Efficient GCN Deployment with Spiking Property on Spatial-Temporal Neuromorphic Chips.
ICONS(2023)
摘要
Spiking Graph Convolutional Networks (SGCNs) are an emerging class of neural networks that operate on graph-structured data and leverage the advantages of both spiking neural networks and GCNs, making them ideal for graph representation learning tasks. However, deploying SGCNs on neuromorphic chips is challenging due to complex spiking dynamics, structured message computation, and limited chip resources, impeding their applications on terminal devices. In this work, we present an algorithm mapping strategy using a contemporary neuromorphic chip to efficiently deploy SGCNs and maximize chip resource utilization. Our mapping scheme leverages the spatio-temporal features of the chip and partitions computation graphs into small sub-graphs, enabling the reuse of computation resources in a temporal and spatial manner by a flexible scheme setting and corresponding scheduling. Simulation experiments show that our approach achieves favorable results on citation benchmark datasets by significantly reducing up to 79.8% memory usage and more than 60% computational requirements of SGCN deployment.
更多查看译文
AI 理解论文
溯源树
样例
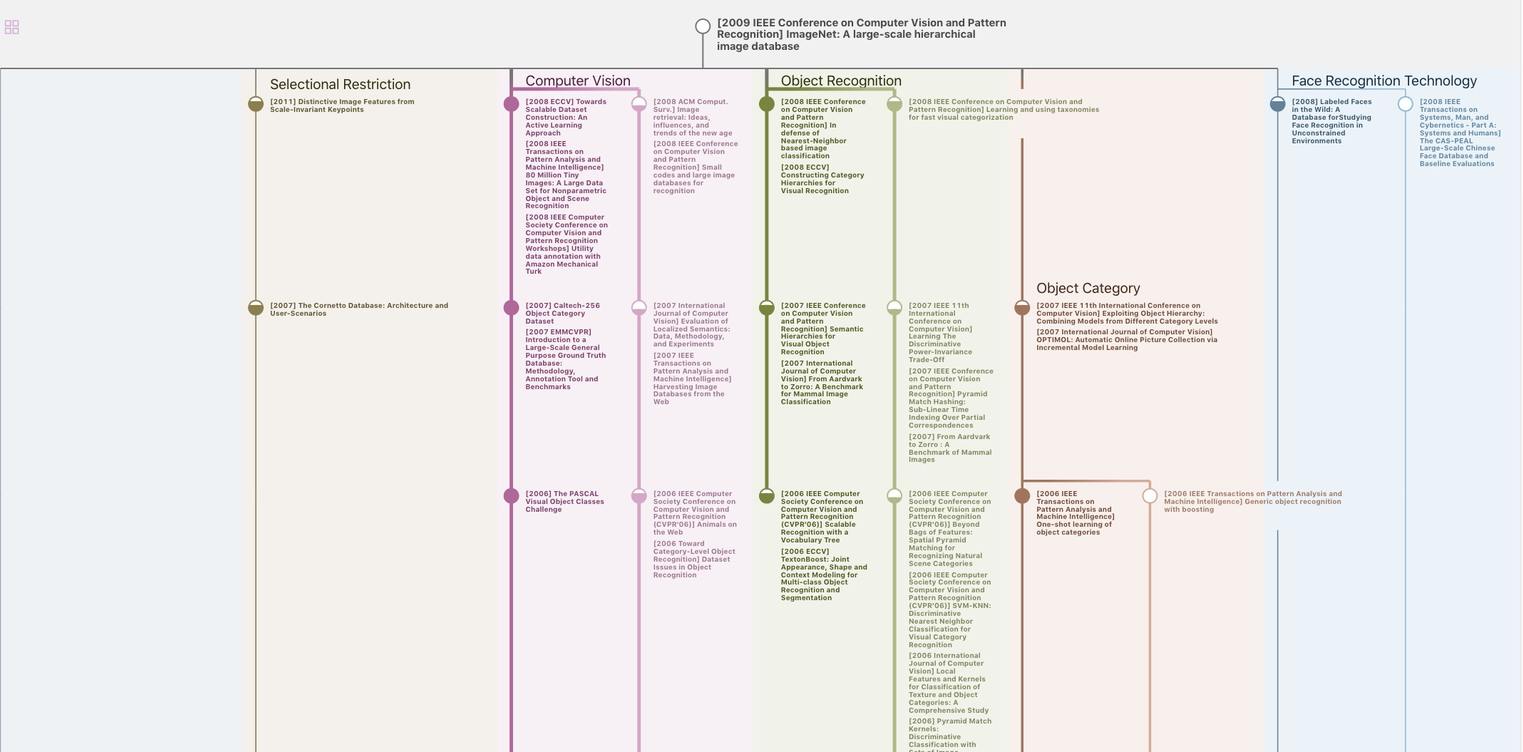
生成溯源树,研究论文发展脉络
Chat Paper
正在生成论文摘要