A Reinforcement Learning-Based Approach for Continuous Knowledge Graph Construction.
KSEM (4)(2023)
摘要
Currently, the knowledge graph construction from the text mainly relies on document-level relation extraction models. However, these models have lower accuracy due to the limitations in capturing relations across sentences and documents. To address this problem, this paper proposes a novel approach to continuously construct a knowledge graph based on a reinforcement learning framework, which leverages a question generation model, question answering model, and sentence-level relation extraction model to mine cross-document domain knowledge for effective knowledge updating. Three joint rewards are designed to optimize the question generation model, making it generate high-quality questions that facilitate the knowledge graph construction. Automatic evaluation combined with manual evaluation is conducted based on the SQuAD dataset, to assess the quality of the questions and generated knowledge graph respectively. The experiments and analyses demonstrate the effectiveness of our approach in improving the quality of the knowledge graph construction.
更多查看译文
关键词
continuous knowledge graph construction,reinforcement,learning-based
AI 理解论文
溯源树
样例
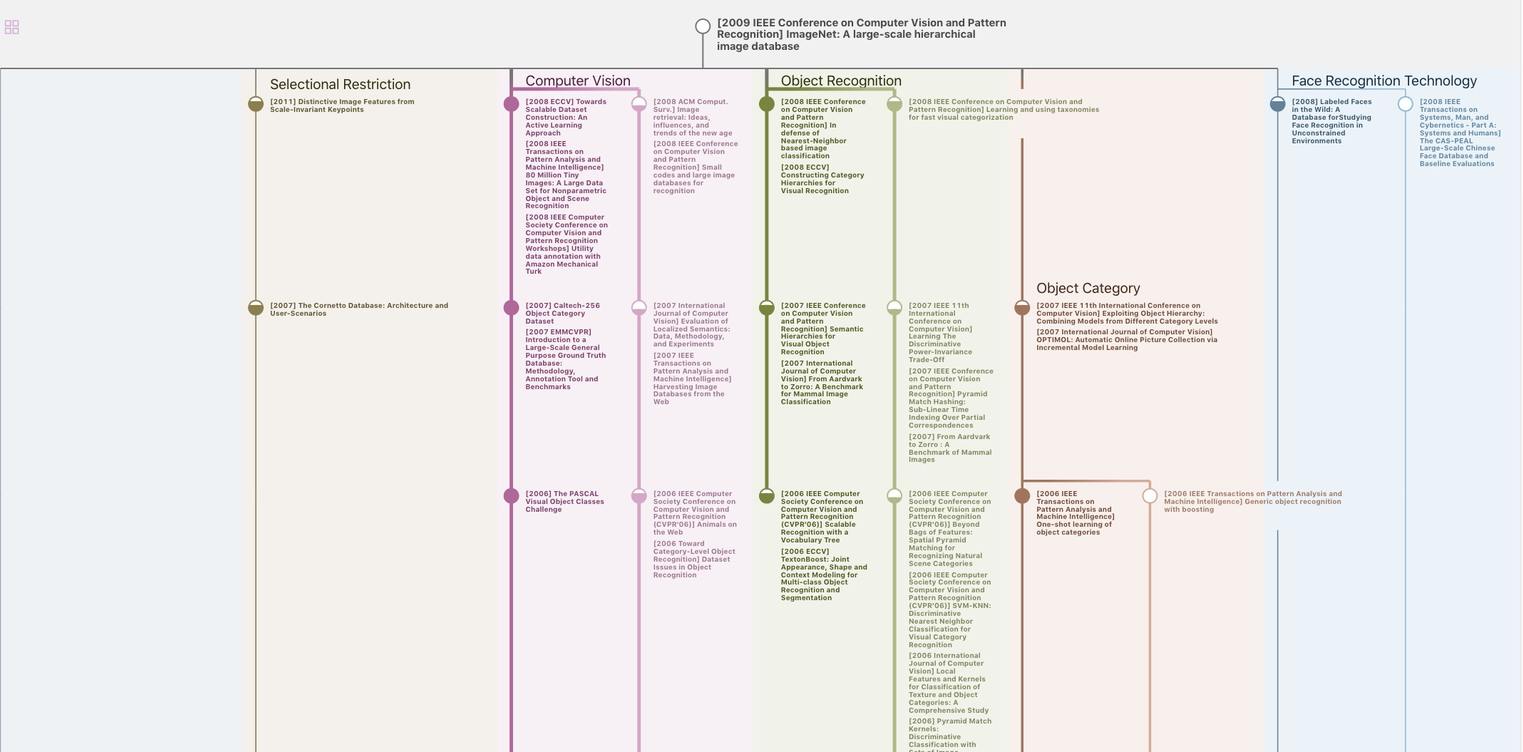
生成溯源树,研究论文发展脉络
Chat Paper
正在生成论文摘要