How Reliable Are the Deep Learning-based Anomaly Detectors? A Comprehensive Reliability Analysis of Autoencoder-based Anomaly Detectors.
ICUFN(2023)
摘要
Autoencoders have been successfully used for detecting anomalies in various intelligent systems. Generally, the autoencoder-based anomaly detection system detects a data point as anomalous if its reconstruction loss is higher than the set threshold. However, the autoencoder-based anomaly detection system lacks generalizability and reliability. Theoretically, autoencoders should produce reconstruction loss higher than the set threshold for all out-of-distribution data points. However, it has been noted that the autoencoders produce lower reconstruction loss for some data points and higher for some specific data points that are out of distribution. For example, autoencoders produce lower reconstruction loss than the set threshold for the input image with motion blur perturbation. In contrast, it produces a higher reconstruction loss for the input image with impulse noise. Motivated by the above-mentioned problem statement, this paper aims to investigate the autoencoders’ reliability in detecting various anomalies. Our experimental results found that autoencoders are highly data-specific, and the detection results are highly unreliable for various anomalies. We proposed a framework with designed reliability evaluation metrics to help assess the reliability of autoencoder-based anomaly detectors. We reported the analysis of the reliability of autoencoders in terms of their reconstruction loss against perturbed versus normal images.
更多查看译文
关键词
Anomaly Detection,Reliability,Autoencoders,Deep Learning,Safety
AI 理解论文
溯源树
样例
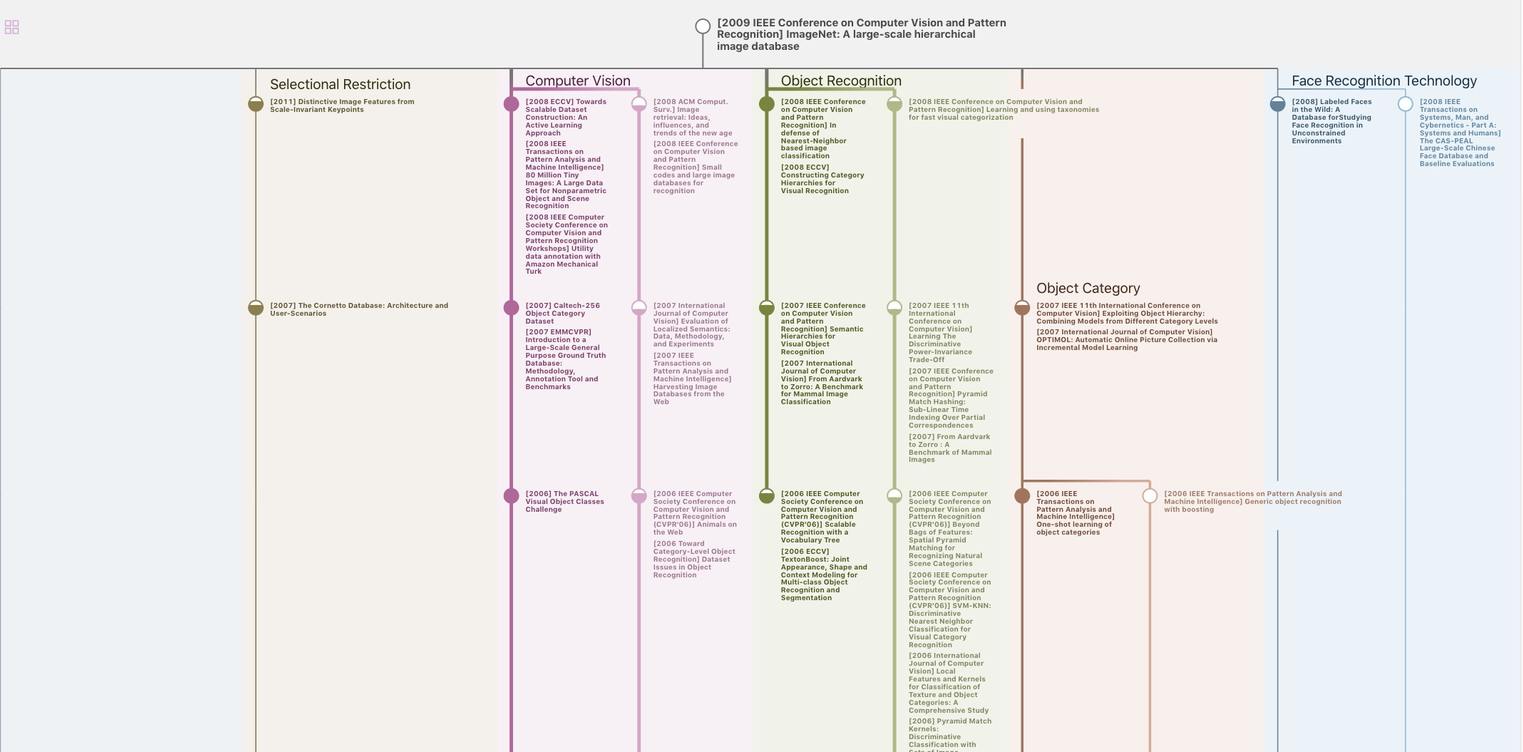
生成溯源树,研究论文发展脉络
Chat Paper
正在生成论文摘要