Dynamic Feed-Forward LSTM.
KSEM (1)(2023)
摘要
We address the insufficient hidden states capabilities and single-direction feeding flaws of existing LSTM caused by its horizontal recurrent steps. To this end, we propose the Dynamic Feed-Forward LSTM (D-LSTM). Specifically, our D-LSTM first expands the capabilities of hidden states by assigning an exclusive state vector to each word. Then, the Dynamic Additive Attention (DAA) method is utilized to adaptively compress local context words into a fixed size vector. Last, a vertical feed-forward process is proposed to search context relations by filtering informative features in the compressed context vector and updating hidden states. With the help of exclusive hidden states, each word can preserve its most correlated context features and hidden states do not interfere with each other. By setting an appropriate context window size for DAA and stacking multiple such layers, the context scope can be gradually expanded from a central word to both sides and achieve the whole sentence at the top layer. Furthermore, the D-LSTM module is compatible with parallel computing and amenable to training via back-propagation for its vertical prorogation. Experimental results on both classification and sequence tagging datasets insist that our models achieve competitive performance compared to existing LSTMs.
更多查看译文
关键词
feed-forward
AI 理解论文
溯源树
样例
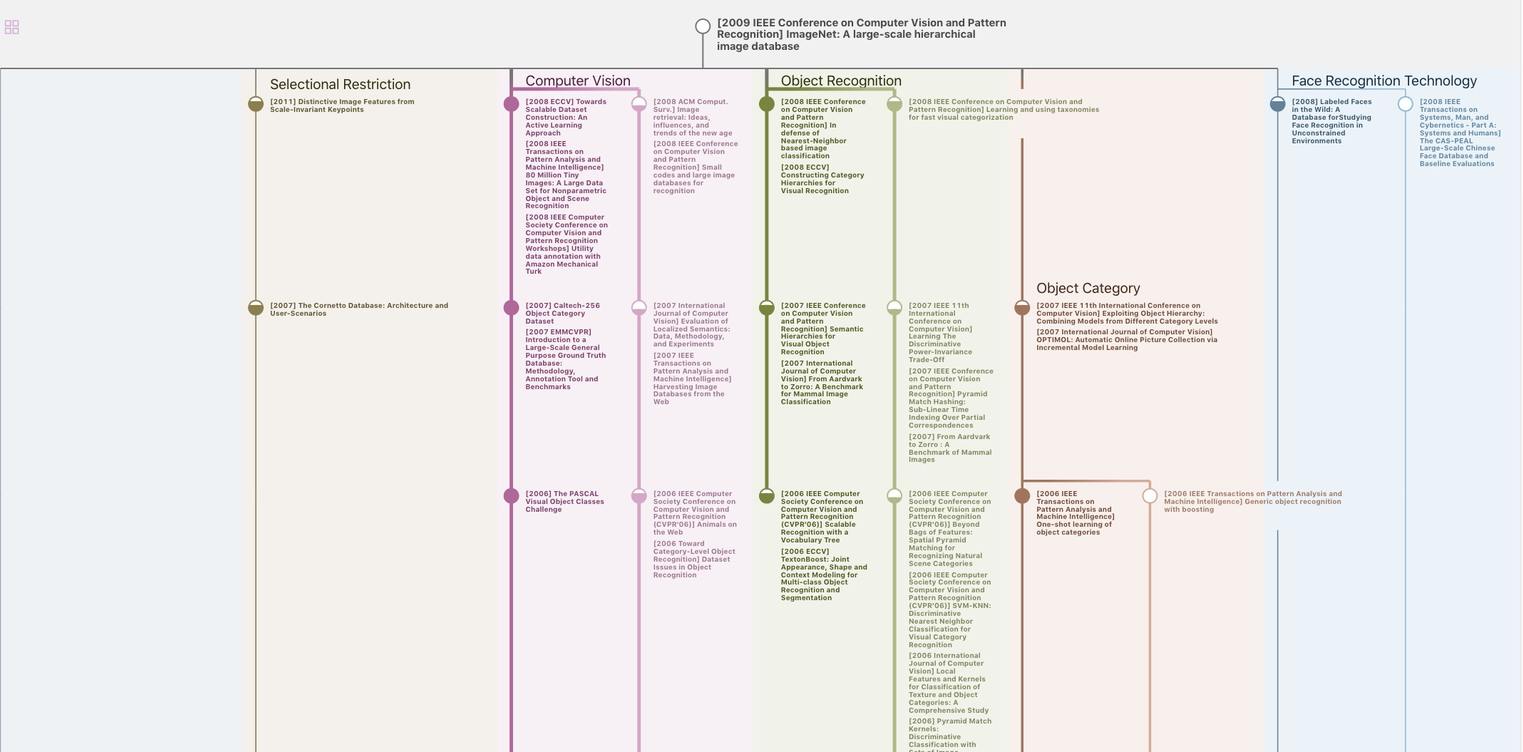
生成溯源树,研究论文发展脉络
Chat Paper
正在生成论文摘要