Meta-Critic Reinforcement Learning for IOS-Assisted Multi-User Communications in Dynamic Environments.
VTC2023-Spring(2023)
摘要
Capable of reflecting and refracting the incident signals on both sides simultaneously, the intelligent omnidirectional surface (IOS) has recently been proposed as a promising solution to enhance the capacity of wireless networks. However, the large number of IOS elements brings a heavy burden to the beamforming scheme design, especially for applications that require a fast response to varying environments. In this paper, aiming to maximize the sum rate of an IOS-aided multi-user system via IOS-enabled beamforming design that can rapidly adapt to dynamic channel states and user mobility, we develop a novel meta-critic reinforcement learning framework where a meta-critic network recognizes the environment change and automatically re-trains of the learning model. A stochastic Explore and Reload procedure is tailored to reduce the high-dimensional action space problem. Simulation results show the proposed scheme can converge to a higher sum rate more rapidly compared to the benchmark methods in dynamic settings. The robustness of our scheme against different IOS sizes is also verified.
更多查看译文
关键词
Intelligent omni-surface, Beamforming, Meta-learning, Dynamic environments
AI 理解论文
溯源树
样例
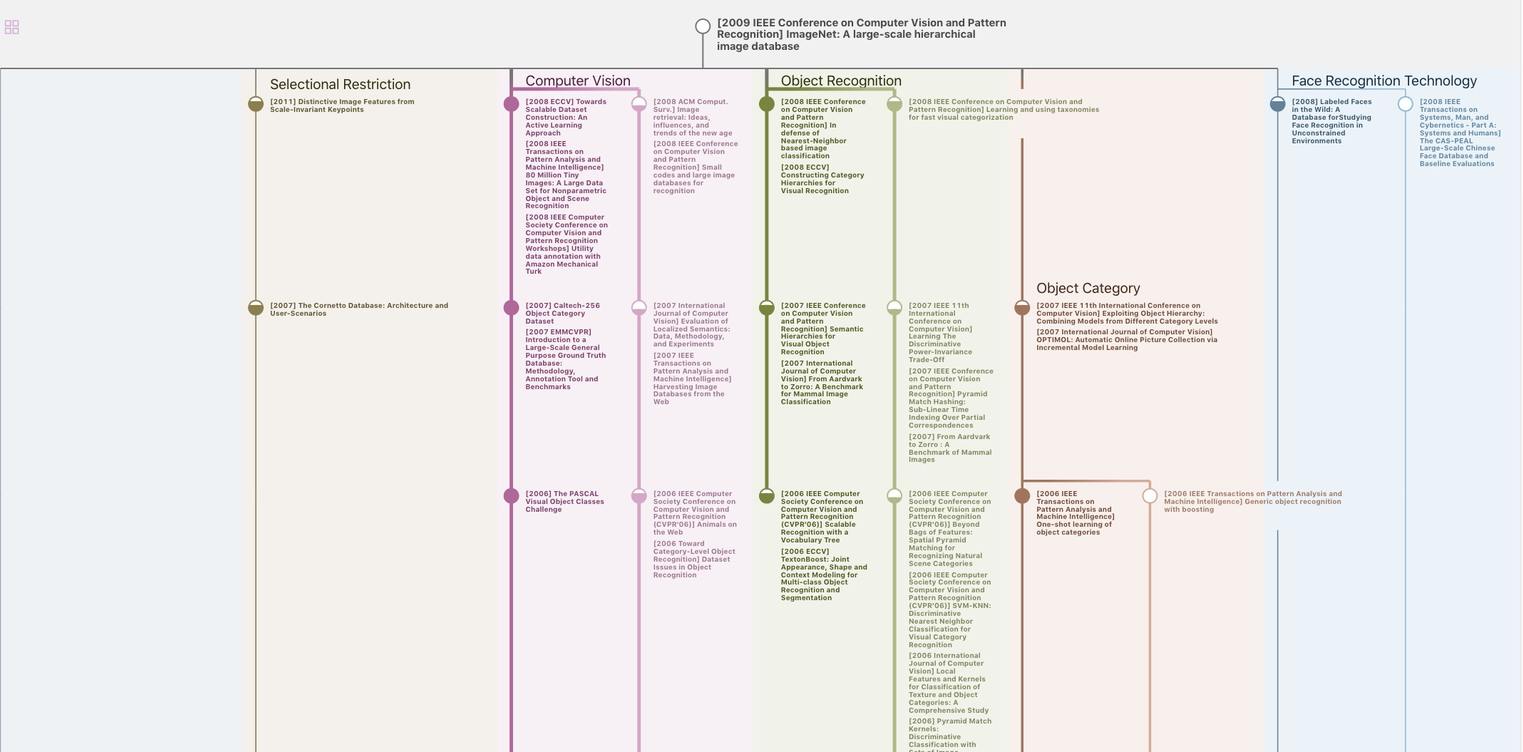
生成溯源树,研究论文发展脉络
Chat Paper
正在生成论文摘要