Multi-agent Reinforcement Learning-based Resource Allocation for Minimizing Age of Synchronization in Vehicular Networks.
ICCMS(2023)
摘要
Vehicle-to-vehicle (V2V) communication has been exploited as a promising solution to avoid accidents and improve traffic efficiency, which requires a high reliability and a low end-to end latency. The freshness of data is critical for some time-sensitive application in V2V communication networks. Due to channel time-varying, and the mobility of vehicles, how to allocate the frequency channels and control the transmit power of vehicles is critical for information transmission timely in vehicular networks. In this work, we investigate the resource allocation problem for V2V communications to minimize the data freshness, where Age of synchronization (AoS) as a new metric is adopted to measure the information freshness from the perspective of information receivers in V2V link. We formulate the AoS minimization problem by jointly optimizing channel selection and power control of V2V links in vehicular networks. To solve it, we propose a multi-agent deep Deterministic Policy Gradient (MADDPG) based resource optimization algorithm, which is with centralized training and decentralized execution characteristics based on the actor-critic framework. Simulation results illustrate that the proposed scheme is with good convergence and outperforms other existing schemes in terms of average AoS performance.
更多查看译文
AI 理解论文
溯源树
样例
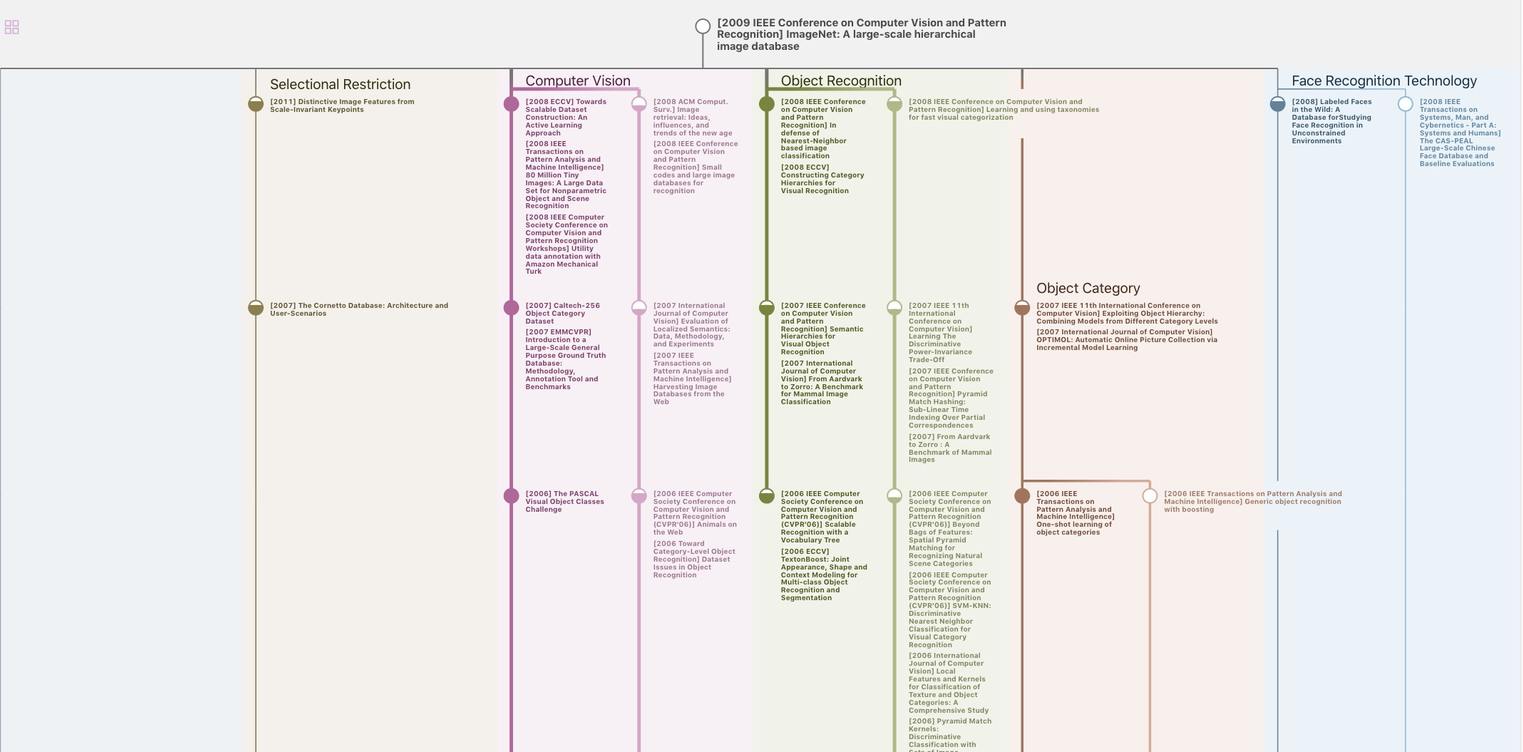
生成溯源树,研究论文发展脉络
Chat Paper
正在生成论文摘要