A Label Embedding Method via Conditional Covariance Maximization for Multi-label Classification.
DEXA (2)(2023)
摘要
Multi-label classification has revealed broad applications and outstanding importance in machine learning and data mining. In the multi-label domain, in addition to the problems including large instance size and high-dimensional features which need to be addressed urgently, the dimensionality of label space is increasing rapidly, leading to high computational overhead and curse of dimensionality. For tackling this issue, a typical technique called label space dimensionality reduction (LSDR) has been proposed, whose various corresponding methods are responsible for converting the high-dimensional label space into a low-dimensional one, including label embedding (LE) and label selection (LS), and then solve a low-dimensional classification or regression problem efficiently. In this paper, we focus on LE approaches, whose performance greatly depends on a preferable LE criterion. We investigate the conditional covariance operator, which originally is a nonlinear kernel-based dependence between two random vectors, formulated as a trace operation including label Gram matrix and feature Gram inverse matrix. We simplify this operator with linear label kernel matrix, which is then maximized under orthonormal constraints, resulting in an eigenvector problem. Therefore, our compression and recovery matrices correspond to those eigenvectors with several top-ranked eigenvalues. Our proposed novel LE method based on conditional covariance maximization is termed as ML-CCM for short. The experiments on four public benchmark data sets demonstrate that our proposed technique is statistically superior to three state-of-the-art LE methods on the basis of two performance evaluation metrics.
更多查看译文
关键词
conditional covariance maximization,classification,multi-label
AI 理解论文
溯源树
样例
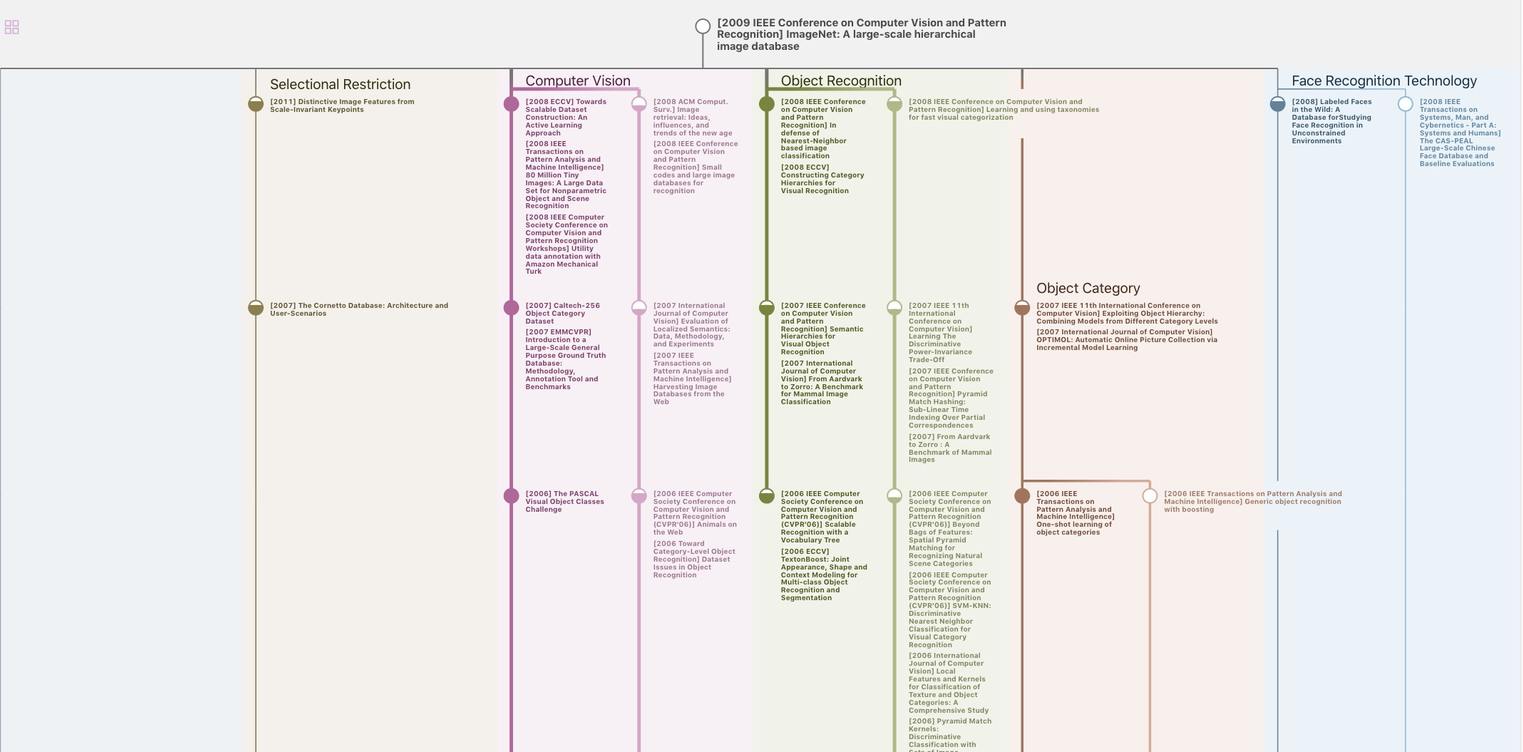
生成溯源树,研究论文发展脉络
Chat Paper
正在生成论文摘要