Machine Learning-based Millimeter Wave Beam Management for Dynamic Terminal Orientation
VTC2023-Spring(2023)
摘要
Time-varying terminal orientation is an often overlooked challenge of beam alignment in millimeter wave communications with multi-panel handset terminals. The use of narrow beams, allied with fast and hard to predict device orientation changes, cause the current measurement-based beam management procedure to rely on potentially outdated beam information, degrading its accuracy. This paper explores the capabilities of deep neural networks to improve user equipment (UE) beam selection under dynamic terminal orientation conditions. Contrary to other works, the proposed solution relies solely on reference signal received power beam measurements, without the aid of other context information. Results show that this simple solution can successfully improve beam selection accuracy for fast rotating UEs, especially in line-of-sight scenarios. No-line-of-sight environments however reduce the proposed solution's effectiveness due to low power-levels and increased channel angular spread.
更多查看译文
关键词
5G NR,beam management,multi-panel,machine learning,orientation change
AI 理解论文
溯源树
样例
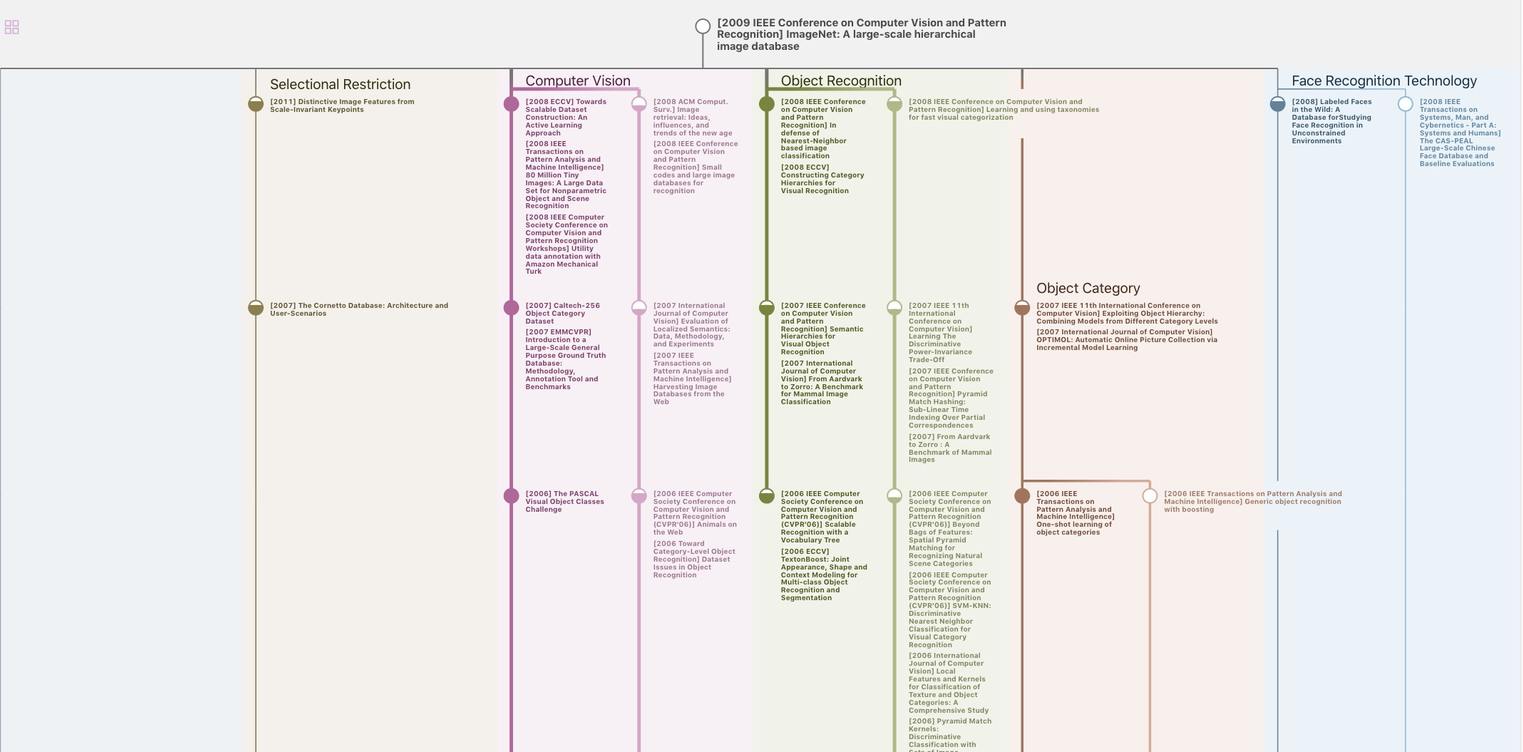
生成溯源树,研究论文发展脉络
Chat Paper
正在生成论文摘要