Combining Max-Tree and CNN for Segmentation of Cellular FIB-SEM Images.
RRPR(2022)
摘要
Max-tree (or component-tree) is a hierarchical representation which associates to a scalar image a descriptive data structure induced by the inclusion relation between the binary components obtained at successive level-sets. Various attributes related to these binary components can be computed and stored into the tree. Max-trees have been involved in many applications, enabling to perform attribute filtering in an efficient algorithmic way. Since the resulting images do not contain any new contour, these kind of filters are called connected operators . In this paper, we propose to rely on max-trees and attribute filters to enrich the input of a convolutional neural network (CNN) to improve a task of segmentation. More precisely, two approaches are considered: a first approach in which images are preprocessed using attribute filters and a second approach in which maps of attributes relying on max-trees are computed. Based on these two different approaches, the resulting maps are used as additional input in a standard CNN in a context of semantic segmentation. We propose to compare different attributes and nodes selection strategies and to experiment their usage on a practical problem: the segmentation of the mitochondria and endoplasmic-reticulum in Focused Ion Beam milling combined with Scanning Electron Microscopy (FIB-SEM) images. We provide original images, annotations, source code and a documentation to reproduce the experimentation results.
更多查看译文
关键词
segmentation,cnn,images,max-tree,fib-sem
AI 理解论文
溯源树
样例
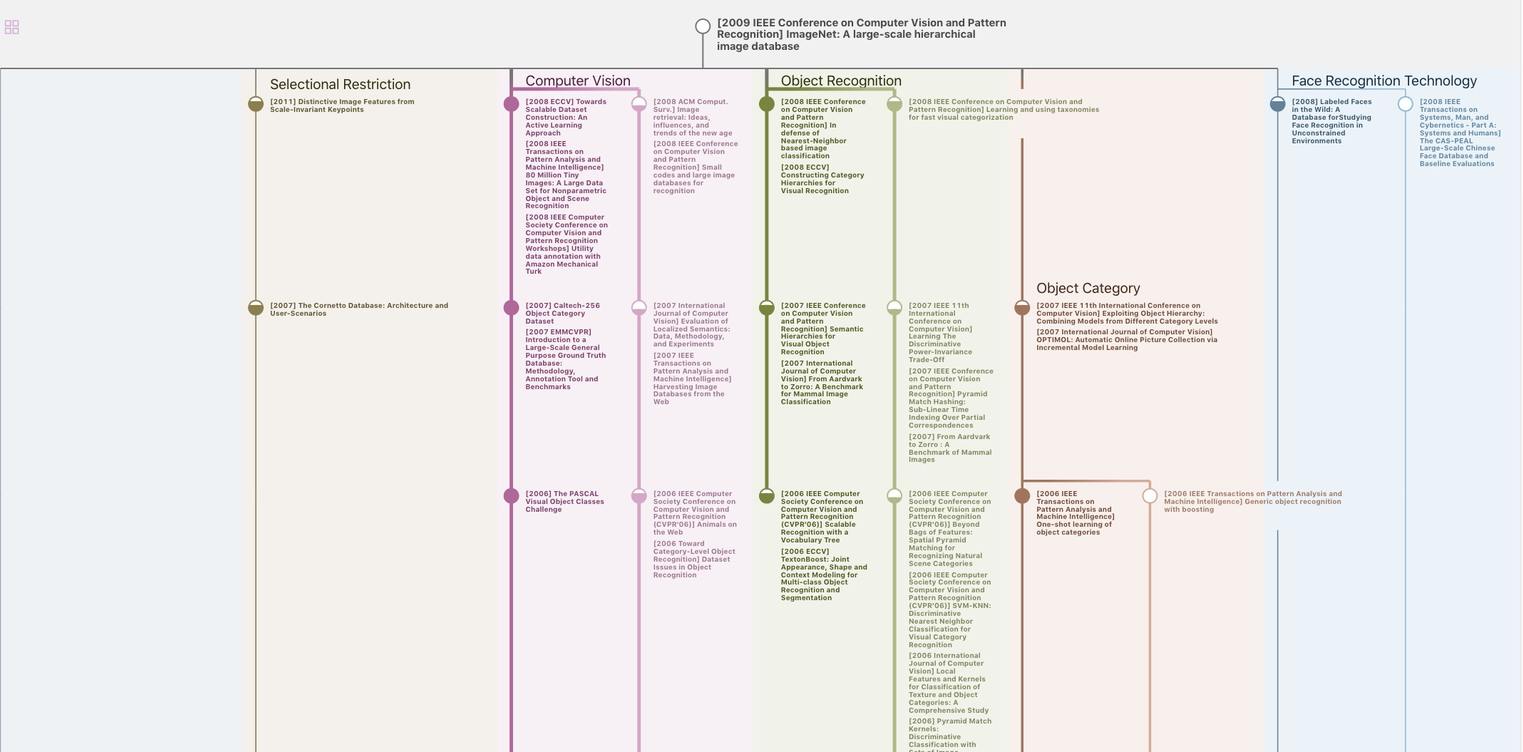
生成溯源树,研究论文发展脉络
Chat Paper
正在生成论文摘要