A Novel Statistically-Aided Learning Framework for Precise Localization of UAVs
VTC2023-Spring(2023)
摘要
The accuracy of localization using global positioning system (GPS) data plays a key role in reliable positioning and control of unmanned aerial vehicles (UAVs). This paper proposes a novel statistically-aided earning-based localization approach, called filtered neural network (FNN) for high-precision localization of UAVs. The proposed FNN framework utilizes an entropy adaptive Kalman filter to fine-tune the inputs to a recurrent neural network, which works in a loop with the filter to generate subsequent robust position estimates. The proposed framework outperforms the state-of-the-art techniques with an nRMSE of approximate to 10(-6), approximate to 97% reduced estimation delay, approximate to 73% reduced modeling time, <= 100 lag samples for FNN training, and only 4-6 overall model retraining instances per flight trajectory. The results are verified over a wide range of mean GPS noise power.
更多查看译文
关键词
Entropy adaptive Kalman filter,filtered neural network (FNN),long short-term memory,UAV localization
AI 理解论文
溯源树
样例
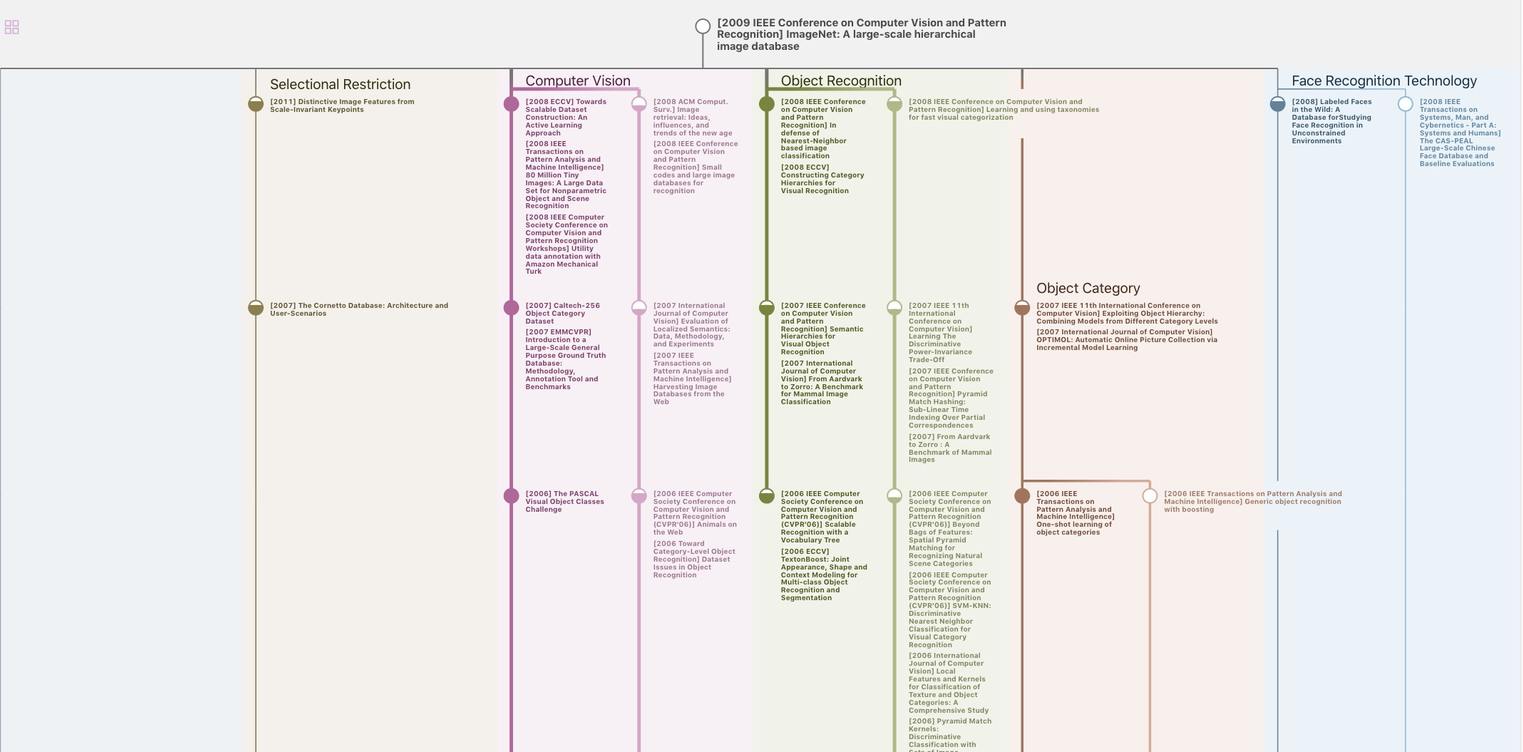
生成溯源树,研究论文发展脉络
Chat Paper
正在生成论文摘要