 理解论文
溯源树
样例
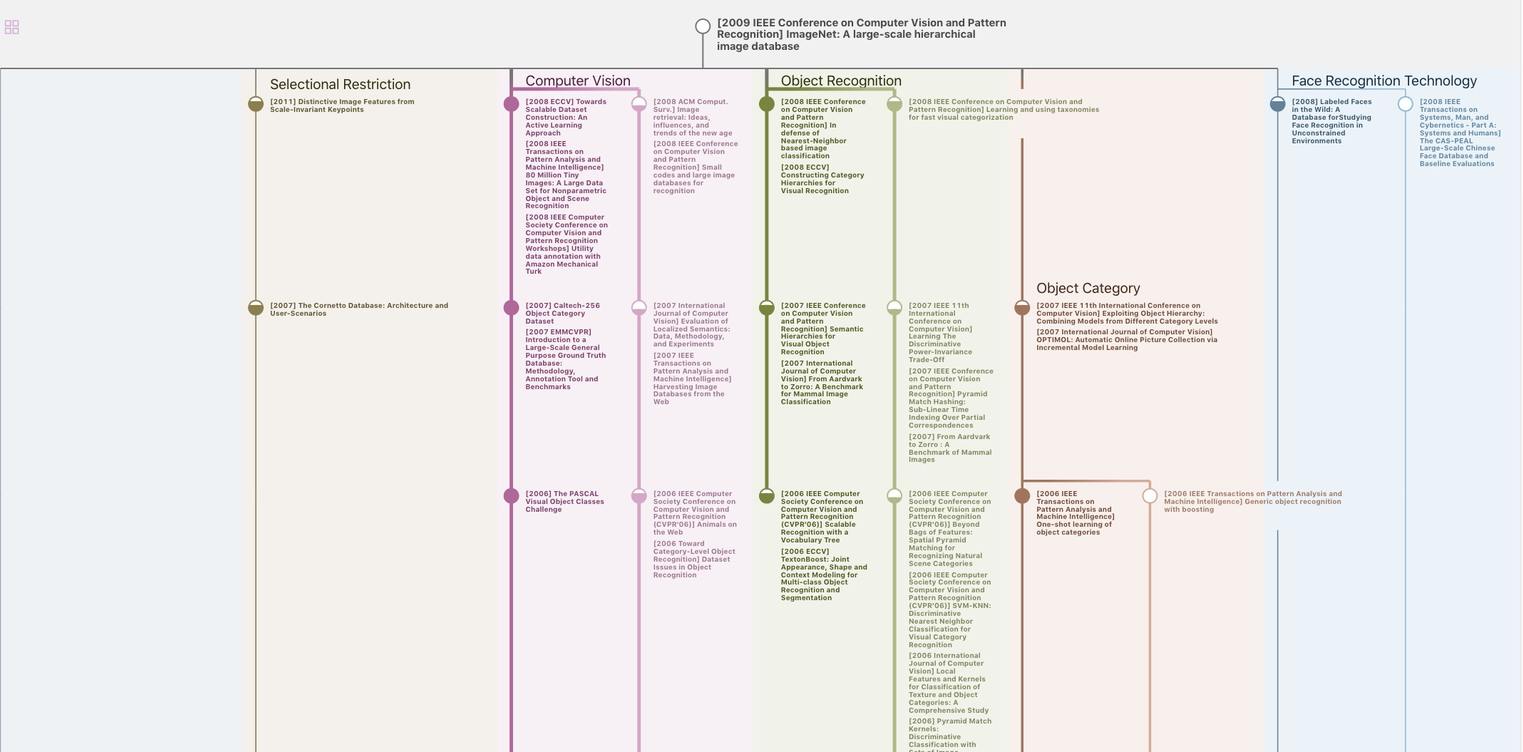
生成溯源树,研究论文发展脉络
Chat Paper
正在生成论文摘要