MsSDEdit: Deep Learning Image Enhancement for Automated Bounding Box Annotations in Automotive Monocular Camera Applications
2023 IEEE 97TH VEHICULAR TECHNOLOGY CONFERENCE, VTC2023-SPRING(2023)
摘要
Recent advances in deep learning image enhancement enable new methods to automate 2D bounding box annotations required to train object detectors based on convolutional neural networks used in automotive monocular cameras. Thus, the following paper proposes an approach to automate the generation of 2D bounding box annotated ground truth images. The implementation of the proposed approach makes use of the CARLA simulation environment and deep learning image enhancement based on stochastic differential editing. Images of a virtually modeled camera sensor are captured in the CARLA simulation environment along with ground truth data of relevant objects in the scene. A multi-step stochastic differential editing deep learning approach then transfers all generated images into the feature space of an actual camera sensor. The enhanced images and their respective ground truth data are used to train an object detection convolutional neural network. Compared to training the convolutional neural network with unenhanced simulation images only, the precision of detecting cars in actual camera images increases by 30%, and the recall improves by 20% without adding computational costs during run-time of the object detection. Hence, the proposed approach contributes to allowing further automation of training pipelines for object detection convolutional neural networks.
更多查看译文
关键词
CARLA simulation,monocular camera,deep learning,image enhancement,SDEdit
AI 理解论文
溯源树
样例
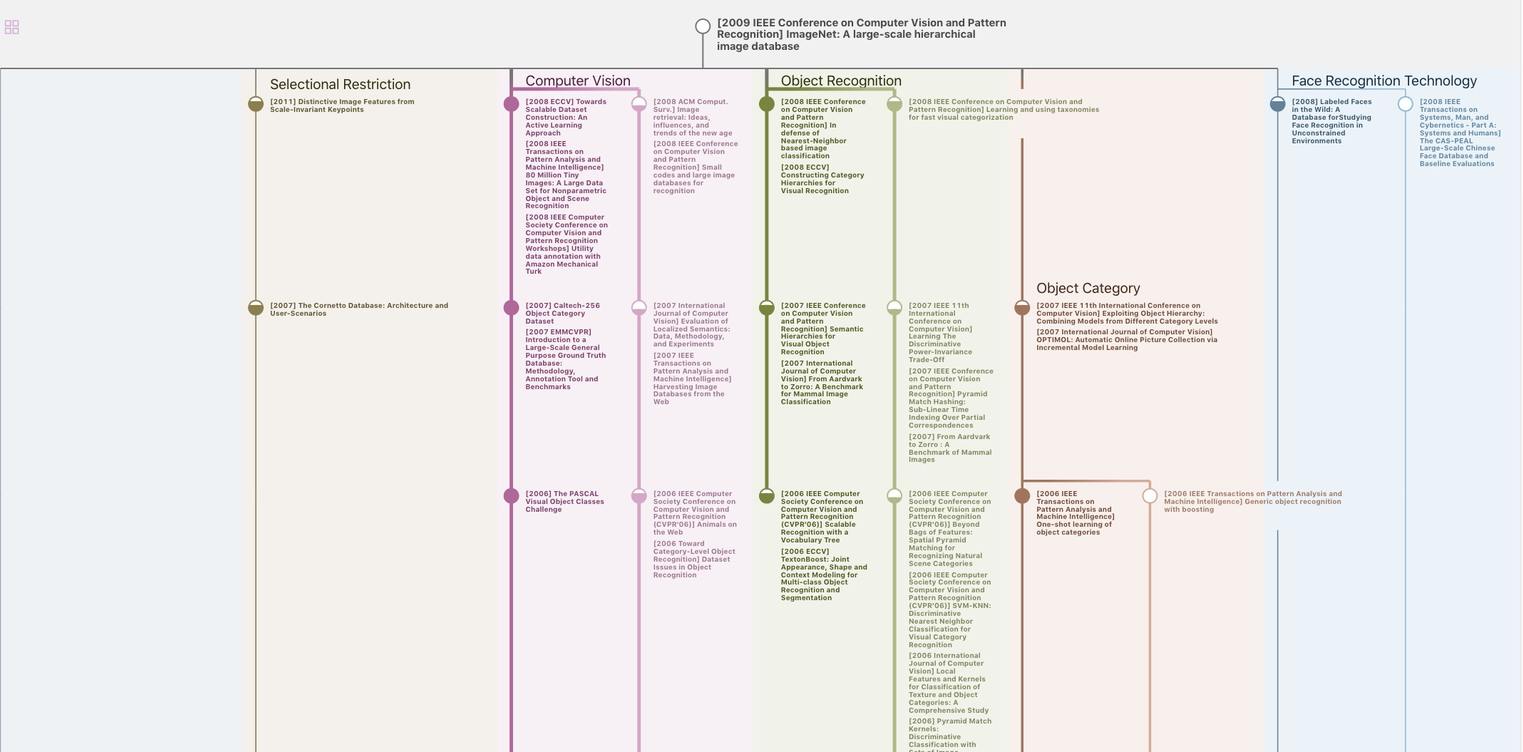
生成溯源树,研究论文发展脉络
Chat Paper
正在生成论文摘要