Temporal-frequency Features based Indoor Localization System under 5G Networks
VTC2023-Spring(2023)
摘要
This paper proposes an indoor localization system by exploring the temporal and frequency features of complex Channel State Information (CSI) under the fifth-generation (5G) cellular network. In particular, we first acquire some successive raw CSIs from multiple base stations (BSs). Then, amplitude-based sequences are obtained by employing a sliding window moving over a consecutive time step on CSI amplitudes. Moreover, the Convolutional Neural Network (CNN) and Long Short Term Memory (LSTM) are adopted to learn robust time-frequency features from the constructed CSI sequences. To emphasize the contributions of the critical elements to final location estimations, we utilize an attention mechanism to assign the local learned features with different weights. We implement the proposed scheme and verify its performance with extensive experiments in some representative indoor scenes.
更多查看译文
关键词
Indoor localization,deep learning,time-frequency features,channel state information
AI 理解论文
溯源树
样例
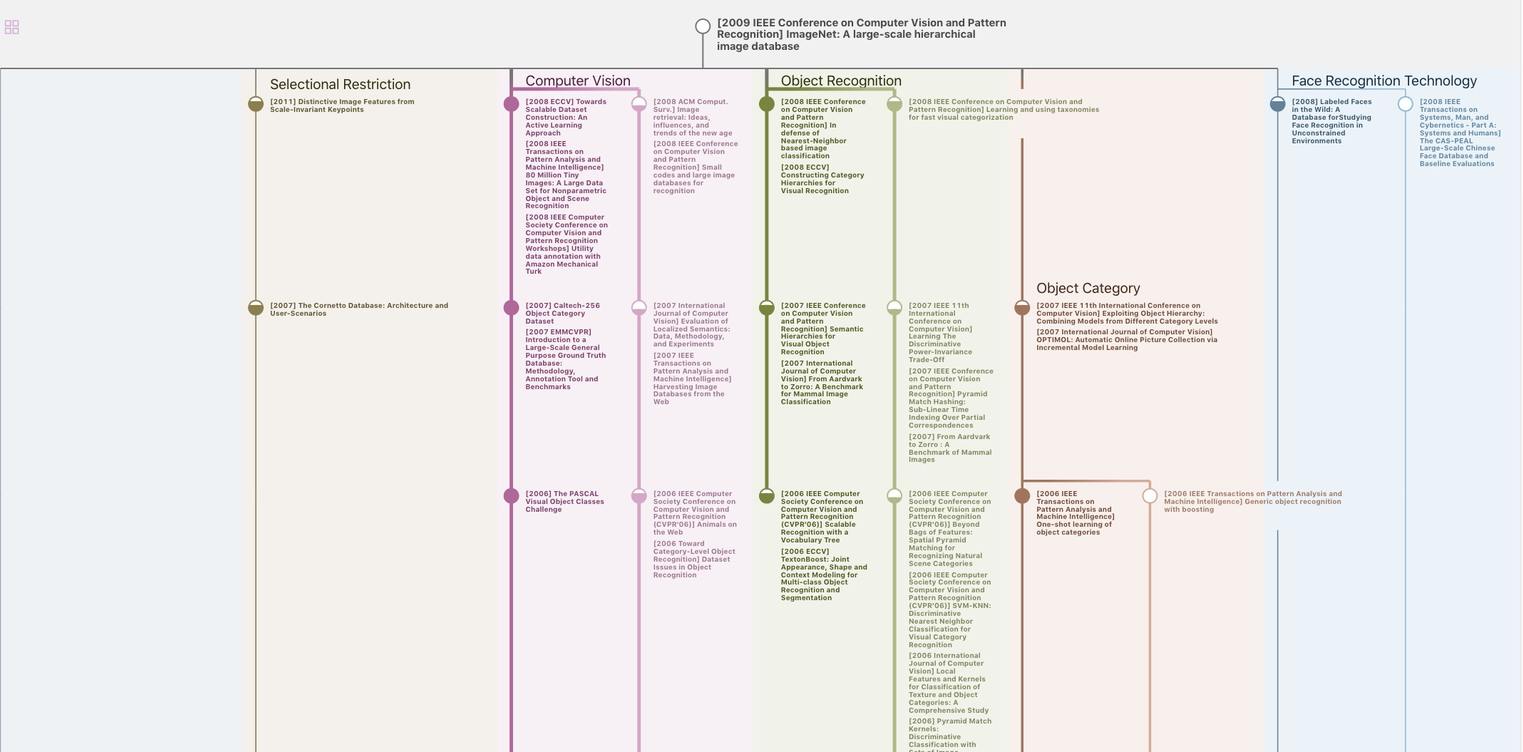
生成溯源树,研究论文发展脉络
Chat Paper
正在生成论文摘要