Multi-level Contrastive Learning for Commonsense Question Answering.
KSEM (4)(2023)
摘要
Recent studies have shown that the integration of external knowledge greatly improves the performance of commonsense question answering. However, the problems of semantic representation discrepancy between questions and external knowledge as well as weak discrimination between choices have not been well ameliorated. To address the above problems, we propose Multi-Level Contrastive Learning named MLCL for commonsense question answering, which includes instance-level and class-level contrastive learning modules. The instance-level contrastive module aims to align questions with knowledge of correct choice in semantic space, and class-level contrastive module focuses on how to make it easier to distinguish between correct and wrong choices. The model achieves state-of-the-art result in CommonsenseQA dataset and outperforms competitive approaches in OpenBookQA. In addition, adequate experiments verify the effectiveness of contrastive learning in multi-choice commonsense question answering.
更多查看译文
关键词
learning,multi-level
AI 理解论文
溯源树
样例
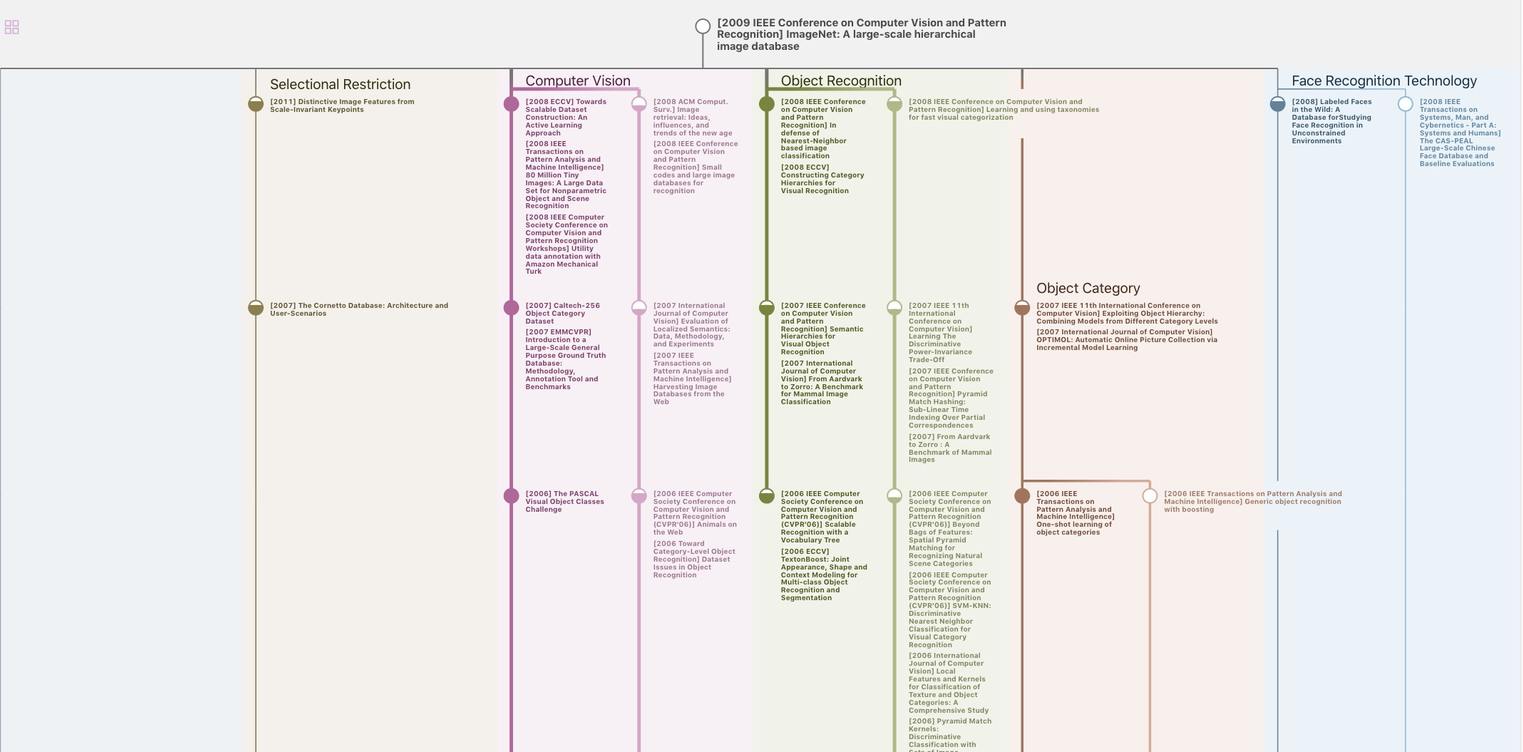
生成溯源树,研究论文发展脉络
Chat Paper
正在生成论文摘要